SRFA-GRL: Predicting group influence in social networks with graph representation learning.
Inf. Sci.(2023)
摘要
Group influence evaluation is the fundamental research in social network analysis, whose main task is evaluating the influence of the group consisting of arbitrary nodes in the social network. Many methods have been proposed to measure group influence, such as centrality-based, Monte Carlo, and path-based methods. Graph Representation Learning (GRL) has profound success in social network node-level, edge-level, and graph-level tasks. GRL integrates nodes, edges, and other information in the network structure to calculate the nodes' embeddings jointly and provide embedding features with richer information than traditional methods. Group influence is related to many factors; existing methods only focus on a single aspect but ignore the various properties. This paper proposes a Subgraph Reconstruction Feature Aggregation Graph Representation Learning based (SRFA-GRL) framework to evaluate the group influence. In the SRFA-GRL framework, a subgraph reconstruction method is proposed to capture the node distribution of the group, and a vector similarity method is proposed to compute the relative distance between the maximal connected subgraph and group embeddings. Vast experiments are conducted on eight real social networks to analyze the effectiveness of the SRFA-GRL model, and the experimental results show that the SRFA-GRL framework outperforms baseline methods.
更多查看译文
关键词
graph representation learning,social networks,group influence,srfa-grl
AI 理解论文
溯源树
样例
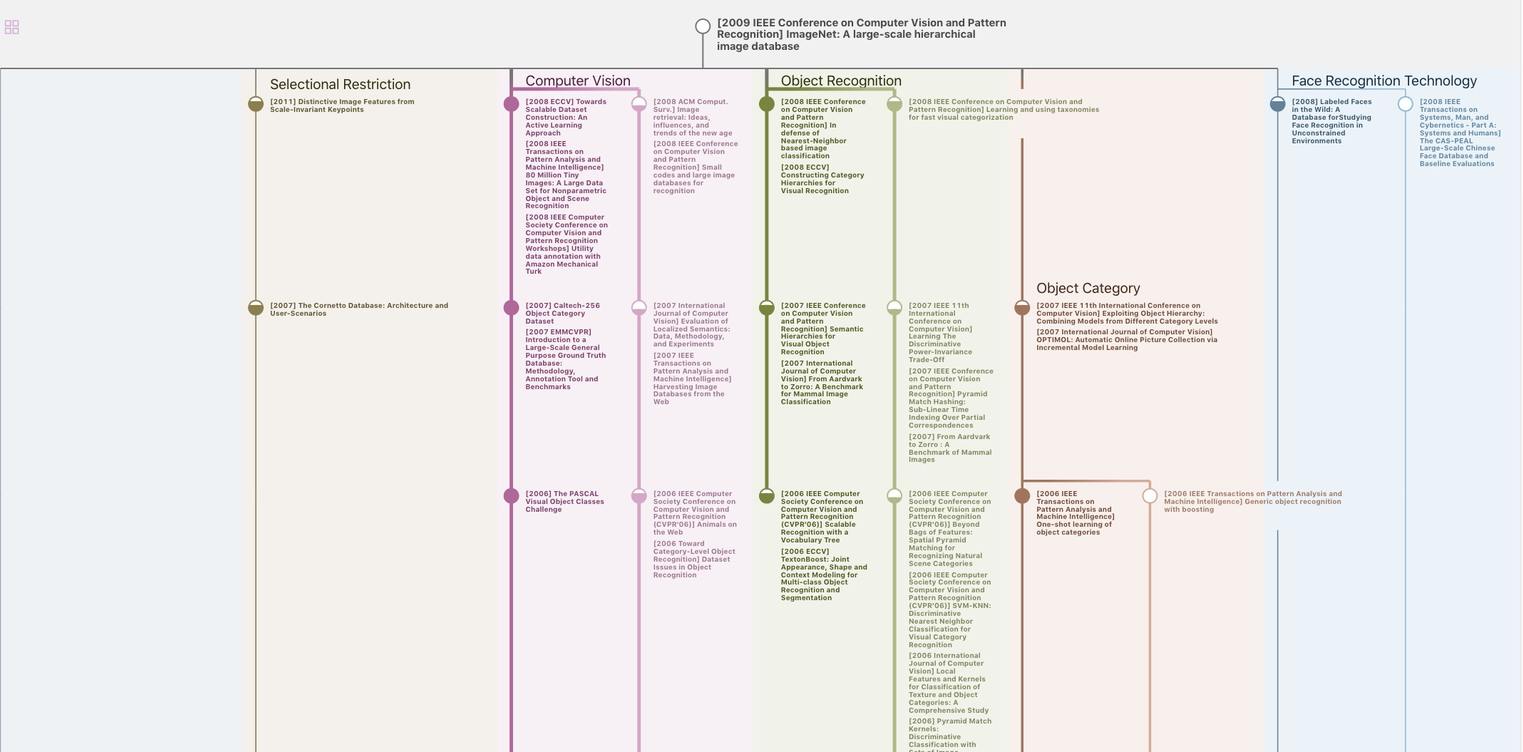
生成溯源树,研究论文发展脉络
Chat Paper
正在生成论文摘要