Consensus-based distributed moving horizon estimation with constraints
Inf. Sci.(2023)
摘要
This paper concerns distributed state estimation for linear systems over a sensor network. Two novel algorithms utilizing the framework of moving horizon estimation (MHE) are proposed, which are fully distributed, scalable and allow for taking into account constraints on the noises and the system states. The proposed methods estimate the state by minimizing a local quadratic objective function, which can be efficiently solved by quadratic programming (QP). Consensus technique is employed to fuse information to construct the local quadratic objective function for each node. The first algorithm, which minimizes a quadratic function involving consensus on measurement costs (CM), approaches the centralized MHE with a sufficiently large number of consensus steps. The second one involving consensus both on arrival costs (CA) and measurement costs, enjoys the benefits of both the CA and CM. To avoid directly running consensus on some functions, a novel consensus strategy for the CM is developed. The estimation errors of the proposed methods are proven to be stochastically ultimately bounded under certain conditions. Finally, numerical results are presented to verify the effectiveness of the developed algorithms.
更多查看译文
关键词
Distributed estimation,Moving horizon estimation,Consensus,Sensor network,Stability analysis
AI 理解论文
溯源树
样例
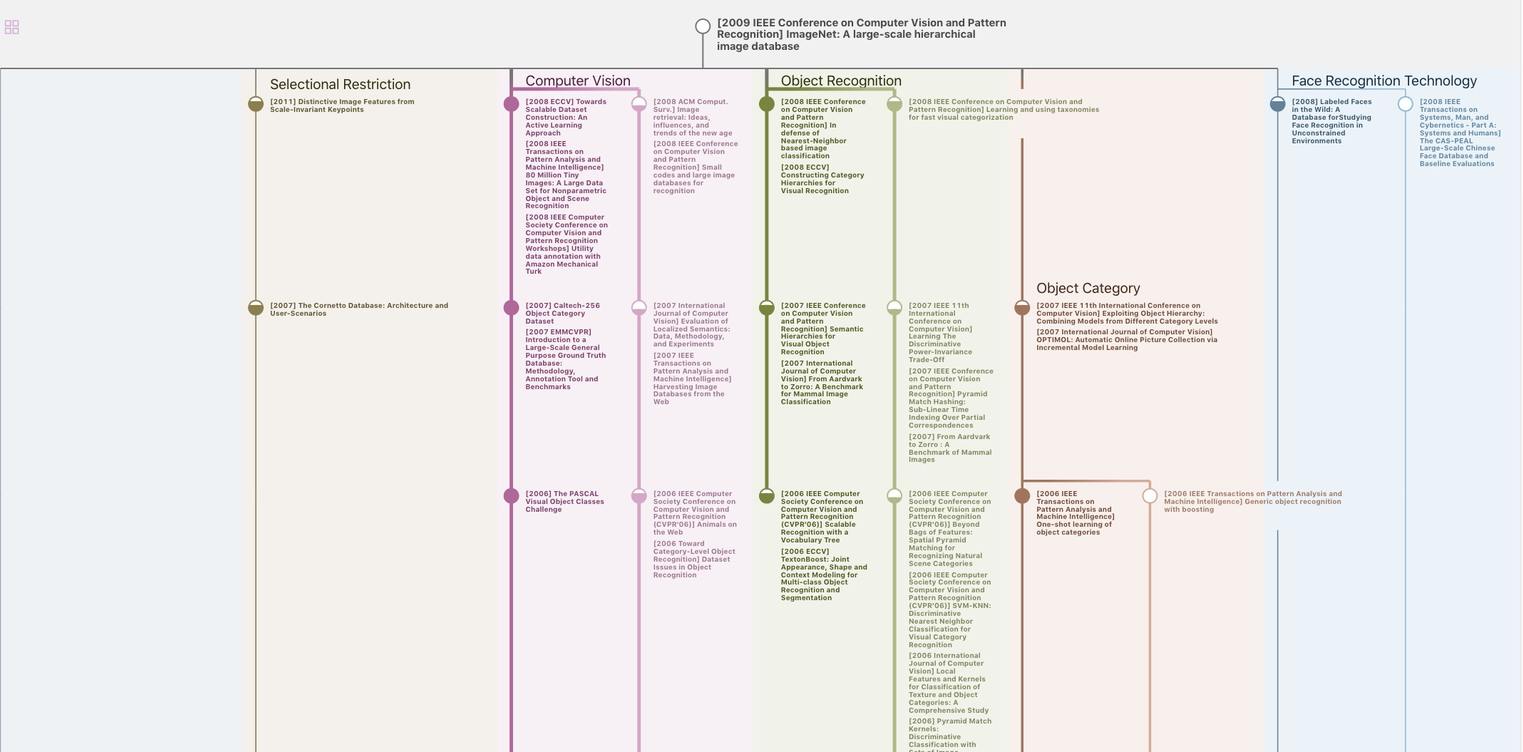
生成溯源树,研究论文发展脉络
Chat Paper
正在生成论文摘要