Modelling Data-Aware Stochastic Processes - Discovery and Conformance Checking.
Petri Nets(2023)
摘要
Process mining aims to analyse business process behaviour by discovering process models such as Petri nets from process executions recorded as sequential traces in event logs. Such discovered Petri nets capture the process behaviour observed in a log but do not provide insights on the likelihood of behaviour: the stochastic perspective. A stochastic Petri net extends a Petri net to explicitly encode the occurrence probabilities of transitions. However, in a real-life processes, the probability of a trace may depend on data variables: e.g., a higher requested loan amount will trigger additional checks. Such dependencies are not described by current stochastic Petri nets and corresponding stochastic process mining techniques. We extend stochastic Petri nets with data-dependent transition weights and provide a technique for learning them from event logs. We discuss how to evaluate the quality of these discovered models by deriving a stochastic data-aware conformance checking technique. The implementations are available in ProM, and we show on real-life event logs that the discovery technique is competitive with existing stochastic process discovery approaches, and that new types of stochastic data-based insights can be derived.
更多查看译文
关键词
stochastic processes,discovery,modelling,data-aware
AI 理解论文
溯源树
样例
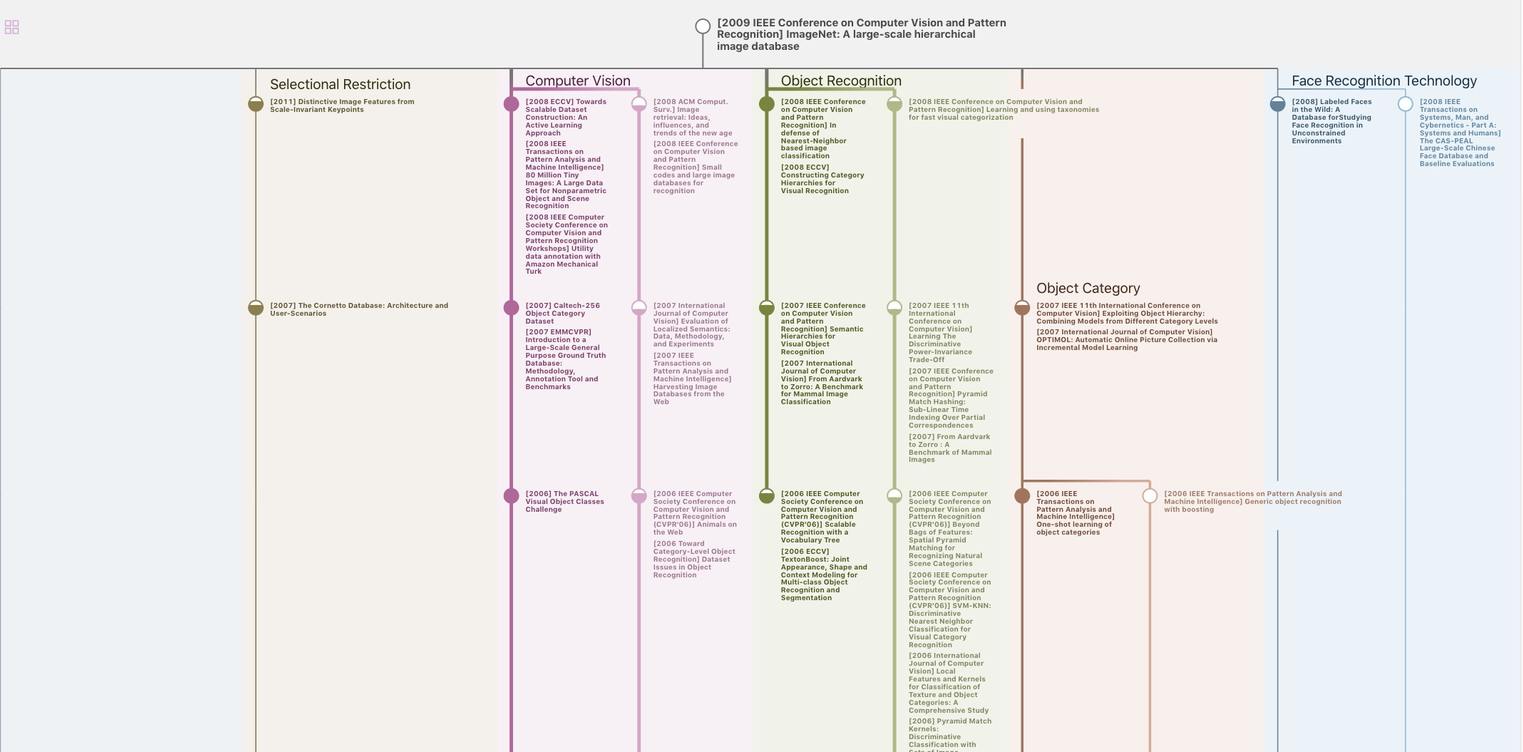
生成溯源树,研究论文发展脉络
Chat Paper
正在生成论文摘要