PESTnet - Pre-IFFT PAPR Estimation using Neural Networks for Improved OFDM Systems.
WCNC(2023)
摘要
For the next generation of wireless technologies, Orthogonal Frequency Division Multiplexing (OFDM) remains a key signaling technique. Peak-to-Average Power Ratio (PAPR) reduction must be included with OFDM to reduce the detrimental high PAPR exhibited by OFDM. The cost of PAPR reduction techniques stems from adding multiple IFFT iterations, which are computationally expensive and increase latency. We propose a novel PAPR Estimation Technique called PESTNet which reduces the necessary IFFT operations for PAPR reduction techniques by using deep learning to estimate the PAPR before the IFFT is applied. This paper gives a brief background on PAPR in OFDM systems and describes the PESTNet algorithm and the training methodologies. A case study of the estimation model is provided where results demonstrate PESTNet is able to give an accurate estimate of PAPR and can compute large batches of resource grids up to 10 times faster than IFFT based techniques.
更多查看译文
关键词
OFDM,5G,LTE,PAPR,CNN
AI 理解论文
溯源树
样例
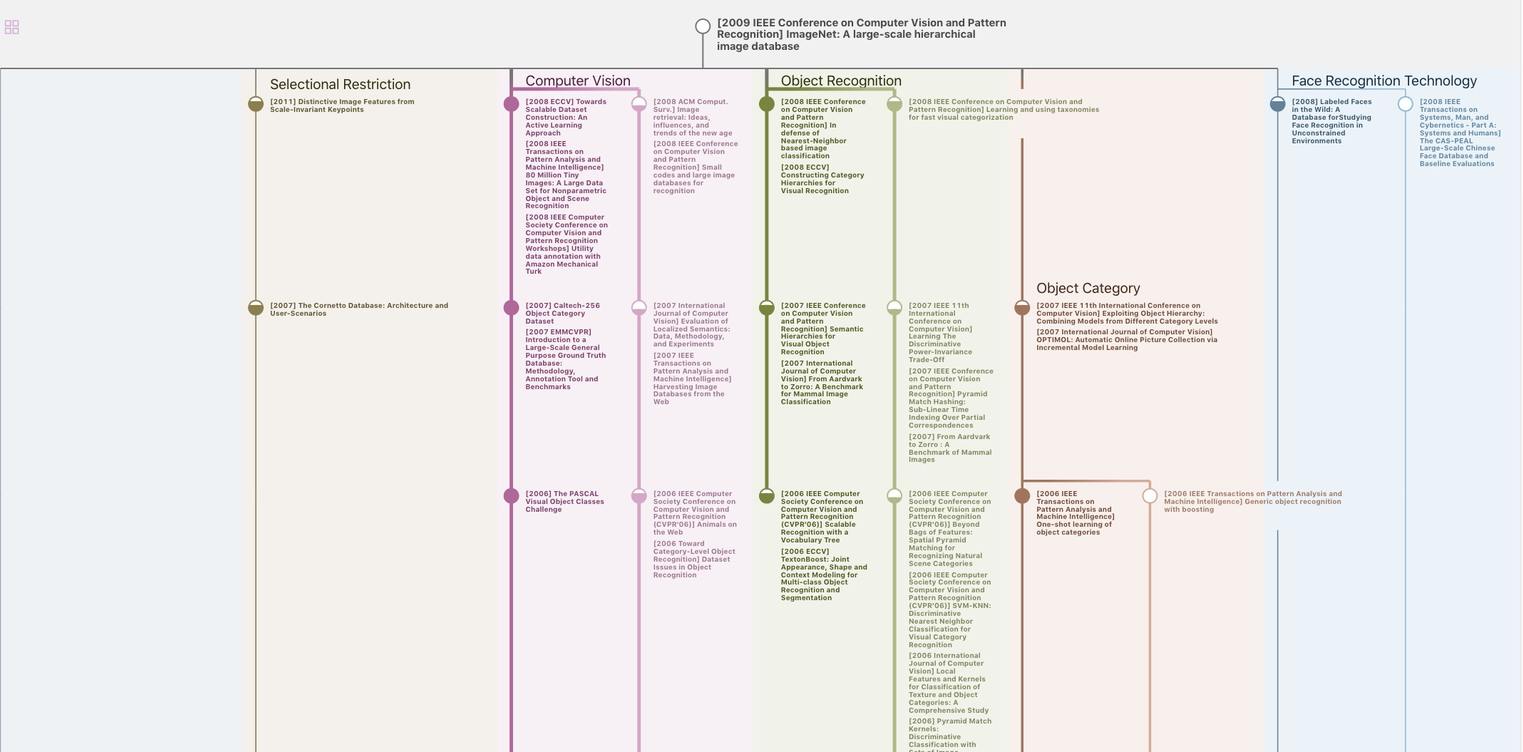
生成溯源树,研究论文发展脉络
Chat Paper
正在生成论文摘要