Less Parameterization Inception-Based End to End CNN Model for EEG Seizure Detection
IEEE Access(2023)
摘要
Many deep-learning-based seizure detection algorithms have achieved good classification, which usually outperformed traditional machine-learning-based algorithms. However, the hand-engineered features increase the computational complexity and potentially have an ineffectiveness problem for the category. Therefore, this paper proposes a novel end-to-end deep-learning model comprising an inception module and a residual module to analyze the multi-scales of original EEG signals and realize seizure detection without feature extraction. Experiments were conducted and evaluated on the Bonn dataset and the CHB-MIT dataset. In the subject-dependent experiments, our model achieved an average F1-score of 69.34% on the CHB-MIT dataset. In subject-independent experiments, our method achieved an average accuracy of 99.04% on the Bonn dataset and an average F1-score of 37.31% on the CHB-MIT dataset. A series of analyses confirmed that our proposed model has better classification performance and lower computational complexity than existing end-to-end seizure detection models.
更多查看译文
关键词
Convolutional neural networks,seizure detection,epilepsy,electroencephalography,end-to-end model
AI 理解论文
溯源树
样例
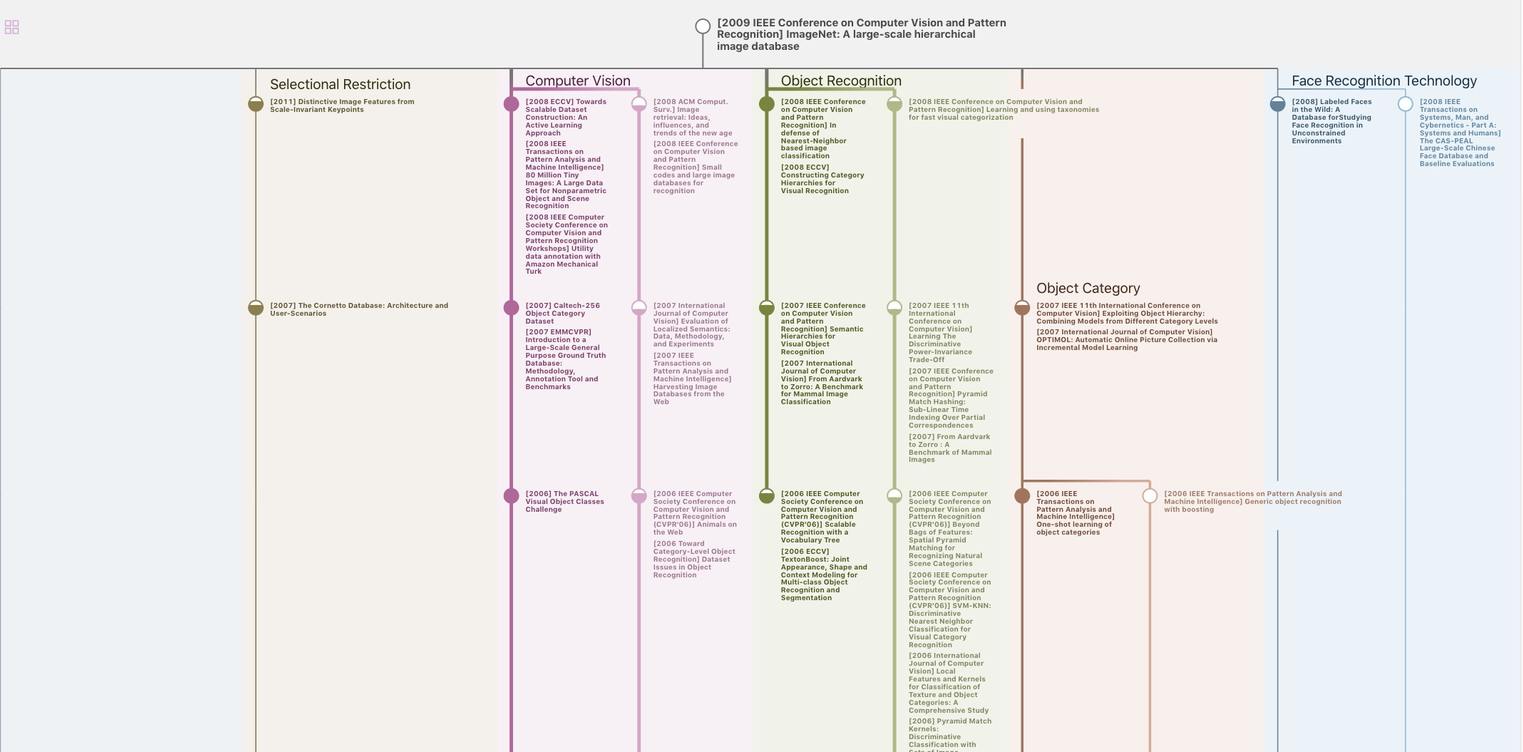
生成溯源树,研究论文发展脉络
Chat Paper
正在生成论文摘要