Deep learning-based framework for monitoring wearing personal protective equipment on construction sites.
J. Comput. Des. Eng.(2023)
摘要
The construction site is one of the most dangerous industries because the number of occupational injuries and fatalities is significantly higher compared to other industries. Proper use of personal protective equipment (PPE) by workers can reduce the risk of occupational injuries and fatalities. However, for a variety of reasons, workers tend not to wear their PPEs properly. To address these issues, we propose a vision-based framework for monitoring wearing PPE. The developed framework is based on the real-time pixel-level detect model YOLACT, which employs MobileNetV3 as a backbone to lightweight the proposed framework. In addition, the framework uses DeepSORT of object tracking algorithm to interpolate frames not predicted by the model. The post-processing algorithm in our framework classifies the correlation between workers and PPE into four statuses based on the results predicted by YOLACT and the interpolated results from DeepSORT. The results showed that the fine-tuned model achieved 66.4 mean average precision(50), and the algorithm successfully determined workers' PPE-wearing status detection with 91.3% accuracy. This study shows the potential to prevent occupational injuries and reduce social costs by automating monitoring at construction sites in real-time.
更多查看译文
关键词
construction safety, computer vision, deep learning, personal protective equipment
AI 理解论文
溯源树
样例
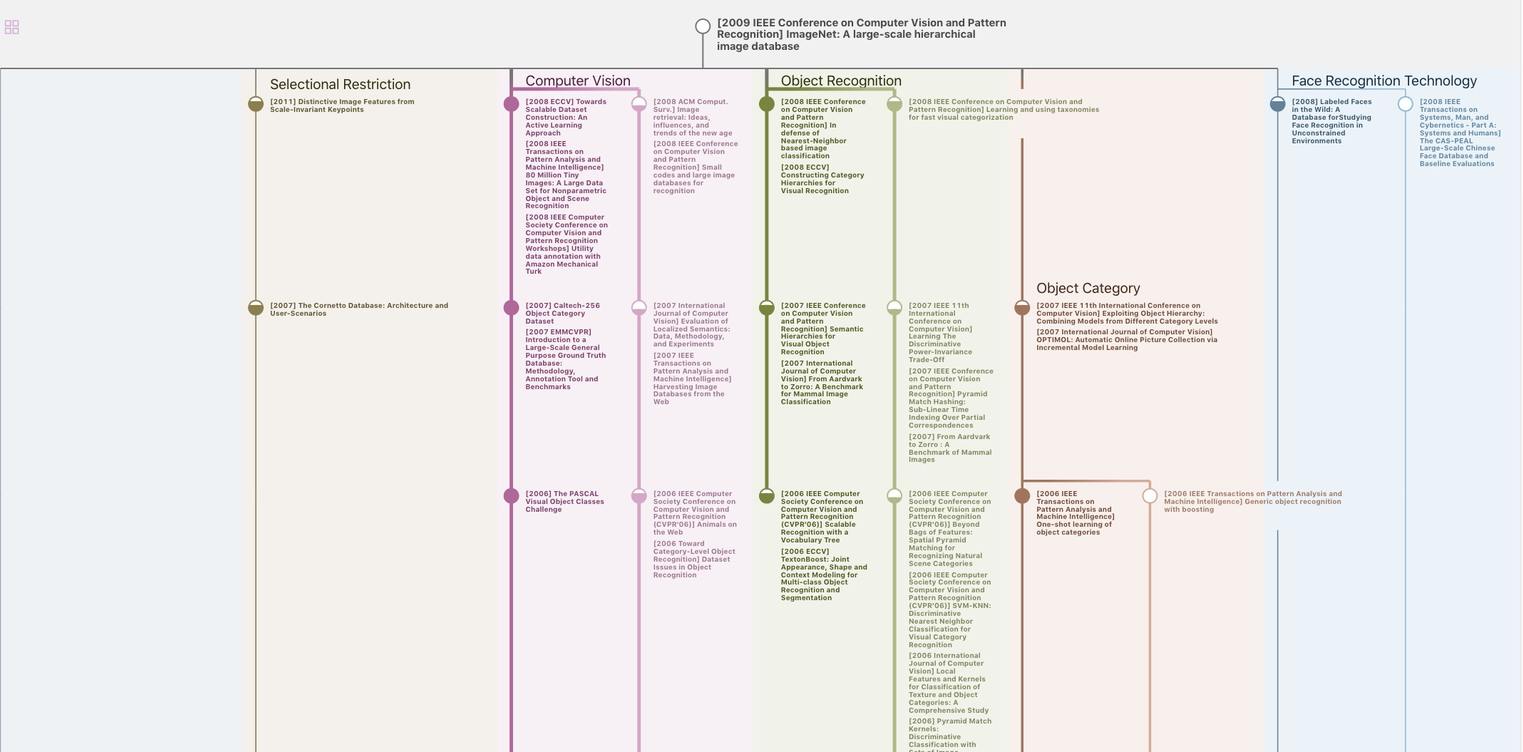
生成溯源树,研究论文发展脉络
Chat Paper
正在生成论文摘要