Automatic Sleep Stage Classification Using Deep Learning Algorithm for Multi-Institutional Database.
IEEE Access(2023)
摘要
Recent deep learning studies for sleep stage classification with polysomnography (PSG) data show two directions, either using 1-dimensional (1-D) raw PSG data or spectrogram images time-frequency domain. We propose a novel approach using images generated from time-signal display of a PSG dataset for 5 class sleep stage classification. The motivation of our approach is not only to imitate the way used by human sleep-scoring experts but also to make use of various methods developed in image classification in Deep Learning, such as augmentation techniques, EfficientNet and LSTM. In addition an explainable AI technique such as Class Activation Map (CAM) can be employed for interpreting how a model makes a decision. We, also, work on "inconsistency" problems occurring among multiple institutions/hospitals where different capturing sensors are used and the labelling mismatch by human experts in different organizations. To solve the problem, we experiment three different approaches in the network design with data of two institutes and 5 sleep stage classification; (i) 5 class classification, (ii) 10-class classification and then post-processing to 5 classes (iii) 10-to-5 class classification. The 10-to-5 class classification is a network where information of two institutes are embedded inside the network. When information of multi institution is inside the network, the results show higher performance. Our experimental results show that all of three proposed methods based on time-signal images achieves higher accuracy performance compared to state-of-the-art models.
更多查看译文
关键词
Brain modeling, Sleep, Deep learning, Data models, Electroencephalography, Spectrogram, Band-pass filters, image classification, sleep, INDEX TERMS, rapid eye movement sleep
AI 理解论文
溯源树
样例
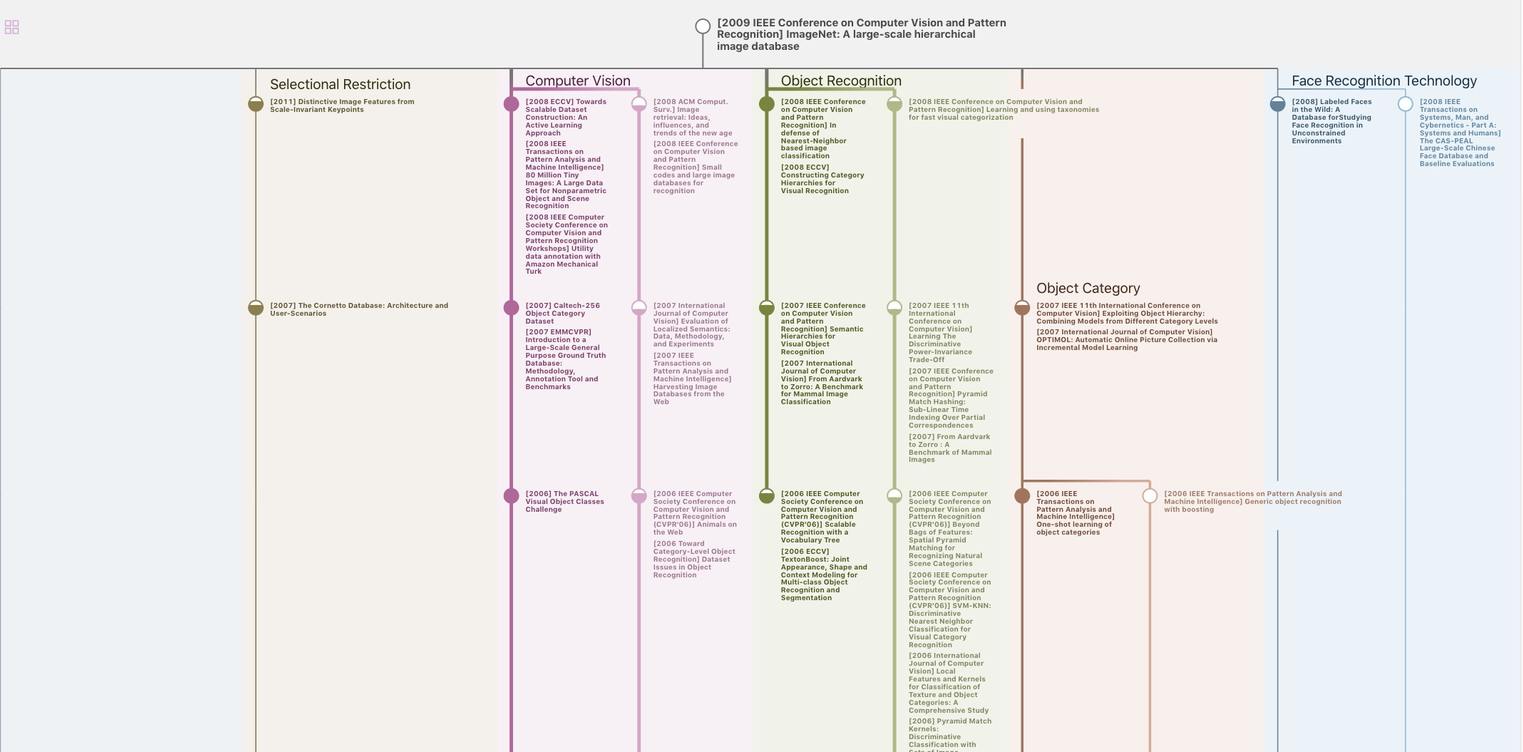
生成溯源树,研究论文发展脉络
Chat Paper
正在生成论文摘要