DKFM: Dual Knowledge-Guided Fusion Model for Drug Recommendation.
PAKDD (3)(2023)
摘要
Drug recommendation is an AI healthcare task that has become increasingly important during the current COVID-19 pandemic. At present, the accumulation of a large number of electronic health records (EHR) provides strong data support for medical and scientific researchers. Most existing work uses splicing diagnosis and procedure to complete the recommendation task while ignoring the problem that the patient’s diagnosis and procedure information are recorded in different levels of detail. Moreover, the splicing of different medical records cannot accurately characterize the patient’s condition, thus reducing the accuracy of recommended drugs. In this paper, we propose a drug recommendation model, DKFM, which introduces a molecular knowledge-guided dual-level drug fusion mechanism. DKFM can integrate a patient’s diagnosis and procedure important information and determine the impact on the current condition. Then the diagnosis and procedure information was respectively injected into the drug functional group encoder to obtain two candidate drug sets. Finally, select the drugs that are really suitable for the patient’s condition at the sets level and the score matrix level as the final recommended drugs. We validate DKFM on the public MIMIC-III dataset, and experimental results show that the proposed model can outperform the state-of-the-art approaches.
更多查看译文
关键词
fusion model,knowledge-guided
AI 理解论文
溯源树
样例
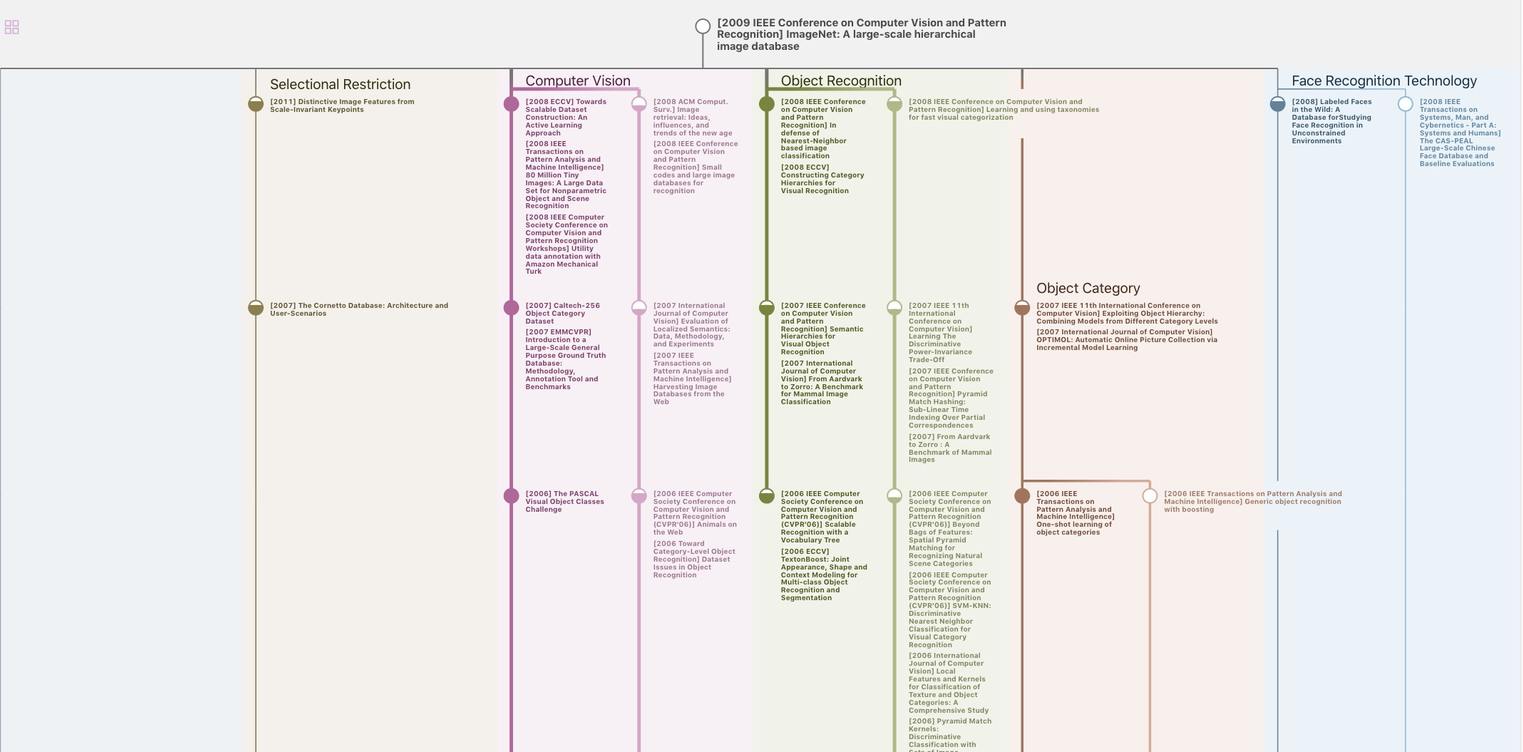
生成溯源树,研究论文发展脉络
Chat Paper
正在生成论文摘要