Voice Spoofing Detection Through Residual Network, Max Feature Map, and Depthwise Separable Convolution
IEEE Access(2023)
摘要
The goal of the "2019 Automatic Speaker Verification Spoofing and Countermeasures Challenge" (ASVspoof) was to make it easier to create systems that could identify voice spoofing attacks with high levels of accuracy. However, model complexity and latency requirements were not emphasized in the competition, despite the fact that they are stringent requirements for implementation in the real world. The majority of the top-performing solutions from the competition used an ensemble technique that merged numerous sophisticated deep learning models to maximize detection accuracy. Those approaches struggle with real-world deployment restrictions for voice assistants which would have restricted resources. We merged skip connection (from ResNet) and max feature map (from Light CNN) to create a compact system, and we tested its performance using the ASVspoof 2019 dataset. Our single model achieved a replay attack detection equal error rate (EER) of 0.30% on the evaluation set using an optimized constant Q transform (CQT) feature, outperforming the top ensemble system in the competition, which scored an EER of 0.39%. We experimented using depthwise separable convolutions (from MobileNet) to reduce model sizes; this resulted in an 84.3 percent reduction in parameter count (from 286K to 45K), while maintaining similar performance (EER of 0.36%). Additionally, we used Grad-CAM to clarify which spectrogram regions significantly contribute to the detection of fake data.
更多查看译文
关键词
Data models,Convolutional neural networks,Feature extraction,Deep learning,Training,Time-frequency analysis,Error analysis,Speech recognition,Voice assistant security,voice spoofing attack,voice synthesis attack,voice presentation attack detection
AI 理解论文
溯源树
样例
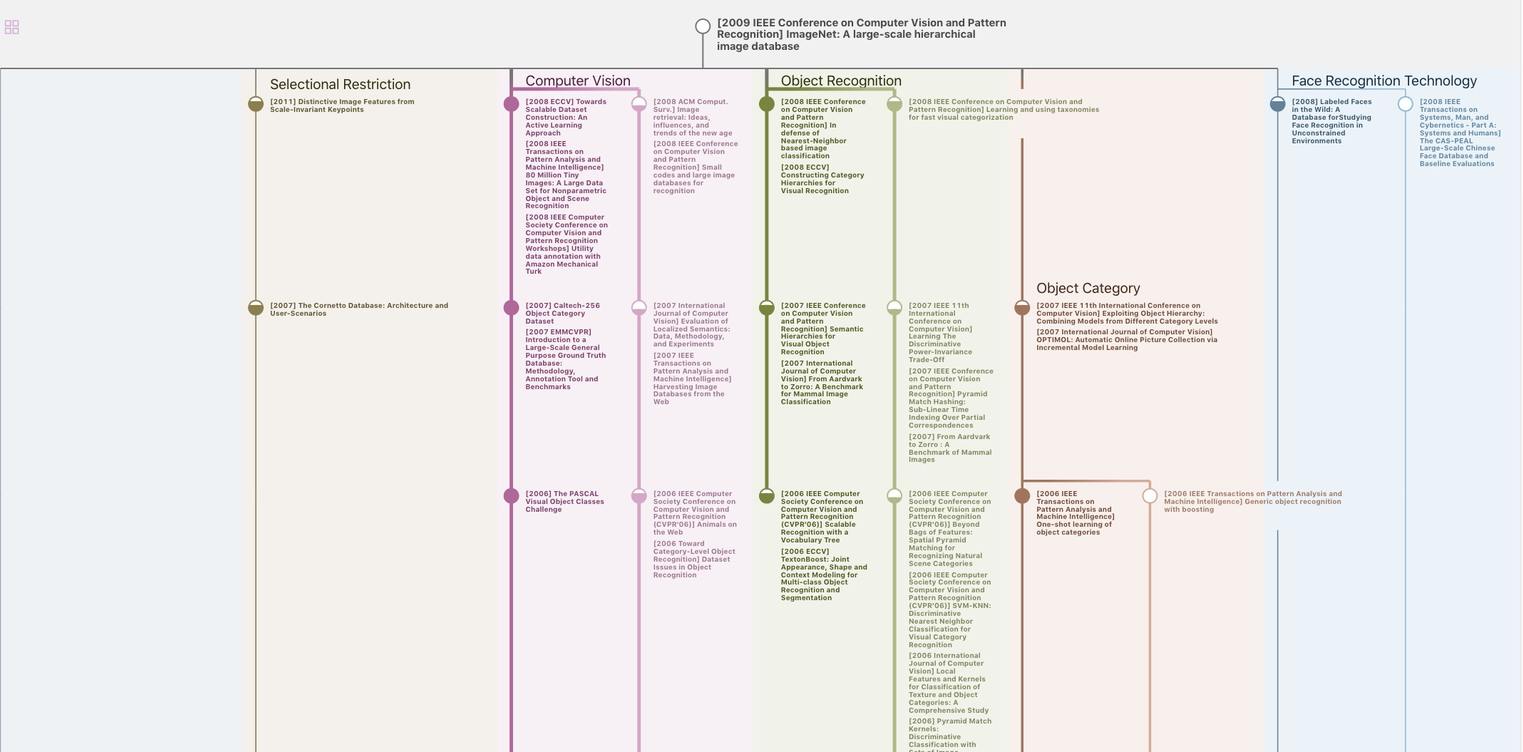
生成溯源树,研究论文发展脉络
Chat Paper
正在生成论文摘要