NR-MVSNet: Learning Multi-View Stereo Based on Normal Consistency and Depth Refinement
IEEE Trans. Image Process.(2023)
摘要
Multi-view Stereo (MVS) aims to reconstruct a 3D point cloud model from multiple views. In recent years, learning-based MVS methods have received a lot of attention and achieved excellent performance compared with traditional methods. However, these methods still have apparent shortcomings, such as the accumulative error in the coarse-to-fine strategy and the inaccurate depth hypotheses based on the uniform sampling strategy. In this paper, we propose the NR-MVSNet, a coarse-to-fine structure with the depth hypotheses based on the normal consistency (DHNC) module, and the depth refinement with reliable attention (DRRA) module. Specifically, we design the DHNC module to generate more effective depth hypotheses, which collects the depth hypotheses from neighboring pixels with the same normals. As a result, the predicted depth can be smoother and more accurate, especially in texture-less and repetitive-texture regions. On the other hand, we update the initial depth map in the coarse stage by the DRRA module, which can combine attentional reference features and cost volume features to improve the depth estimation accuracy in the coarse stage and address the accumulative error problem. Finally, we conduct a series of experiments on the DTU, BlendedMVS, Tanks & Temples, and ETH3D datasets. The experimental results demonstrate the efficiency and robustness of our NR-MVSNet compared with the state-of-the-art methods. Our implementation is available at https://github.com/wdkyh/NR-MVSNet.
更多查看译文
关键词
Costs,Three-dimensional displays,Image reconstruction,Feature extraction,Task analysis,Point cloud compression,Estimation,Multi-view stereo,deep learning,3D model reconstruction,normal consistency,feature attention,plane sweep algorithm
AI 理解论文
溯源树
样例
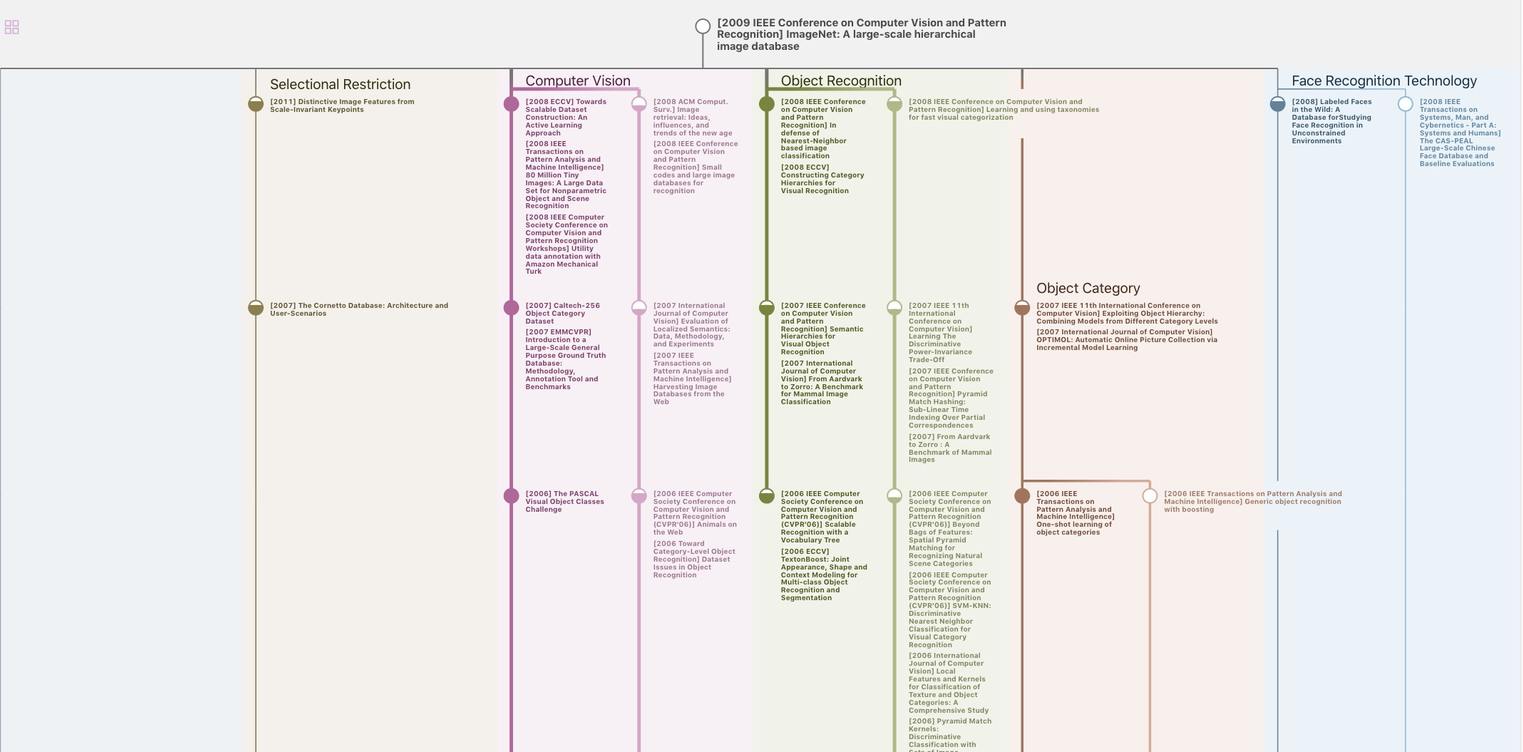
生成溯源树,研究论文发展脉络
Chat Paper
正在生成论文摘要