Research on Digital Twin Driven Rolling Bearing Model-Data Fusion Life Prediction Method
IEEE Access(2023)
摘要
In industry, accurate remaining useful life (RUL) prediction is critical in improving system reliability and reducing downtime and accident risk. Numerous data-driven RUL prediction approaches have been proposed and achieved impressive performance in RUL prediction. However, most of them are still faced with the dilemma of limited samples, and most of popular transfer learning and domain adaptive methods adopt single-source domain adaptation (DA), ignoring the domain-shift within source domain and failing to fully utilize the multi-condition data. This article proposes a model-data fusion life prediction method based on digital twin (DT) and multi-source regression adversarial domain adaptation (MRADA) to address the aforementioned issues. For data-driven life prediction model, the model-based DT technology among them offers a significant amount of multi-condition training data. The proposed MRADA fully utilizes the benefits of DT simulation data by using intra-group alignment strategy, inter-group alignment strategy, adversarial learning, and regressor alignment strategy to learn domain-invariant features and supervision from multiple sources. The experimental findings demonstrate that the proposed fusion life prediction method can successfully address the issue of small samples and improve the accuracy of rolling bearing life prediction results.
更多查看译文
关键词
Digital twin,domain adaptation,remaining useful life prediction,small sample size
AI 理解论文
溯源树
样例
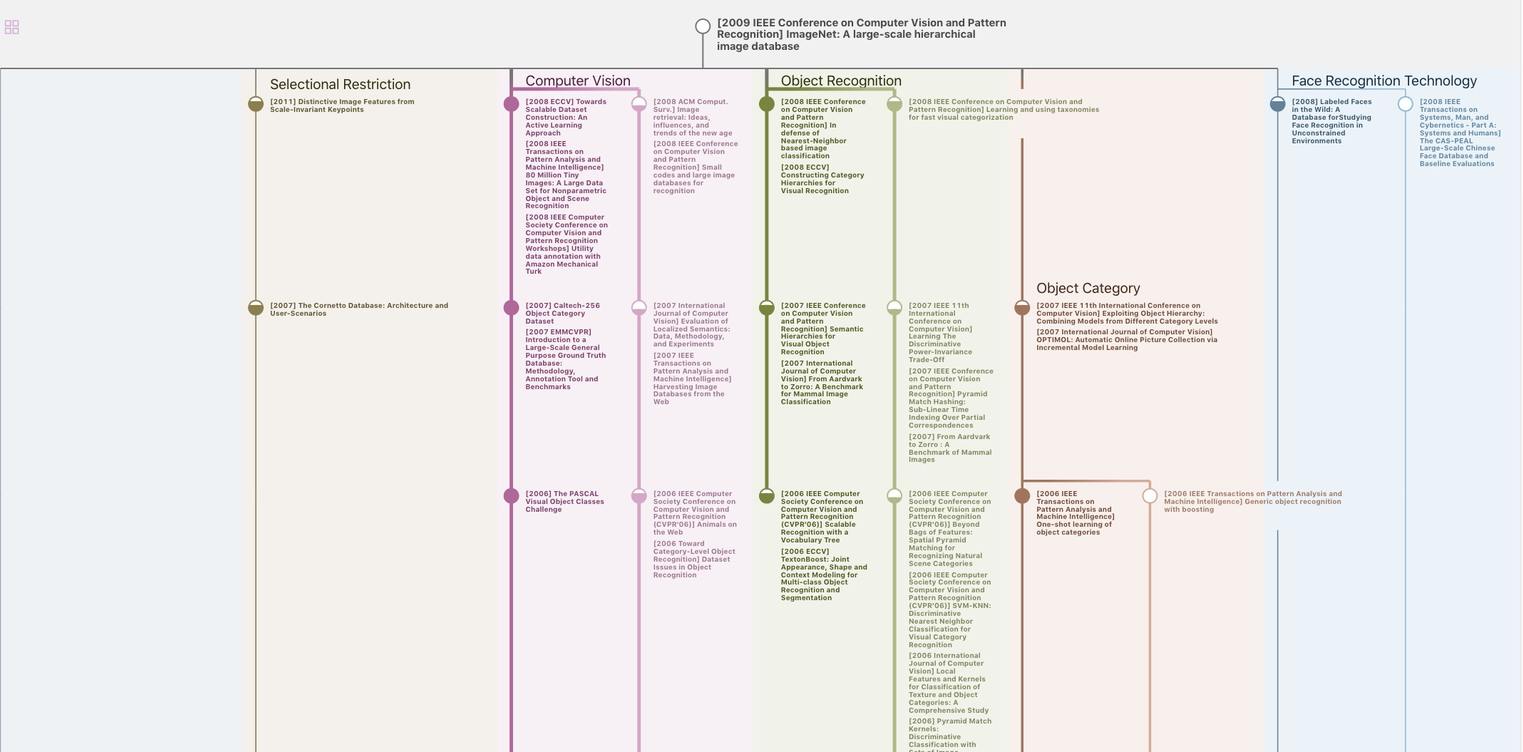
生成溯源树,研究论文发展脉络
Chat Paper
正在生成论文摘要