Multi-scale modeling temporal hierarchical attention for sequential recommendation.
Inf. Sci.(2023)
摘要
Multi-scale modeling of items interacting in a sequence of users' historical behaviors in a sequential recommendation task is crucial. In real scenarios, the user's choice of items depends not only on static preferences (long-term interests) but also on recent dynamic preferences (short-term interests). In recent years, micro-video sharing platforms have been very favorable, and correspondingly, more efficient recommendation method will be needed to support users in finding their interested micro-videos (items). Compared to traditional online videos (such as YouTube), micro-videos are created by grassroots users, shot by smartphones, are short (typically tens of seconds), and have fewer tags or descriptive text, which makes recommending it a challenging task. In this work, we explore how to model users' historical behavior sequences at multiple scales to predict their click-through rates on micro-videos and then determine whether to recommend them to users. We present a novel Multi-scale Modeling Temporal Hierarchical Attention (MMTHA) method for modeling users' behavior sequences inspired by recent deep network-based approaches. Specifically, firstly, we capture users' short-term dynamic interests using temporal windows; secondly, we utilize a category-level attention mechanism to describe the coarse-grained interests of users and an item-level attention mechanism to capture the fine-grained interests of users; thirdly, we employ a forward multi-headed self-attention mechanism to identify and integrate long-term correlations between previously segmented temporal windows. We conducted extensive experiments on two publicly available datasets to verify their effectiveness. The experimental results show that our proposed MMTHA model achieves state-of-the-art performance in all tests.
更多查看译文
关键词
User behavior sequences,Multi-scale modeling,Sequential recommendation,Temporal hierarchical attention,Micro-video
AI 理解论文
溯源树
样例
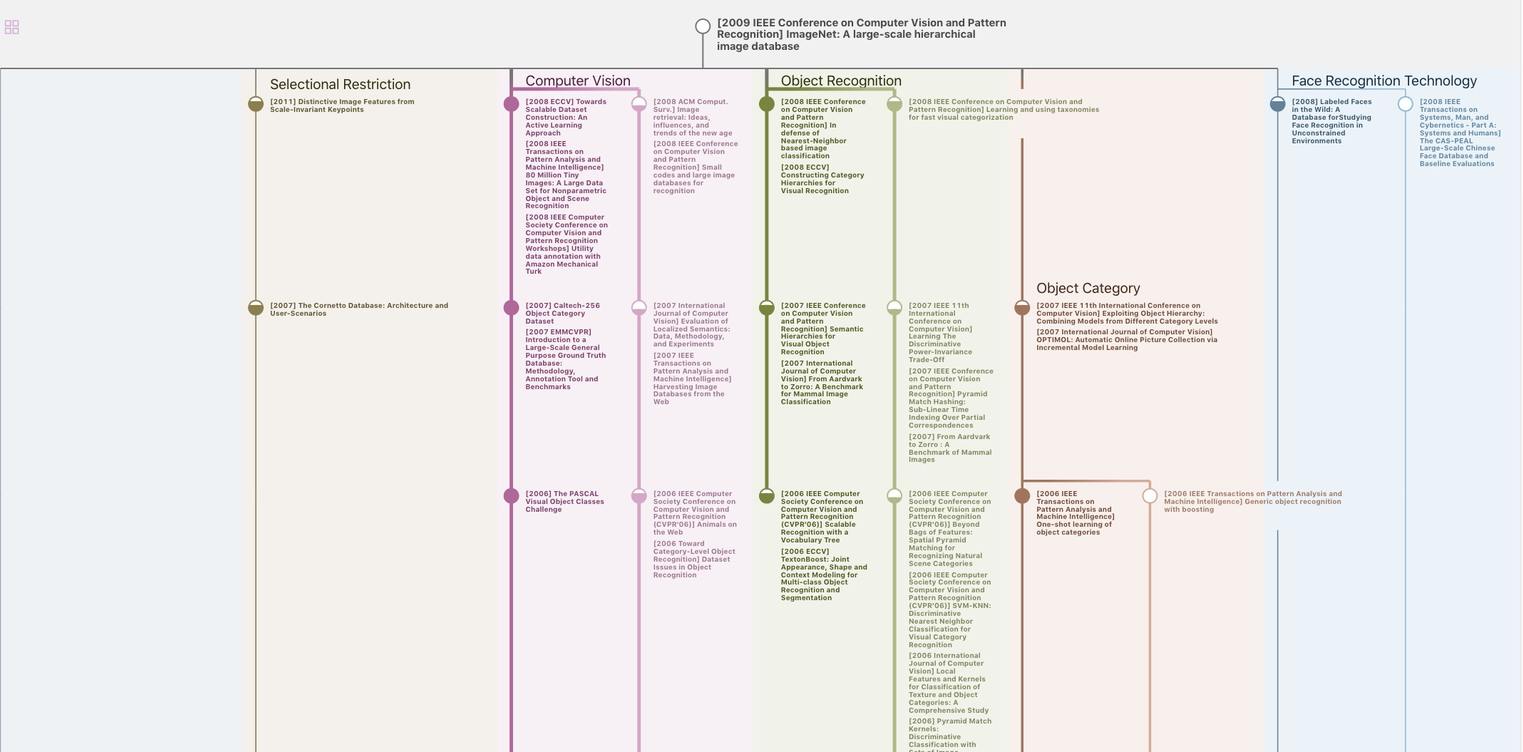
生成溯源树,研究论文发展脉络
Chat Paper
正在生成论文摘要