Improving numerical methods for the steel yield strain calculation in reinforced concrete members with Machine Learning algorithms.
Expert Syst. Appl.(2023)
摘要
In the context of reinforced concrete members subjected to shear, the steel behaviour, assumed as embedded in the concrete, has been modelled through different strategies. One of them, the so-called Refined Compression Field Theory (RCFT), is based on the concept of the concrete tension stiffening area, and shows a better fitting with the experimental results than other shear theories. However, for certain standard design conditions, the RCFT non-linear formulation does not throw a real physical solution for the steel yield strain, what hinders its numerical calculation. In fact, previous works have defined a solvability region for such strain. This non-linear equation is usually solved by iterative methods. However, the convergence of these methods depends on the root location. Moreover, their robustness may diminish in the boundary of the solvability region. This work validates Machine Learning (ML) approaches as alternative tools for the iterative prediction of the steel yield strain. To this aim, the calculation of the steel yield strain is performed in two stages, first locating the root to calculate using ML-based classification techniques, and second calculating such root with an accurate adjustment as a function of its location, using ML-based regression algorithms. As result, the efficiency of the proposed method no longer depends on the steel strain location. Finally, despite the high influence of the concrete bond parameter in the yield strain field, the ML prediction error is homogeneous over all the problem physical domain.
更多查看译文
关键词
Reinforced concrete, Steel yield strain calculation, Iterative numerical methods, Machine learning
AI 理解论文
溯源树
样例
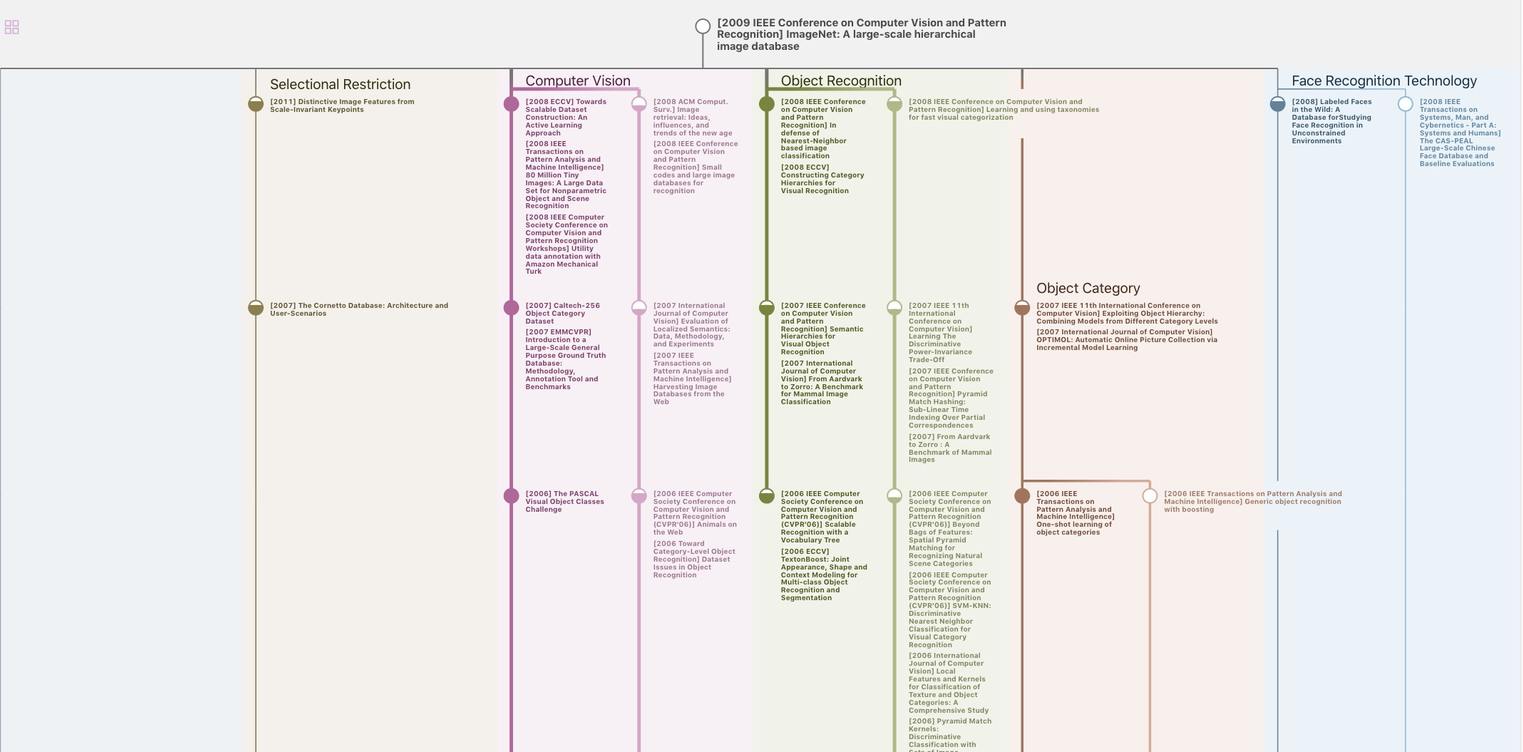
生成溯源树,研究论文发展脉络
Chat Paper
正在生成论文摘要