Anomaly detection for streaming data based on grid-clustering and Gaussian distribution.
Inf. Sci.(2023)
摘要
A massive amount of real-time and evolving streaming data are produced from various devices and applications. Anomaly detection is one of the main tasks of streaming data mining with many practical applications. However, without prior knowledge, it is difficult to detect the anomaly accurately and quickly. In this paper, we propose an unsupervised anomaly detection algorithm (GC-ADS), which is based on the idea of grid clustering and Gaussian distribution. Specifically, the data space is first segmented using the grid structure, then the data points are mapped to grids and finally grids are clustered. The anomaly can be preliminarily judged according to the cluster density. To solve the problem that clustering cannot distinguish between noise and anomaly, based on the idea of data similarity and Gaussian distribution, a noise recognition model is designed. In addition, a data filtering model based on grid and sliding window is designed to save memory and retain valid information. The proposed method is compared with the state-of-the-art methods on the Numenta anomaly benchmark. Experimental results indicate that GC-ADS detects anomalies more accurately than other methods with less time cost.
更多查看译文
关键词
Streaming data,Anomaly detection,Real-time,Unsupervised learning,Grid-clustering
AI 理解论文
溯源树
样例
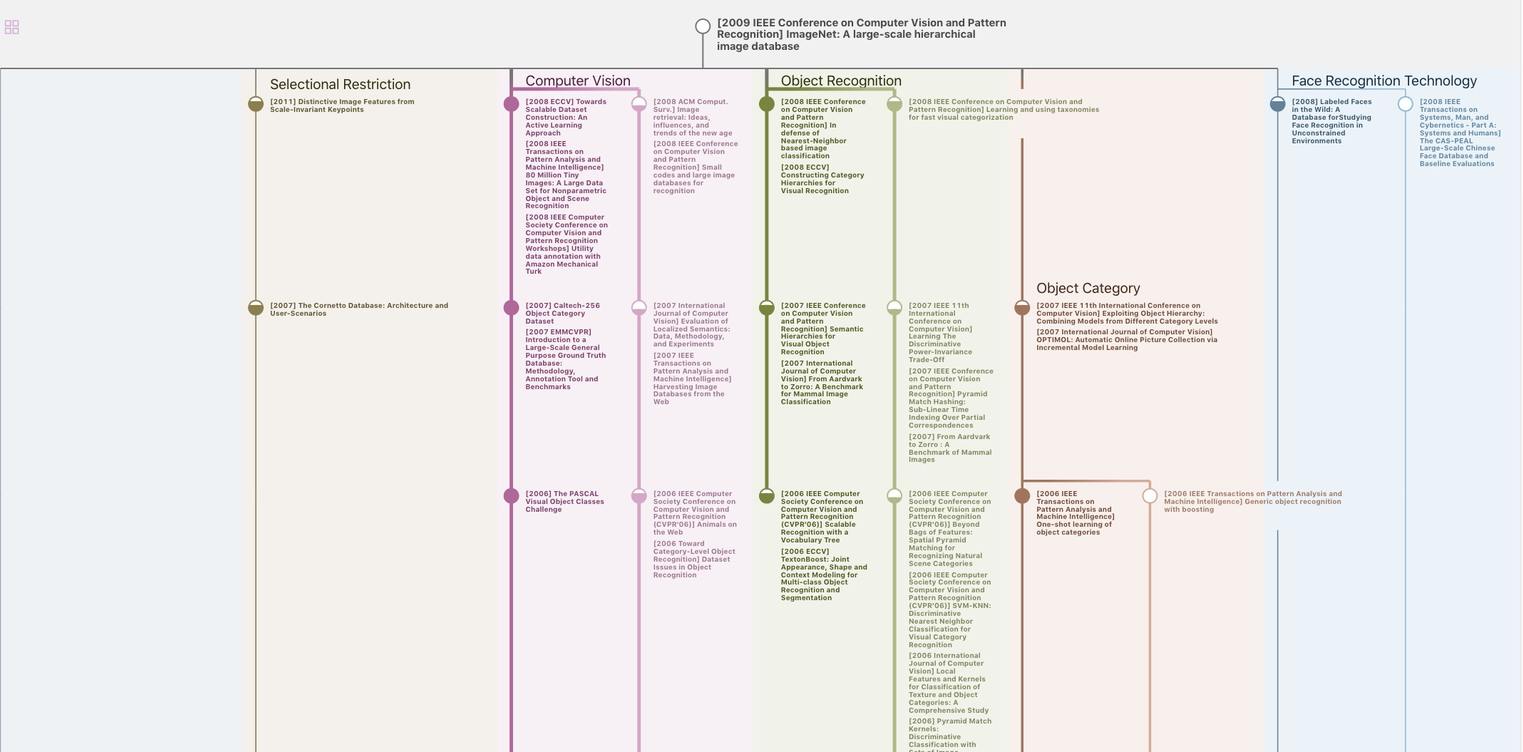
生成溯源树,研究论文发展脉络
Chat Paper
正在生成论文摘要