Query-efficient model extraction for text classification model in a hard label setting.
J. King Saud Univ. Comput. Inf. Sci.(2023)
摘要
Designing a query-efficient model extraction strategy to steal models from cloud-based platforms with black-box constraints remains a challenge, especially for language models. In a more realistic setting, a lack of information about the target model’s internal parameters, gradients, training data, or even confidence scores prevents attackers from easily copying the target model. Selecting informative and useful examples to train a substitute model is critical to query-efficient model stealing. We propose a novel model extraction framework that fine-tunes a pretrained model based on bidirectional encoder representations from transformers (BERT) while improving query efficiency by utilizing an active learning selection strategy. The active learning strategy, incorporating semantic-based diversity sampling and class-balanced uncertainty sampling, builds an informative subset from the public unannotated dataset as the input for fine-tuning. We apply our method to extract deep classifiers with identical and mismatched architectures as the substitute model under tight and moderate query budgets. Furthermore, we evaluate the transferability of adversarial examples constructed with the help of the models extracted by our method. The results show that our method achieves higher accuracy with fewer queries than existing baselines and the resulting models exhibit a high transferability success rate of adversarial examples.
更多查看译文
关键词
Model extraction,Language model stealing,Model privacy,Adversarial attack,Natural language processing,Performance Evaluation
AI 理解论文
溯源树
样例
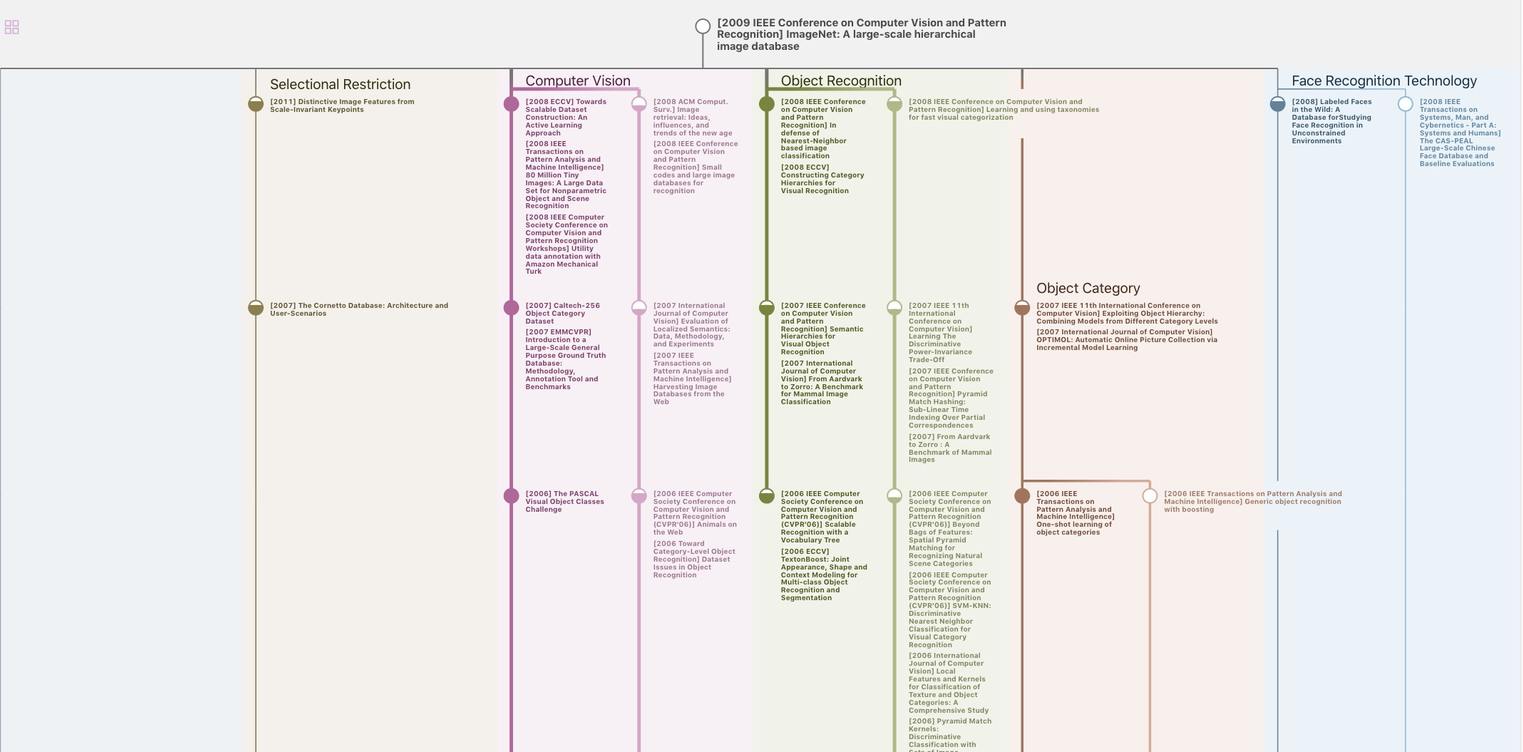
生成溯源树,研究论文发展脉络
Chat Paper
正在生成论文摘要