A Cross-direction Task Decoupling Network for Small Logo Detection.
CoRR(2023)
摘要
Logo detection plays an integral role in many applications. However, handling small logos is still difficult since they occupy too few pixels in the image, which burdens the extraction of discriminative features. The aggregation of small logos also brings a great challenge to the classification and localization of logos. To solve these problems, we creatively propose Cross-direction Task Decoupling Network (CTDNet) for small logo detection. We first introduce Cross-direction Feature Pyramid (CFP) to realize cross-direction feature fusion by adopting horizontal transmission and vertical transmission. In addition, Multi-frequency Task Decoupling Head (MTDH) decouples the classification and localization tasks into two branches. A multi-frequency attention convolution branch is designed to achieve more accurate regression by combining discrete cosine transform and convolution creatively. Comprehensive experiments on four logo datasets demonstrate the effectiveness and efficiency of the proposed method.
更多查看译文
关键词
object detection,logo detection,multi-scale feature,attention
AI 理解论文
溯源树
样例
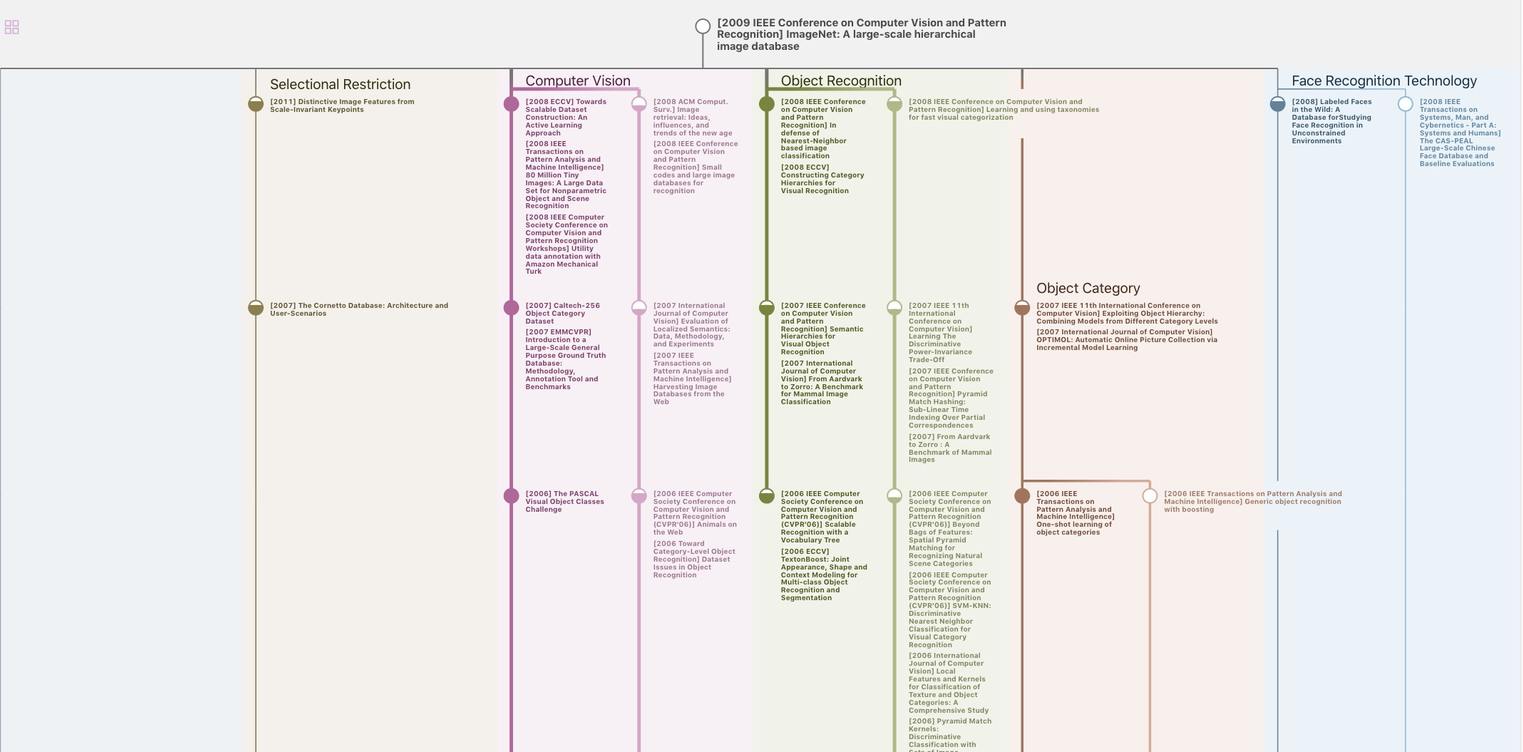
生成溯源树,研究论文发展脉络
Chat Paper
正在生成论文摘要