ADMM-SRNet: Alternating Direction Method of Multipliers Based Sparse Representation Network for One-Class Classification.
IEEE Trans. Image Process.(2023)
摘要
One-class classification aims to learn one-class models from only in-class training samples. Because of lacking out-of-class samples during training, most conventional deep learning based methods suffer from the feature collapse problem. In contrast, contrastive learning based methods can learn features from only in-class samples but are hard to be end-to-end trained with one-class models. To address the aforementioned problems, we propose alternating direction method of multipliers based sparse representation network (ADMM-SRNet). ADMM-SRNet contains the heterogeneous contrastive feature (HCF) network and the sparse dictionary (SD) network. The HCF network learns in-class heterogeneous contrastive features by using contrastive learning with heterogeneous augmentations. Then, the SD network models the distributions of the in-class training samples by using dictionaries computed based on ADMM. By coupling the HCF network, SD network and the proposed loss functions, our method can effectively learn discriminative features and one-class models of the in-class training samples in an end-to-end trainable manner. Experimental results show that the proposed method outperforms state-of-the-art methods on CIFAR-10, CIFAR-100 and ImageNet-30 datasets under one-class classification settings.
更多查看译文
关键词
One-class classification,contrastive learning,dictionary learning,deep learning,sparse representation
AI 理解论文
溯源树
样例
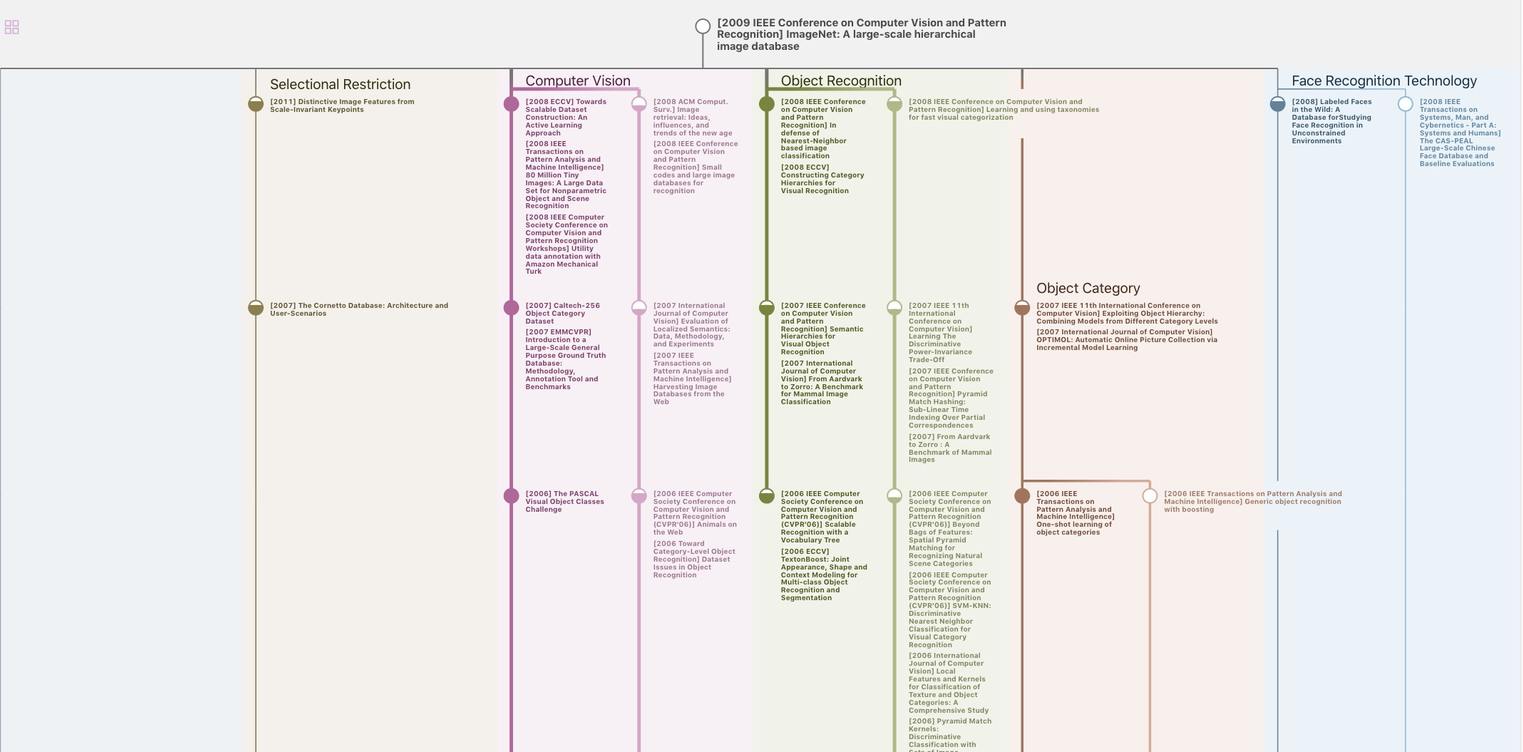
生成溯源树,研究论文发展脉络
Chat Paper
正在生成论文摘要