Anomaly Detection for Hyperspectral Imagery via Tensor Low-Rank Approximation With Multiple Subspace Learning.
IEEE Trans. Geosci. Remote. Sens.(2023)
摘要
Hyperspectral anomaly detection (HAD) is regarded as an indispensable, pivotal technology in remote sensing and Earth science domains. Nevertheless, most existing detection approaches for anomaly targets flatten 3-D hyperspectral images (HSIs) with spatial and spectral information into 2-D spectral vector data, which virtually breaks up the internal spatial structure in HSIs and degenerates the detection performance. To this end, we directly consider the HSI data cube as a 3-D tensor and develop a novel tensor low-rank approximation (TLRA) detection algorithm to separate the sparse anomalous component from the background with low-rank characteristics. Then, in light of the multisubspace structure in heterogeneous backgrounds, we utilize multiple subspace learning (MSL) theory to encode the background tensor with a coefficient tensor and corresponding dictionary tensor. In addition, considering that different singular values indicate different information quantities and should be penalized to different extents, we introduce a tighter tensor rank surrogate named the E-shrinkage tensor nuclear norm (E-TNN) to recover the low-rank component more accurately. Meanwhile, concerning the sparse anomaly target, the l(2,1) constraint is incorporated to represent the group sparsity of the abnormal component. Finally, an effective iterative optimization algorithm based on the alternating direction method of multipliers (ADMM) is devised to solve the proposed TLRA-MSL model. We conduct extensive experiments on six hyperspectral datasets to prove the effectiveness and robustness of our method. The experimental results illustrate that better detection performance is obtained using the proposed model compared with other state-of-the-art algorithms.
更多查看译文
关键词
ϵ-shrinkage tensor nuclear norm (ϵ-TNN),anomaly detection,hyperspectral images (HSIs),multiple subspace learning (MSL),tensor low-rank approximation (TLRA)
AI 理解论文
溯源树
样例
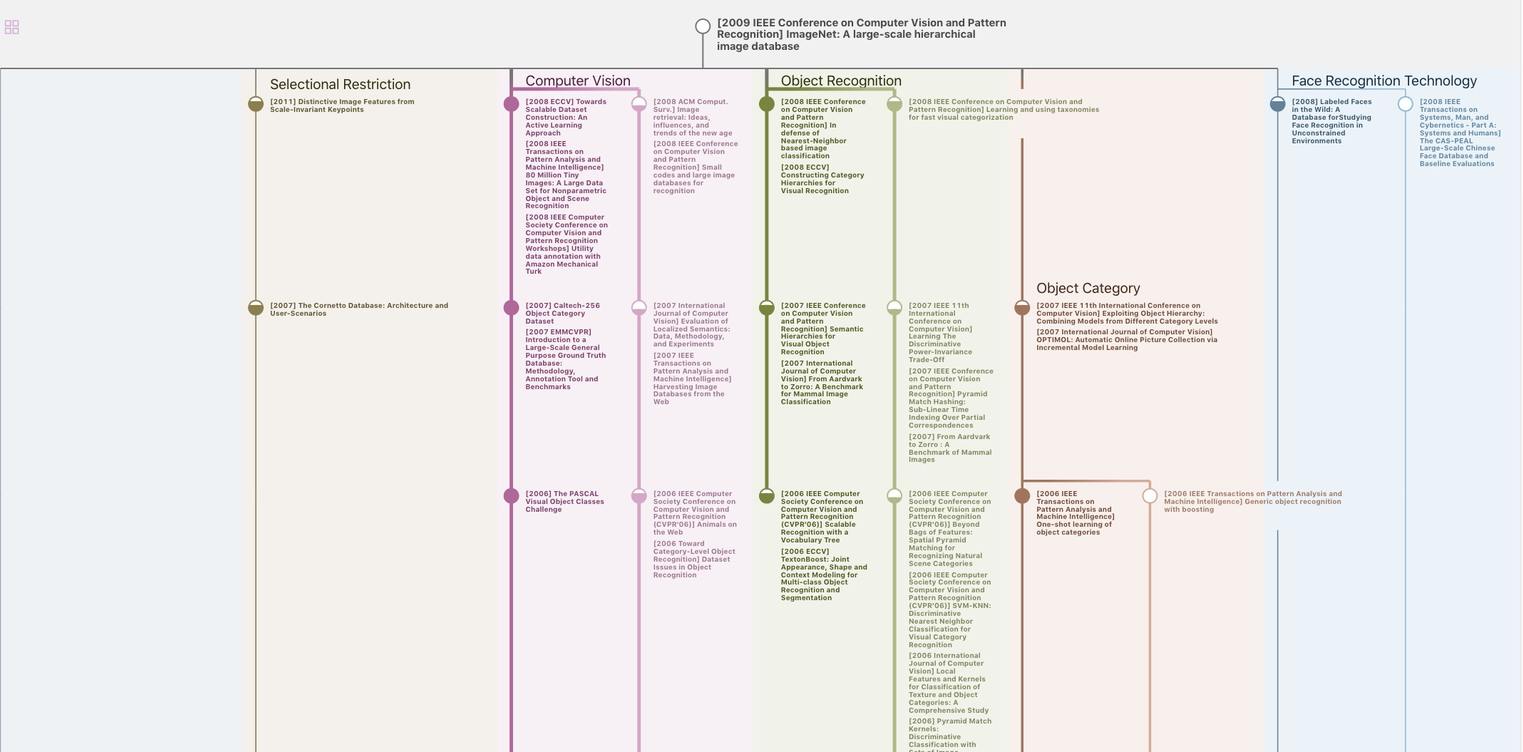
生成溯源树,研究论文发展脉络
Chat Paper
正在生成论文摘要