Stacked graph bone region U-net with bone representation for hand pose estimation and semi-supervised training.
Image Vis. Comput.(2023)
摘要
3D hand estimation from 2D joint information is an essential task in human-machine interaction, which has achieved great progress as an application of deep learning. However, regression-based methods do not perform well because the structural information is not effectively exploited, and the joint coordinates are variable. To address these issues, the hand pose is represented with bone vectors instead of joint coordinates in this study, which are stabler to learn and allow for easier encoding of the hand geometric structure and joint dependency. A novel graph bone region U-Net is specifically designed for bone representation to learn multiscale structural features, where the proposed novel elements (graph convolution, pooling and unpooling) incorporate hand structural knowledge. Under the introduced “finger-to-hand” framework, the network gradually decreases the scale from bone to finger to hand for learning more meaningful multiscale features. Moreover, the unit network is stacked repeatedly to extract multilevel features. Based on the above network, a simple but effective semi-supervised approach is introduced to address the lack of 3D hand pose labels. Many experiments are conducted to evaluate the proposed approach on two challenging datasets. The experimental results show that the proposed supervised approach outperforms the state-of-the-art methods, and the proposed semi-supervised approach can still achieve favorable performance when the labeled data are scarce.
更多查看译文
关键词
bone representation,hand,graph,u-net,semi-supervised
AI 理解论文
溯源树
样例
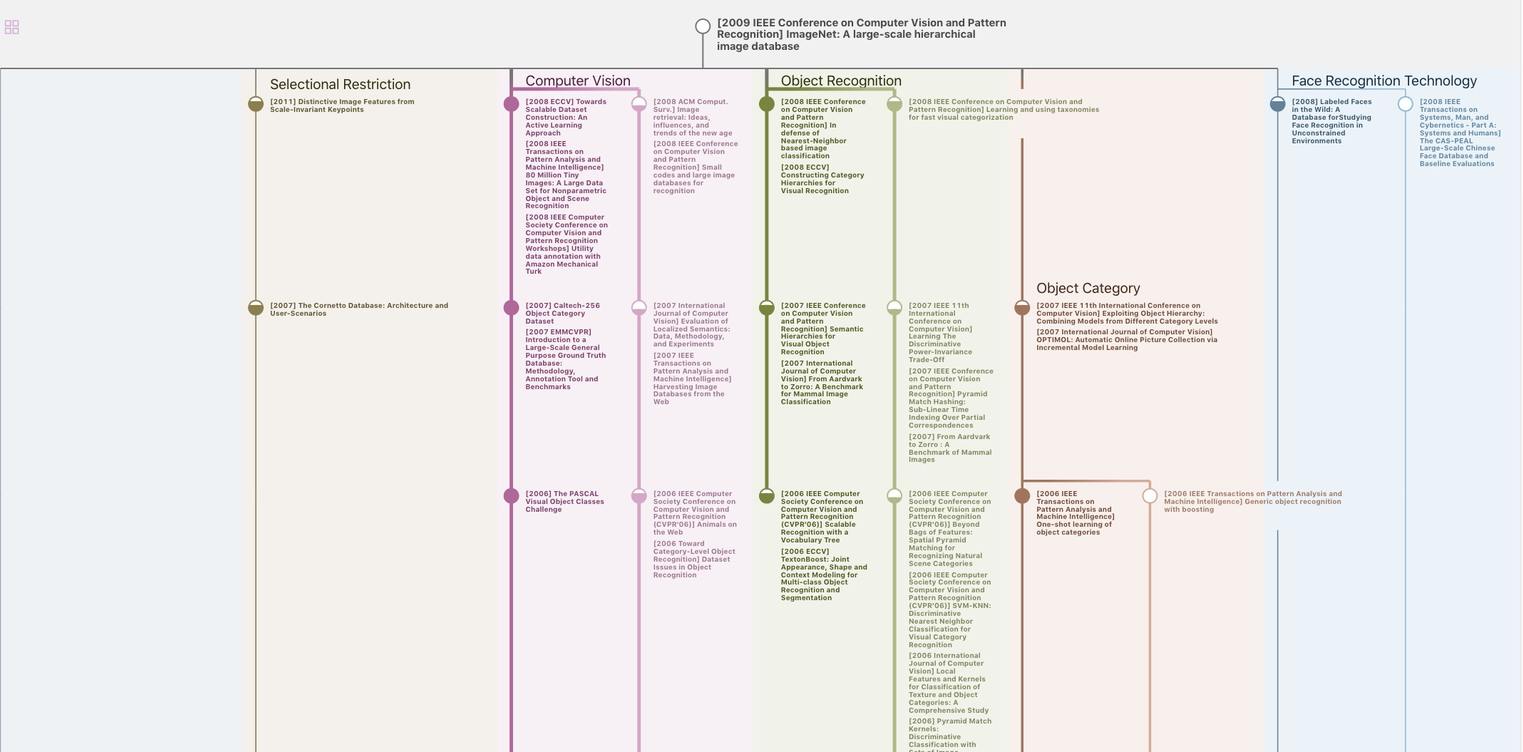
生成溯源树,研究论文发展脉络
Chat Paper
正在生成论文摘要