DCI-PGCN: Dual-Channel Interaction Portable Graph Convolutional Network for Landslide Detection.
IEEE Trans. Geosci. Remote. Sens.(2023)
摘要
Landslide, a kind of destructive natural disaster, often occurs in the mountainous areas of China. Landslide information instant collection plays an important role in taking appropriate remedial measures and personnel evacuation. In recent years, the use of convolutional neural network (CNN) for landslide regional detection achieved good performance; however, most CNN-based methods had no regard for the internal connection of the cover materials in the disaster occurrence area. Moreover, the information revealed by the internal deformation features was ignored, and the same surface object in the image presents different features under different illumination, environment, and resolution, which makes it difficult to extract the structural features of landslide images. In this article, we propose a novel graph convolutional network for landslide detection, inspired by attention mechanism's ability to focus on selective information supplemented with both different channels. The global maximum node connection strategy with positive and negative connectivity makes the graph convolution network (GCN) more portable, which is used as the basic unit of graph feature propagation to construct a multilayer residual connection module. In order to learn interactively and spread graph information, channel dimension is added to make the boundary of features between classes more discriminative. Extensive experiments on Sichuan Province and Bijie landslide datasets show that our proposed method outperforms other detection models and achieves high precision and accuracy. In addition, we also carried out landslide detection for Zhaotong of Yunnan Province on GF-2 original images to prove the effectiveness and applicability of the algorithm.
更多查看译文
关键词
Terrain factors, Feature extraction, Convolution, Convolutional neural networks, Remote sensing, Semantic segmentation, Data mining, Channel interaction, landslide detection, portable graph convolution, semantic segmentation
AI 理解论文
溯源树
样例
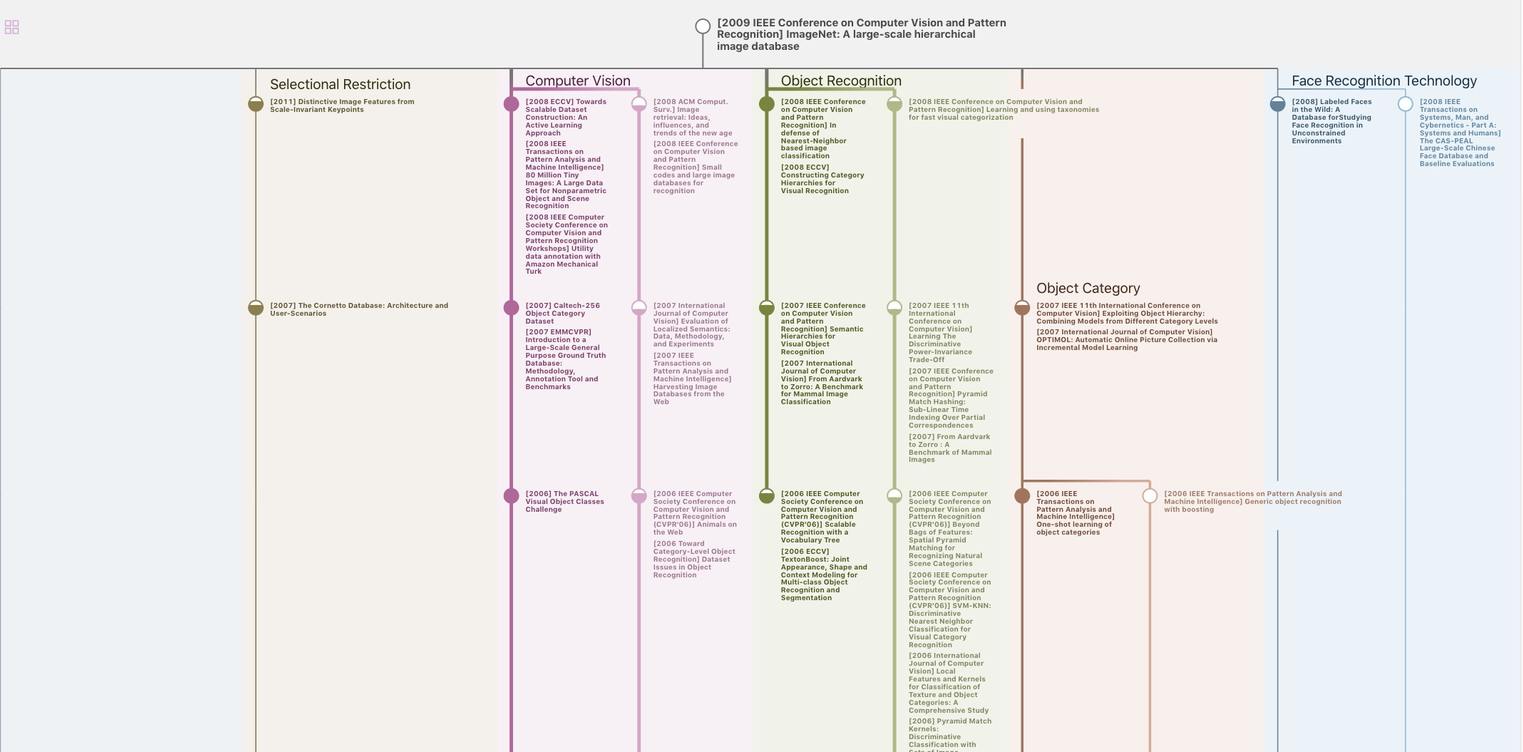
生成溯源树,研究论文发展脉络
Chat Paper
正在生成论文摘要