Region contrastive camera localization
PATTERN RECOGNITION LETTERS(2023)
摘要
Visual camera localization is a well-studied computer vision problem and has many applications. Recently, deep convolutional neural networks have begun to be utilized to solve six-degree-of-freedom (6DoF) camera pose estimation via scene coordinate regression from a single RGB image and they outperform the traditional methods. However, recent works do not consider scene variations such as viewpoint, light, scale, etc due to the camera motion. In this work, we propose a region contrastive representation learning approach to alleviate these problems. The proposed approach maps image features from different camera views of the same 3D region to nearby points in the learned feature space. In contrast, it pushes visual features of other regions to distant points. Our method improves the existing camera localization methods and achieves state-of-the-art results on indoor 7-Scenes and outdoor Cambridge Landmarks datasets. Experimental results show that the proposed approach reduces the pose and angle errors and increases the average accuracy from 84.8% to 85.62% on the state-of-the-art baseline model. In addition, we perform an ablation study on a baseline network with different settings to demonstrate the efficiency of the proposed region contrastive camera localization method. (c) 2023 Elsevier B.V. All rights reserved.
更多查看译文
关键词
Camera localization,Contrastive learning,Pose estimation
AI 理解论文
溯源树
样例
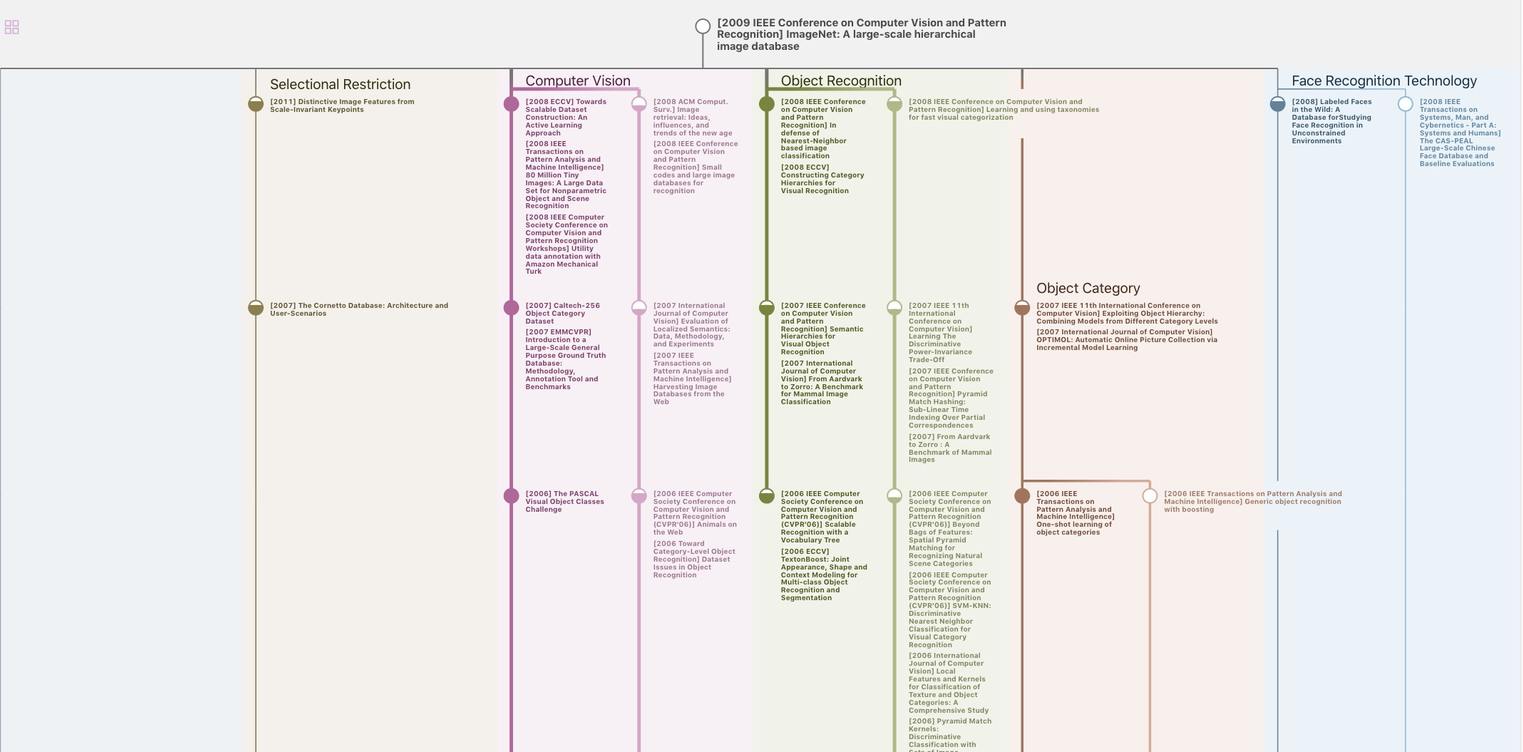
生成溯源树,研究论文发展脉络
Chat Paper
正在生成论文摘要