Detecting Outliers in CI/CD Pipeline Logs Using Latent Dirichlet Allocation
ENASE(2023)
摘要
Continuous Integration and Continuous Delivery are best practices used in the context of DevOps. By using automated pipelines for building and testing small software changes, possible risks are intended to be detected early. Those pipelines continuously generate log events that are collected in semi-structured log files. In practice, these log files can amass 100 000 events and more. However, the relevant sections in these log files must be manually tagged by the user. This paper presents an online learning approach for detecting relevant log events using Latent Dirichlet Allocation. After grouping a fixed number of log events in a document, our approach prunes the vocabulary to eliminate words without semantic meaning. A sequence of documents is then described as a discrete sequence by applying Latent Dirichlet Allocation, which allows the detection of outliers within the sequence. By integrating the latent variables of the model, our approach provides an explanation of its prediction. Our experiments show that our approach is sensitive to the choice of its hyperparameters in terms of the number and choice of detected anomalies.
更多查看译文
关键词
Log Analysis,Anomaly Detection,Event-Streaming,Latent Dirichlet Allocation
AI 理解论文
溯源树
样例
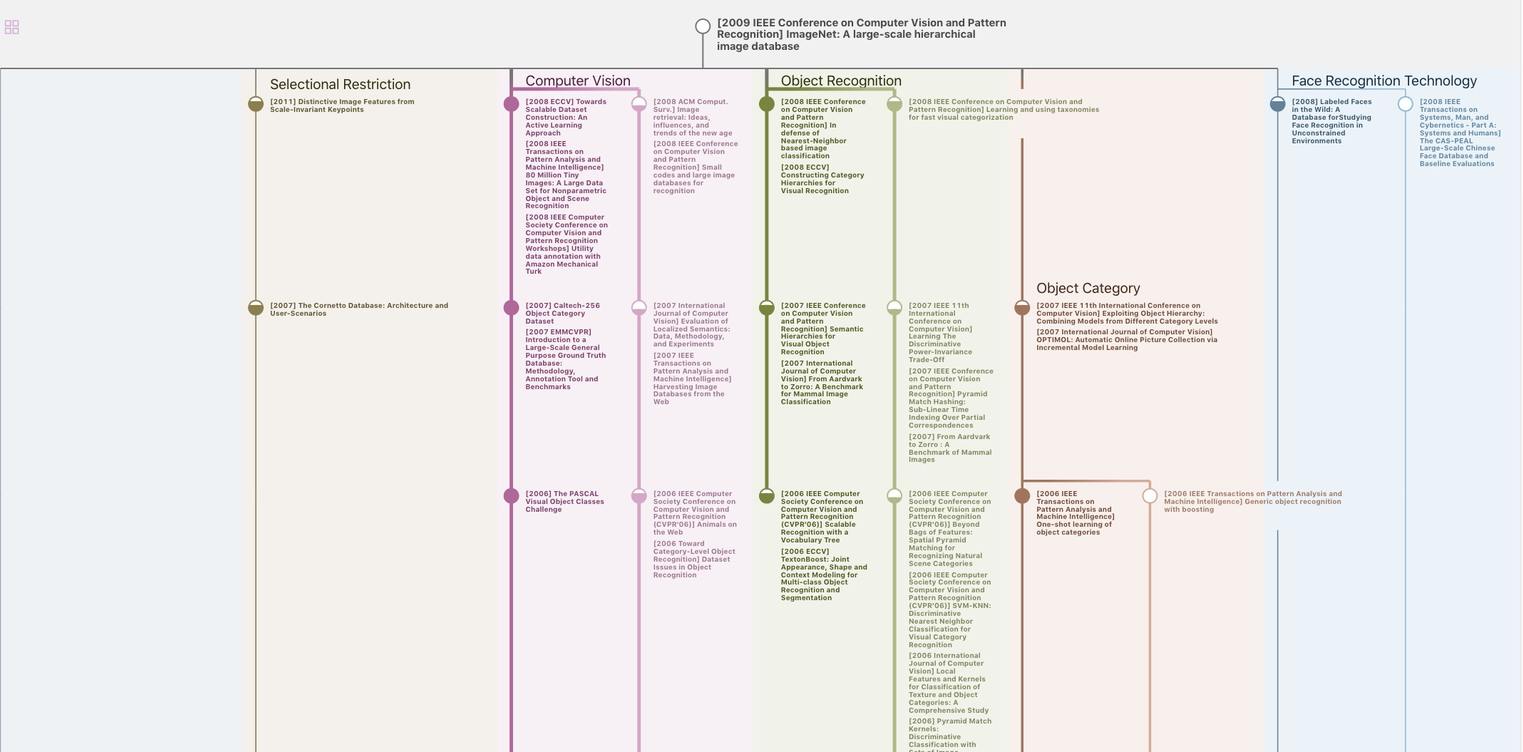
生成溯源树,研究论文发展脉络
Chat Paper
正在生成论文摘要