Accuracy and diversity-aware multi-objective approach for random forest construction.
Expert Syst. Appl.(2023)
摘要
Random Forest is an ensemble classification approach. It aims to design a discrete finite group of decision trees constructed based on bootstrap samples and random attribute selection. Random Forests have strong generalization capacities due to the variance in the training and attribute couple subsets used for constructing different decision trees in the forest. However, to construct a robust and effective random forest, two main issues need to be taken into account namely: (1) increasing the accuracy and diversity of decision trees; (2) decreasing the number of decision trees. In this paper, a genetic algorithm-based approach to tackle the aforementioned challenges related to random forest construction is proposed. Three objectives are taken into consideration. First, strengthening the classification accuracy of individual decision trees as well as that of the forest. Second, making use of diversity measures among the decision trees to improve the generalization of the constructed model. Third, minimizing the number of trees in the forest and finding an optimal subset of the random forest. An experimental evaluation on several datasets from the UCI Machine Learning Repository is conducted. The obtained results show that the proposed approach outperforms state-of-the-art classical as well as evolutionary random forest construction methods. Finally, the proposed approach is used to build a reliable random forest model for detecting Botnet traffic in Internet of Things environment.
更多查看译文
关键词
Random forest,Decision tree,Classification,Genetic algorithm,Diversity,Internet of Things
AI 理解论文
溯源树
样例
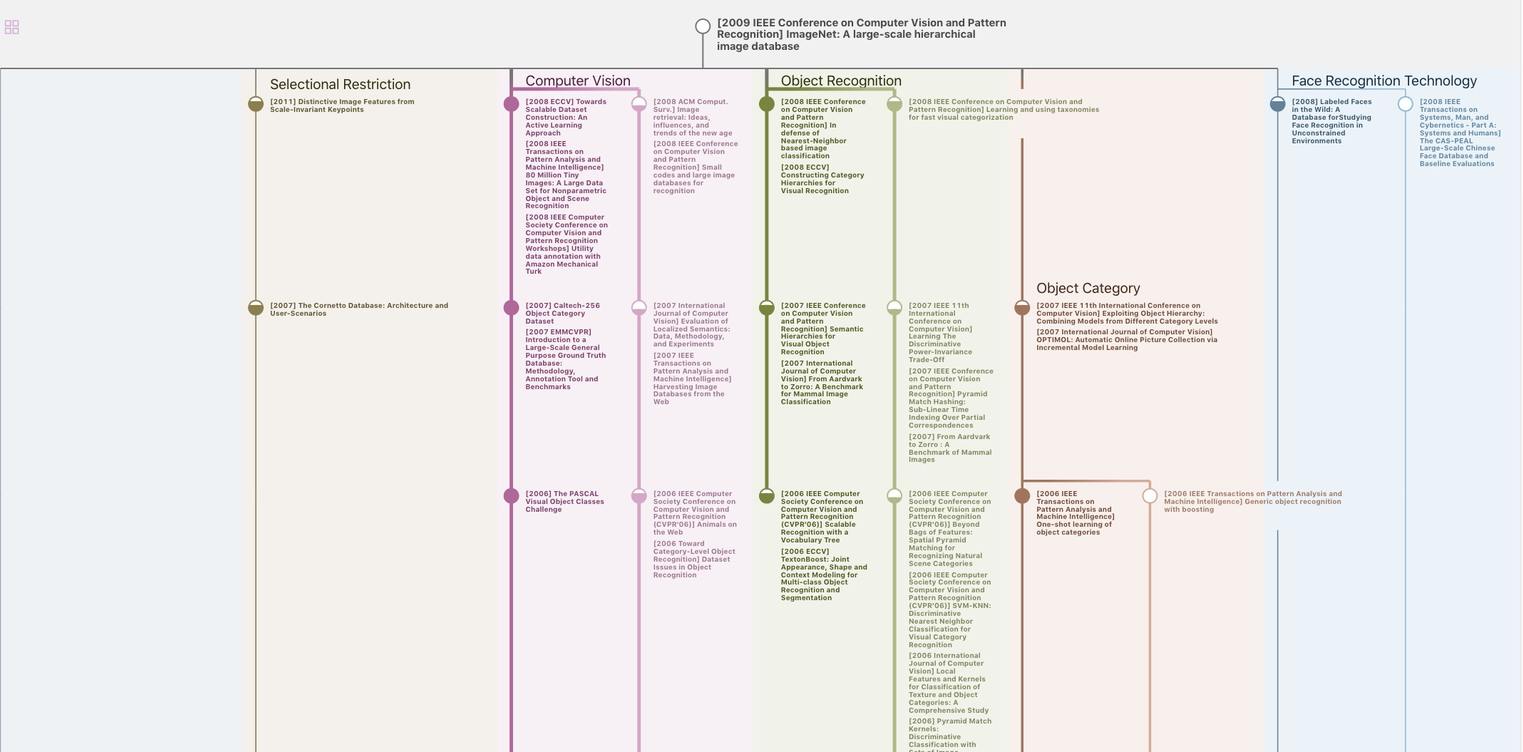
生成溯源树,研究论文发展脉络
Chat Paper
正在生成论文摘要