A Review of Deep Learning Methods for Automated Clinical Coding.
ICCAE(2023)
摘要
Clinical coding is an administrative function in hospitals, which involves the transformation of clinical notes into structured codes that can be analyzed statistically. Coding data has a number of benefits, including speeding up the administration process of insurance companies and hospitals, improving global data sharing, and facilitating statistical analysis and forecasting. Current healthcare practice involves an individual as a clinical coder interpreting information about an aspect of patient care and assigning standardised codes. Thus, the current manual coding process is labor-intensive, time-consuming, and error-prone. Computer-assisted coding has emerged in the healthcare industry in recent years. AI-based systems together with expert-led services can help reduce labor costs, facilitate the administration process, and provide more informed and efficient healthcare. Consequently, researchers are increasingly interested in the use of deep neural networks to automate the process of clinical coding. The objective of this brief literature review is to summarize and describe the characteristics of the International Classification of Diseases (ICD), list the commonly used ICD-coded datasets, discuss the state-of-the-art deep learning models for ICD coding and the effect of injecting ICD ontology into these models, and present the interpretability mechanism that have been developed and implemented for clinical coding.
更多查看译文
关键词
Automated Clinical Coding,Electronic Health Records,Explainable and Interpretable AI,Multi-label Classification
AI 理解论文
溯源树
样例
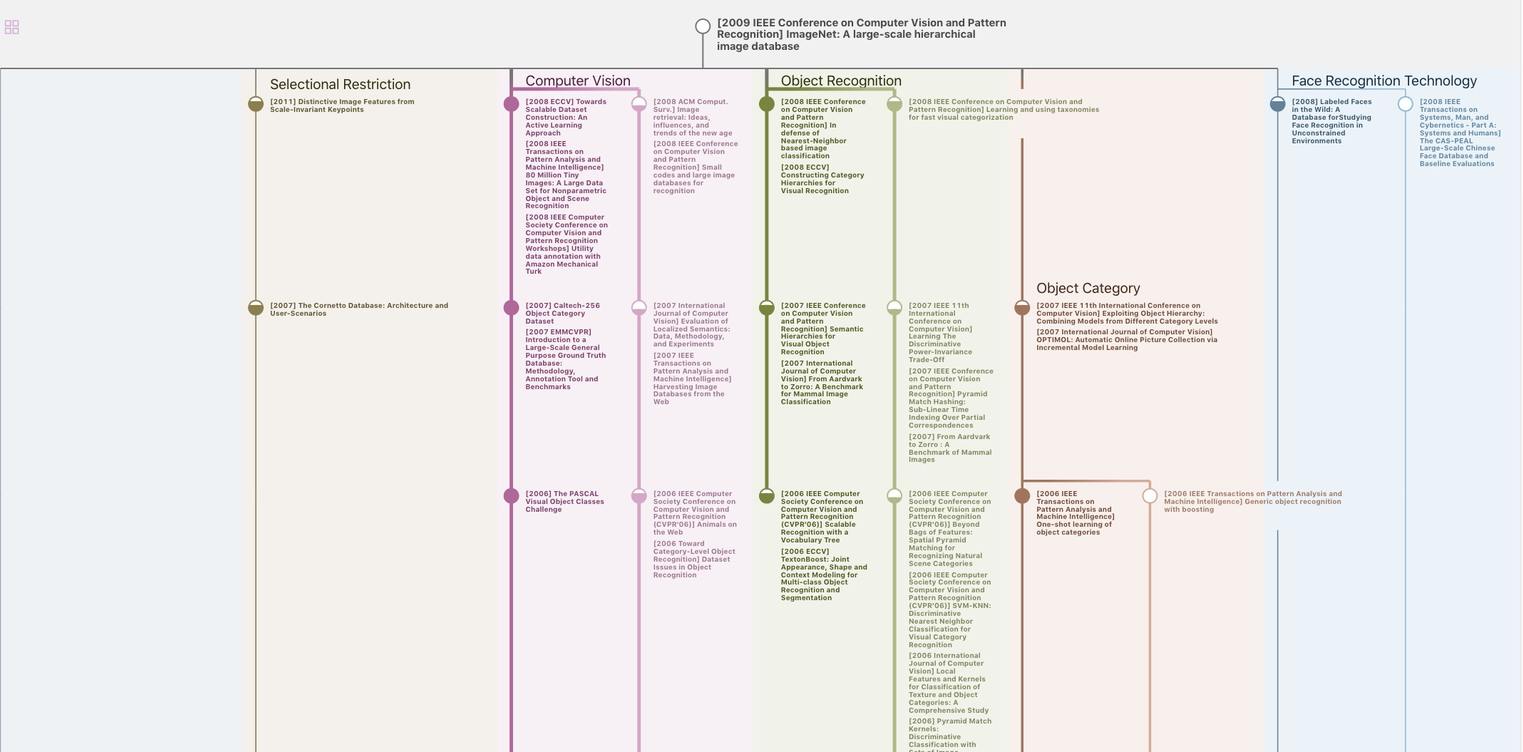
生成溯源树,研究论文发展脉络
Chat Paper
正在生成论文摘要