RSCAT: Towards zero touch congestion control based on actor-critic reinforcement learning and software-defined networking.
J. Netw. Comput. Appl.(2023)
摘要
Network congestion is a phenomenon present in contemporaneous data centers (DCs) independently of scale and underlying technologies. The small-scale presence of congestion causes information delay for the DC hosted services, while in large-scale data losses or even service downtimes are not uncommon. Several congestion control algorithms out in the market have no network support, and as such, they must act and rely only on the data provided by the servers they are running on. Some algorithms have used network's feedback to improve the performance, however these solutions depend on tuning parameters for marking queued packets, an arduous task on DCs shared by multiple applications with different workloads. In turn, Software-Defined Networking (SDN) created an opportunity to avoid congestion once the centralized controller can gather ongoing and historical information from all network switches and flows. The data gathered is enormous, and fast-computing algorithms are crucial for decision-making. In this sense, this work proposes Reinforcement Learning and SDN-aided Congestion Avoidance Tool (RSCAT), which uses data classification to determine if the network is congested and actor-critic reinforcement learning to find better Transmission Control Protocol (TCP) parameters. We evaluated RSCAT based on three workloads: a diversified DC traffic simulation; high-performance computing from NAS benchmark; and Hadoop TeraSort application. Our results show RSCAT fundamentals (reinforcement learning, data classification, and SDN) are potential candidates to achieve zero touch network congestion control. Specifically, our experimental analysis shows RSCAT, running alongside with traditional TCP congestion control algorithms like DCTCP and CUBIC, can decrease the flow completion time and applications' runtimes in several cases, without requiring any software update on DC end-points.
更多查看译文
关键词
00-01,99-00
AI 理解论文
溯源树
样例
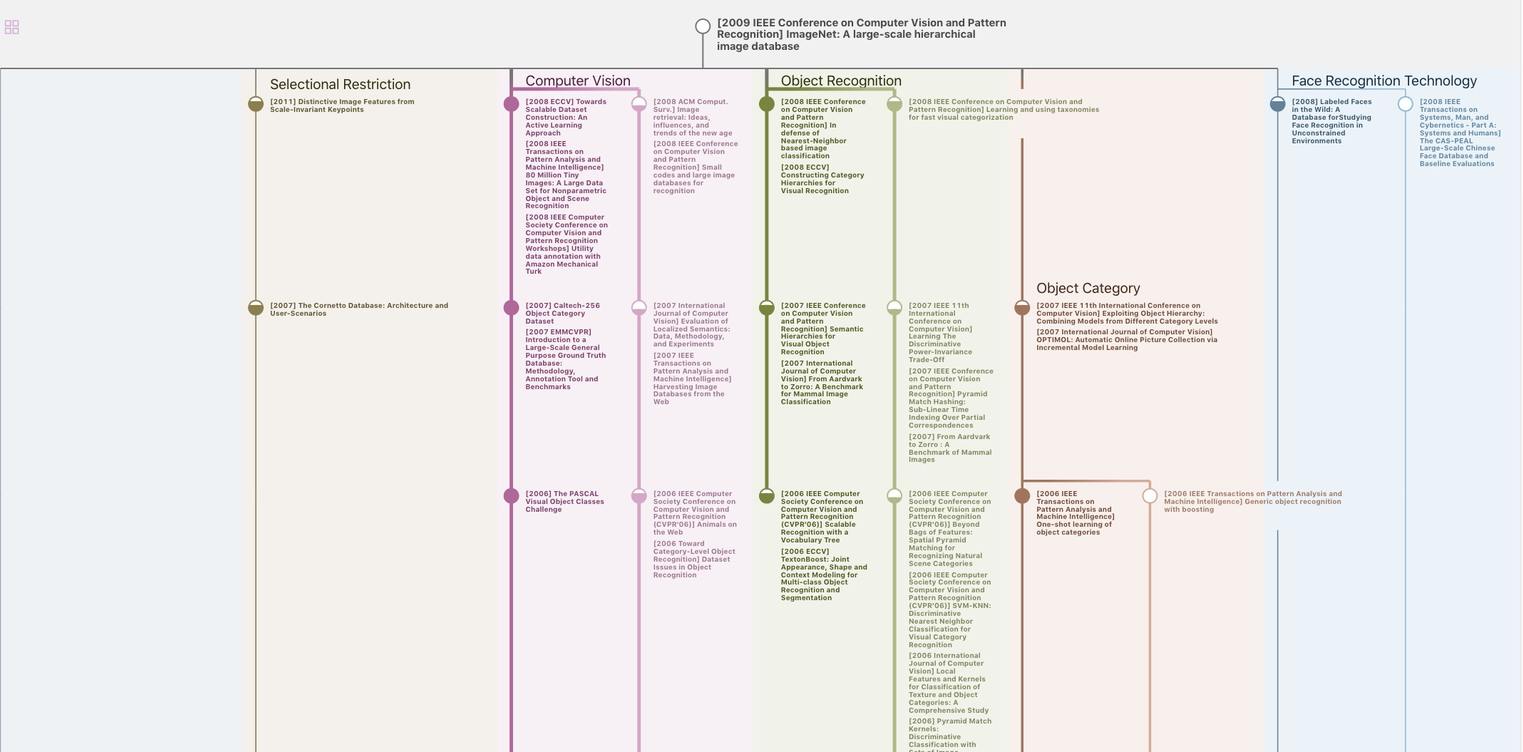
生成溯源树,研究论文发展脉络
Chat Paper
正在生成论文摘要