Leveraging Interactive Paths for Sequential Recommendation.
DASFAA (2)(2023)
摘要
Sequential recommendation systems dynamically predict the users’ next behaviors from chronological historical records to provide more accurate recommendations. However, in many real-world scenarios, recommenders find it difficult to make recommendations for cold-start users who have limited interactions with items. To address this issue, existing methods utilize auxiliary information to capture user preferences, which have achieved performance improvement to some extent. But with the gradual awareness of personal privacy, external information becomes difficult to obtain. Therefore, we develop a novel model called Path-based sequential Recommender for cold-start users (PathRec). PathRec exploits the rich sequential dependencies within the interactive paths to give reasonable recommendations for cold-start users without additional external information. Specifically, (i) PathRec constructs the global graph to learn the representations of the items. (ii) Then, PathRec elaborately selects the sampled paths to derive the underlying dependencies of the cold-start sequence. (iii) Finally, PathRec provides rich and complementary information to user-item interactions by strongly coupling the paths to give suitable recommendations. Extensive experiments on three datasets demonstrate the effectiveness of PathRec. In particular, our model can adapt to the cold-start users and has potential interpretability for recommendations.
更多查看译文
关键词
interactive paths,recommendation
AI 理解论文
溯源树
样例
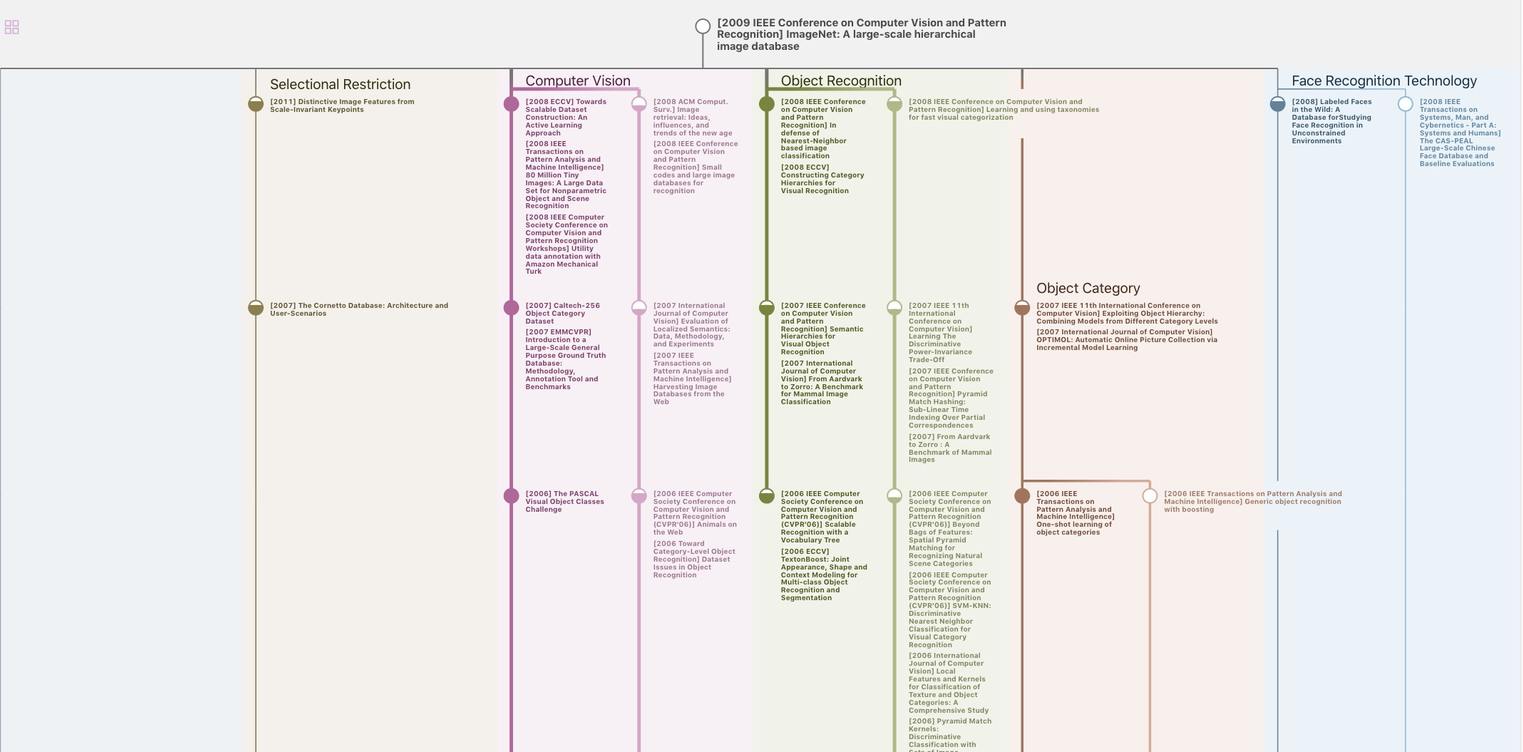
生成溯源树,研究论文发展脉络
Chat Paper
正在生成论文摘要