Multi-Agent Meta-Reinforcement Learning with Coordination and Reward Shaping for Traffic Signal Control.
PAKDD (2)(2023)
摘要
Traffic signal control (TSC) plays an important role in alleviating heavy traffic congestion problem. It is helpful to provide an effective transportation system by optimizing traffic signals intelligently. Recently, many deep reinforcement learning methods are proposed to solve TSC. However, most of these methods are trained and tested in a fixed roadnet with the same traffic flow environment. They can not adapt to new environments. Some meta-reinforcement learning methods are proposed to solve this problem, but they can not properly decide when to coordinate traffic signals. This paper proposes a multi-agent meta-reinforcement learning method with coordination and reward shaping to solve TSC. The proposed method combines independent learning and neighbor-aware learning to adapt to different TSC environments. Besides, the proposed method constructs a novel reward shaping. The reward shaping can enhance traffic efficiency by encouraging adjacent intersections to generate more green waves. Based on green waves, vehicles can go straight through multiple intersections without stopping. Experimental results demonstrate that the proposed method achieves the state-of-the-art generalization performance on synthetic and real-world datasets.
更多查看译文
关键词
reward shaping,traffic,multi-agent,meta-reinforcement
AI 理解论文
溯源树
样例
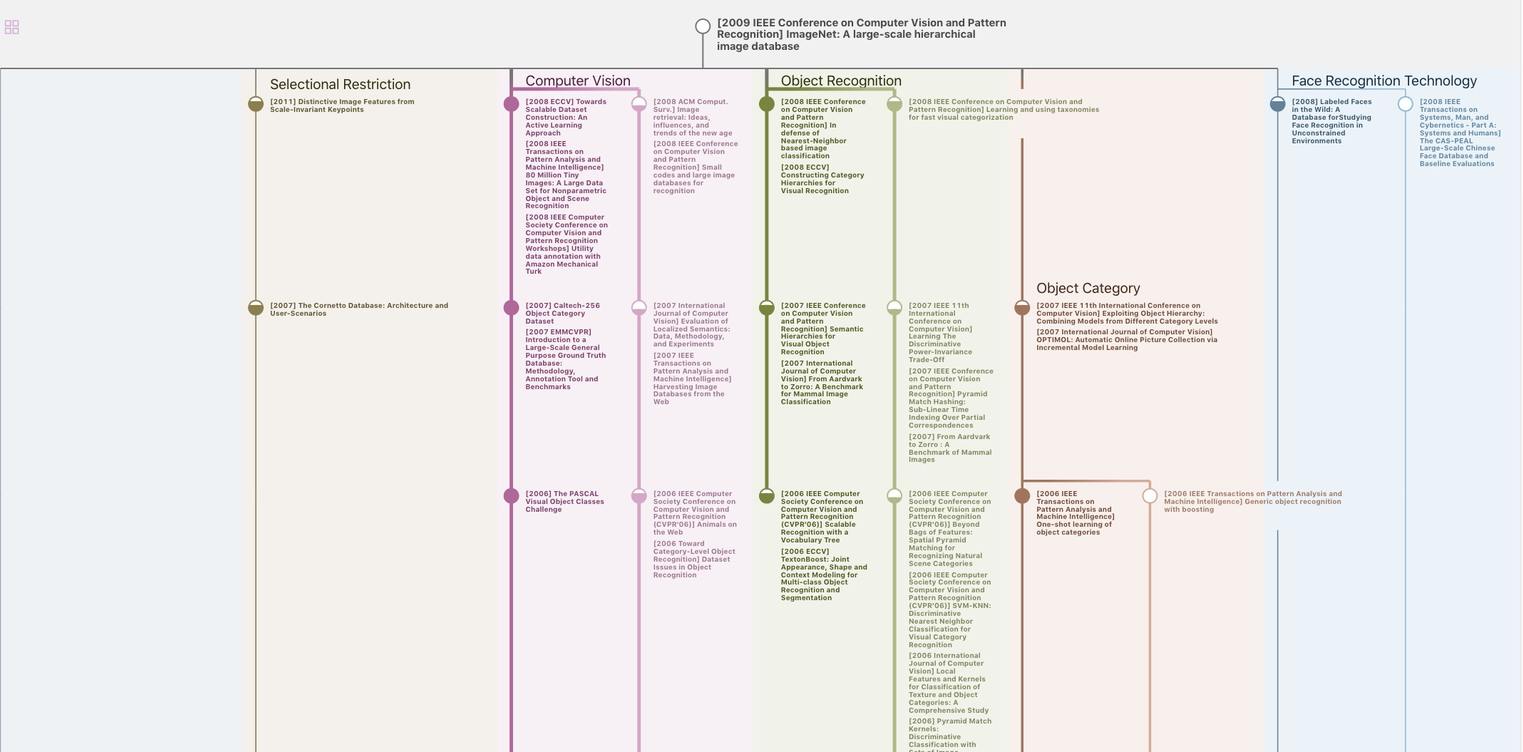
生成溯源树,研究论文发展脉络
Chat Paper
正在生成论文摘要