Knowledge-Enhanced Prototypical Network with Structural Semantics for Few-Shot Relation Classification.
PAKDD (3)(2023)
摘要
Few-shot relation classification (RC) aims to determine the labeled relation between two entities in a given sentence using only a few training instances. Previous studies integrate models with explicit triple knowledge, using the inherent concepts of entities to improve the instance representation. However, these studies neglect the implicit structural knowledge present in the knowledge graph (KG). In this paper, we present SKProto, a knowledge-enhanced prototypical network that leverages deep structured semantic knowledge from the multi-hop neighbors of entity-linked concepts. Specifically, we propose a concept-guided hybrid attention mechanism to learn implicit structural semantic knowledge for enhancing the context-aware instance representation. To further distinguish subtle semantic differences among the concepts, the multi-granularity semantic distinction approach is proposed to construct the negative samples with various difficulties (i.e. hard, medium, and easy) based on the conceptual hierarchical structure. Experimental results on the FewRel 2.0 benchmark show that SKProto outperforms state-of-the-art models. We also demonstrate that SKProto has better robustness than other competitive models in low-shot scenarios.
更多查看译文
关键词
structural semantics,relation,knowledge-enhanced,few-shot
AI 理解论文
溯源树
样例
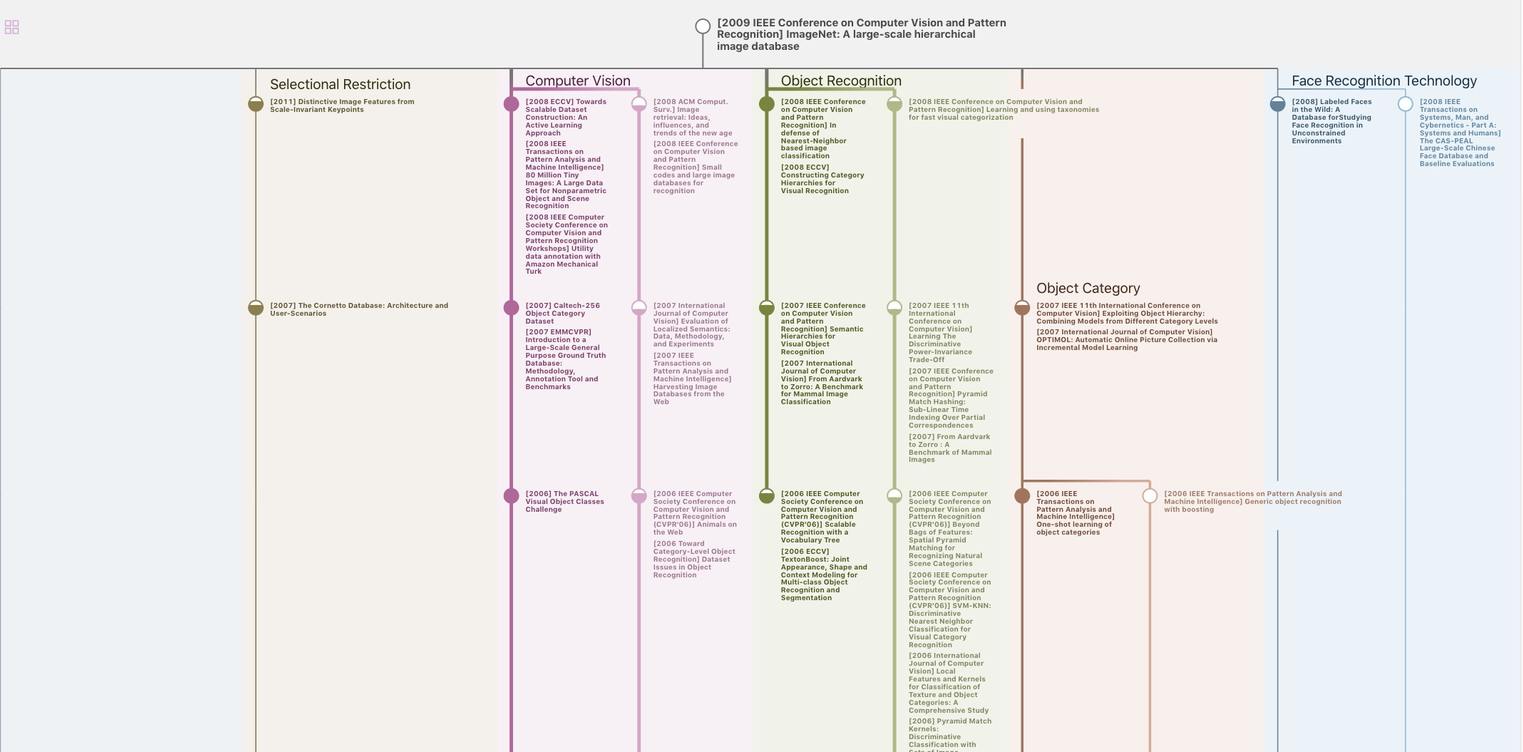
生成溯源树,研究论文发展脉络
Chat Paper
正在生成论文摘要