Denoising Diffusion Probabilistic Feature-Based Network for Cloud Removal in Sentinel-2 Imagery
Remote. Sens.(2023)
摘要
Cloud contamination is a common issue that severely reduces the quality of optical satellite images in remote sensing fields. With the rapid development of deep learning technology, cloud contamination is expected to be addressed. In this paper, we propose Denoising Diffusion Probabilistic Model-Cloud Removal (DDPM-CR), a novel cloud removal network that can effectively remove both thin and thick clouds in optical image scenes. Our network leverages the denoising diffusion probabilistic model (DDPM) architecture to integrate both clouded optical and auxiliary SAR images as input to extract DDPM features, providing significant information for missing information retrieval. Additionally, we propose a cloud removal head adopting the DDPM features with an attention mechanism at multiple scales to remove clouds. To achieve better network performance, we propose a cloud-oriented loss that considers both high- and low-frequency image information as well as cloud regions in the training procedure. Our ablation and comparative experiments demonstrate that the DDPM-CR network outperforms other methods under various cloud conditions, achieving better visual effects and accuracy metrics (MAE = 0.0229, RMSE = 0.0268, PSNR = 31.7712, and SSIM = 0.9033). These results suggest that the DDPM-CR network is a promising solution for retrieving missing information in either thin or thick cloud-covered regions, especially when using auxiliary information such as SAR data.
更多查看译文
关键词
cloud removal,SAR-optical images,generative models,deep learning
AI 理解论文
溯源树
样例
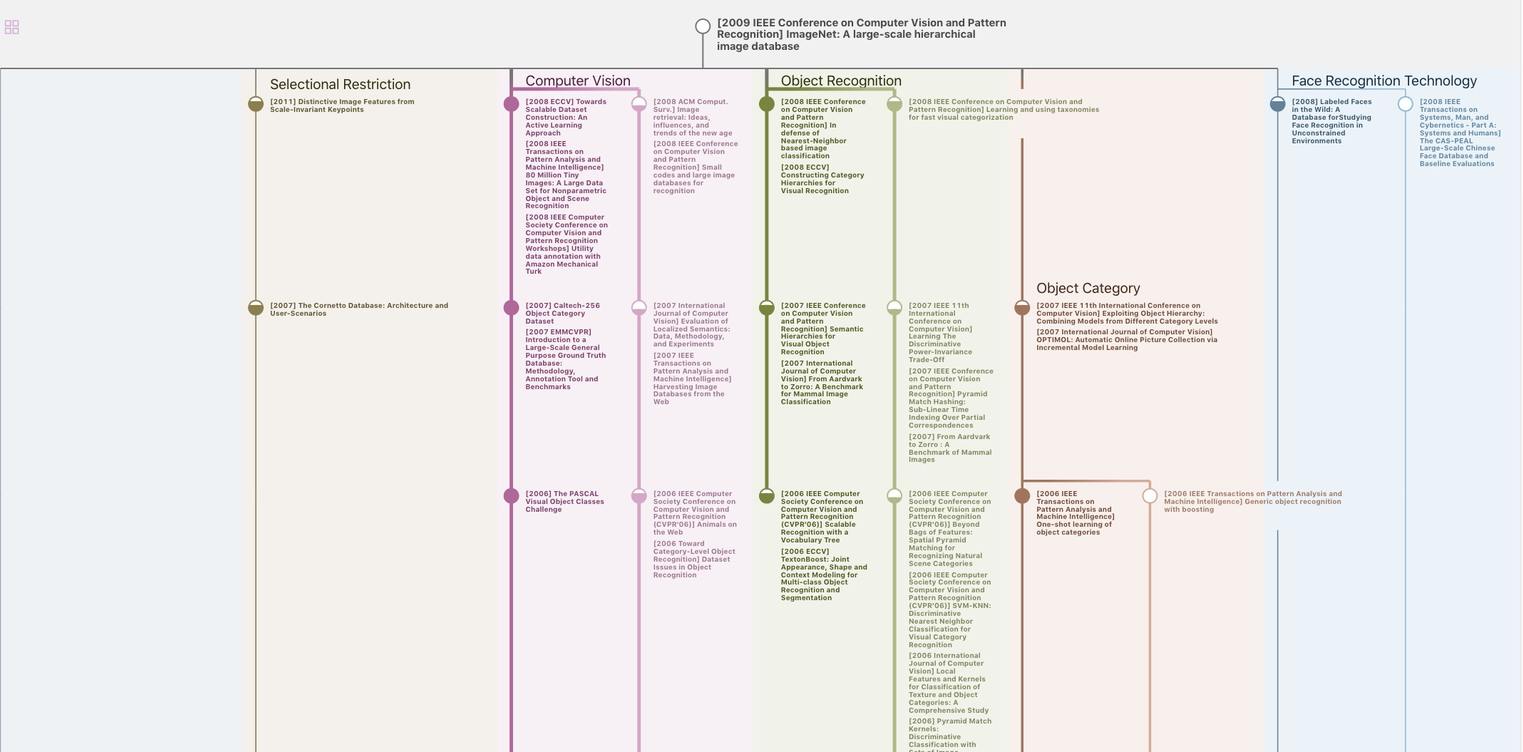
生成溯源树,研究论文发展脉络
Chat Paper
正在生成论文摘要