Constructing Low-Redundant and High-Accuracy Knowledge Graphs for Education.
ICWL(2022)
摘要
Motivated by the successful applications of commonsense knowledge graphs (KGs) and encyclopedia KGs, many KG-based applications have been developed in education, such as course content visualization and learning path/material recommendations. While KGs for education are often constructed manually, attempts have been made to leverage machine learning algorithms to extract triples from teaching materials. However, education-related KGs learned by existing algorithms contain significant amounts of redundancy and noise. It is because the entities and relations in teaching materials are often instructional, abstract, and implicit, while textbooks often contain detailed explanations, examples, and illustrations. Off-the-shelf KG construction algorithms are designed for concrete entities. To this end, we propose an effective framework to construct low-redundant and high-accuracy KGs for education. First, we design an ontology that is tailored for education. By choosing related Wikidata items, we construct an instructional entity set. We avoid using traditional methods such as named-entity recognition to extract entities from textbooks, aiming to reduce redundancy. Then, we add subtopic relations among our selected instructional entities based on the corresponding hierarchy in Wikidata, and form a backbone. Second, we design a machine reading comprehension model with pre-defined questions to extract other types of relations, such as equivalent to, applied to, and inventor of. Third, we apply active KG error detection to further refine the KG with minimal human effort. In the experiments, we take the artificial intelligence domain as an example and demonstrate the effectiveness of the proposed framework. Our KG achieves an accuracy of around 80% scored by domain experts.
更多查看译文
关键词
knowledge,education,low-redundant,high-accuracy
AI 理解论文
溯源树
样例
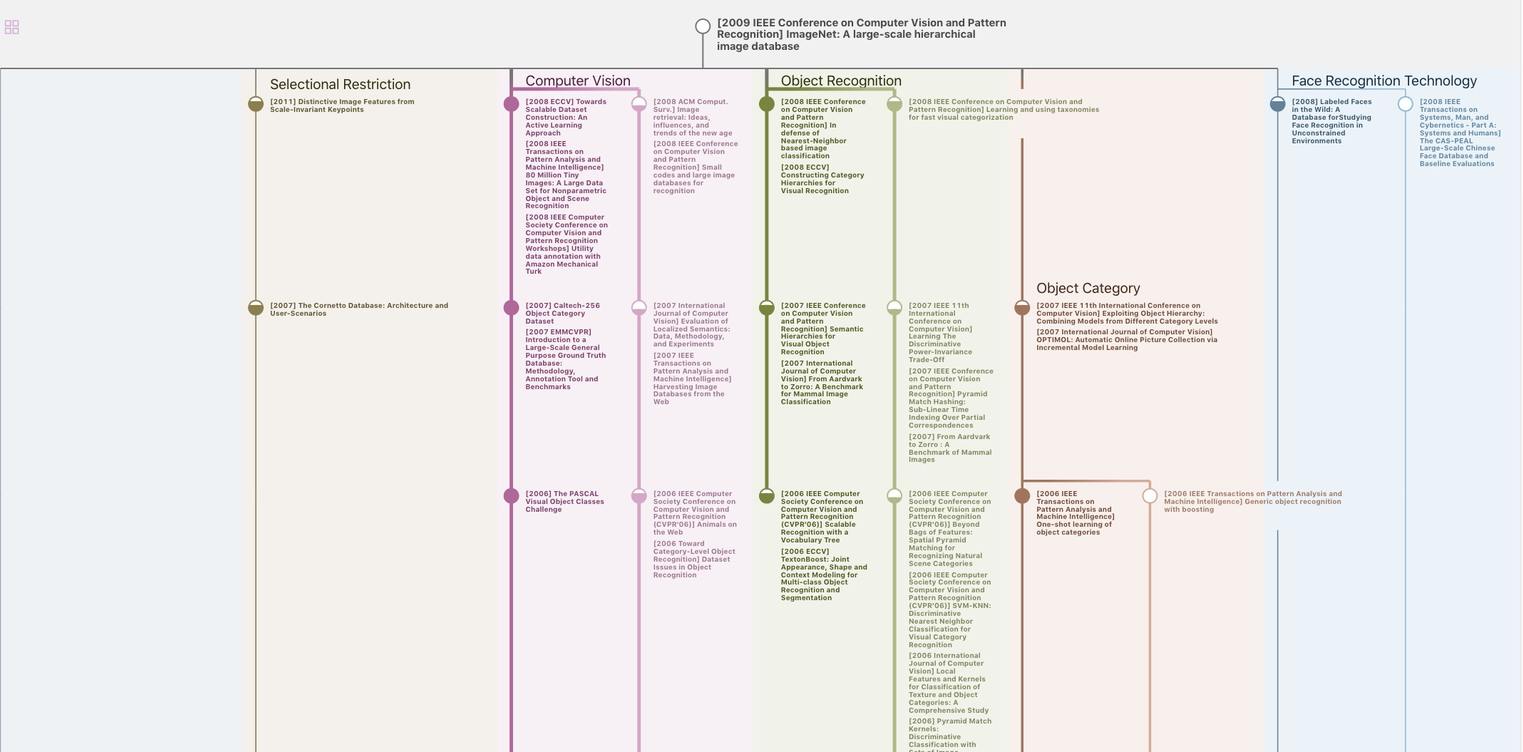
生成溯源树,研究论文发展脉络
Chat Paper
正在生成论文摘要