A2TP: Aggregator-aware In-network Aggregation for Multi-tenant Learning
EuroSys(2023)
摘要
Distributed Machine Learning (DML) techniques are widely used to accelerate the training of large-scale machine learning models. However, during training iterations, gradients need to be frequently aggregated across multiple workers, resulting in communication bottleneck. To reduce the communication overhead of DML, several In-Network Aggregation (INA) protocols are proposed to reduce the volume of aggregation traffic by offloading aggregation functions into switches, thus alleviating network bottlenecks. Nevertheless, these protocols couple the congestion control of in-switch aggregator resources and link bandwidth resources, together with the straggler-oblivious manner in aggregator allocation, leading to low aggregation efficiency. To solve the above problem, we propose an Aggregator-aware in-networkAggregation Transmission Protocol (A(2)TP), which adopts two congestion windows to decouple the congestion control of two resources and combines with the straggling estimation scheme to efficiently allocate aggregator resources according to the straggler degree for multiple jobs, eliminating the impact of straggler jobs on the overall aggregation process. We implement A(2)TP at P4-programmable switch and a kernel bypass protocol stack at the end-host. The evaluation results show that A(2)TP reduces the training time by up to 66% than the state-of-the-art INA protocols in real-world benchmark models.
更多查看译文
关键词
in-network aggregation,machine learning,transmission protocol
AI 理解论文
溯源树
样例
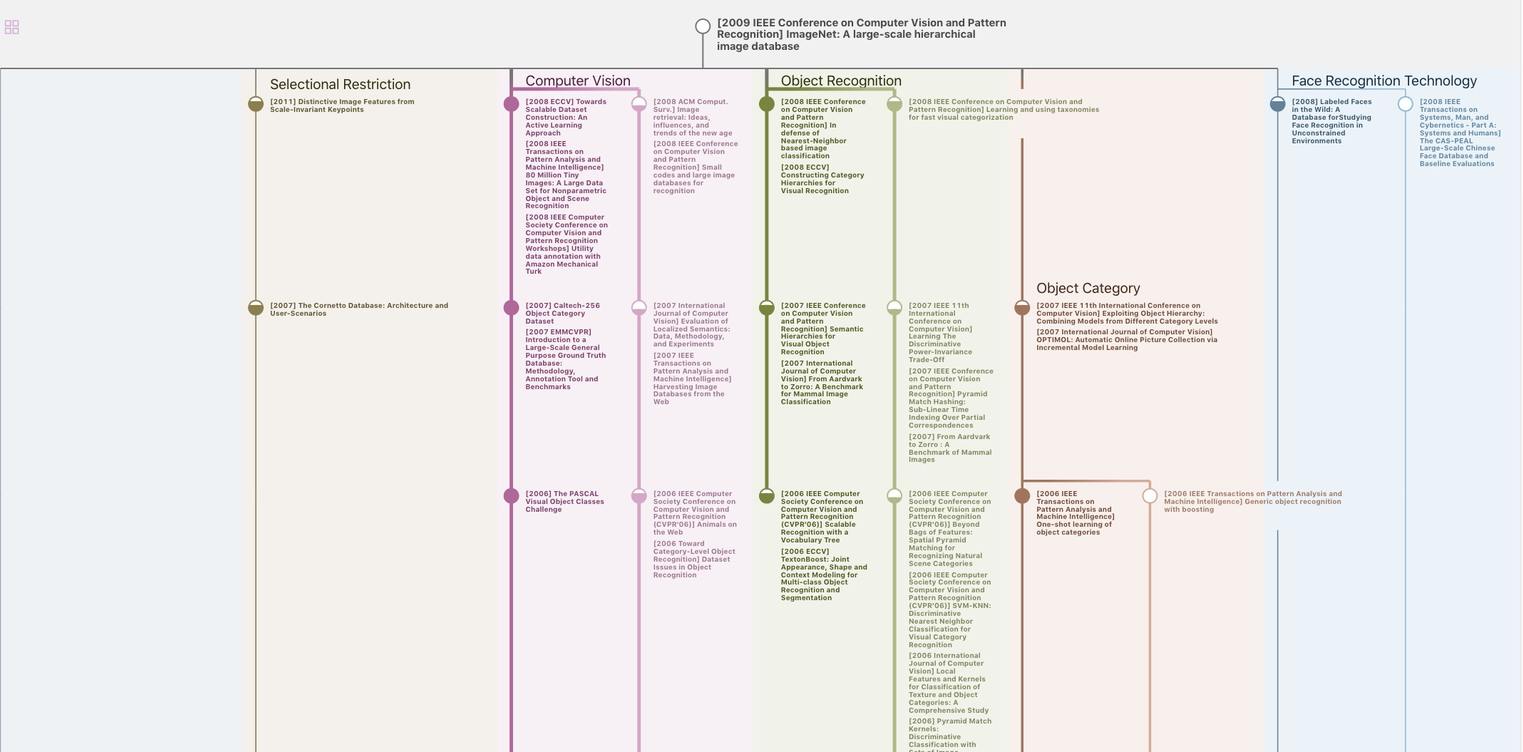
生成溯源树,研究论文发展脉络
Chat Paper
正在生成论文摘要