Same-Subject-Modalities-Interactions: A Novel Framework for MRI and PET Multi-Modality Fusion for Alzheimer's Disease Classification
IEEE Access(2023)
摘要
Alzheimer's disease is a growing concern, and neuroimaging techniques such as Magnetic Resonance Imaging (MRI) and Positron Emission Tomography (PET) scans are widely used to classify AD patients. While MRI captures structural information and measures brain atrophy, PET shows functional changes associated with neurological disorders, and both modalities have been proven to be AD biomarkers. However, combining MRI and PET in the same test without considering their inherent structural differences can result in a loss of important information. To address this issue, this paper proposes a novel machine learning framework for combining MRI and PET modalities and a new set of interactions known as Same-Subject-Modalities-Interactions (SSMI) to extract complementary information and new insights. The SSMI relation is derived from MRI and PET and subjected to PCA to construct the SSMI set, which is then concatenated with the other sets. The best set of features is selected and used for classification using Ridg-Classifier. Freesurfer is used to extract measures from 183 ADNI subjects (69 in the AD group and 114 in the CN group), and different classifiers are performed with train-test-split, cross-validation, and validation-set from ADNI-2/GO. The results showed high accuracy, precision, specificity, recall, F1-score, and AUC, with values of 98.94%, 98.27%, 97.10%, 100%, 99.13 and 98.55%, respectively, from ADNI and 98.75%, 98.48%, 93.75%, 100.0%, 99.23%, 96.80% from ADNI2/Go. These results are higher than those achieved by single-modality classification tasks and state-of-the-art approaches. Furthermore, the regions selected by Ridge Classifier are shown to be highly related to Alzheimer's disease biomarkers.
更多查看译文
关键词
Multimodality,MRI,PET,fusion,machine learning,feature selection
AI 理解论文
溯源树
样例
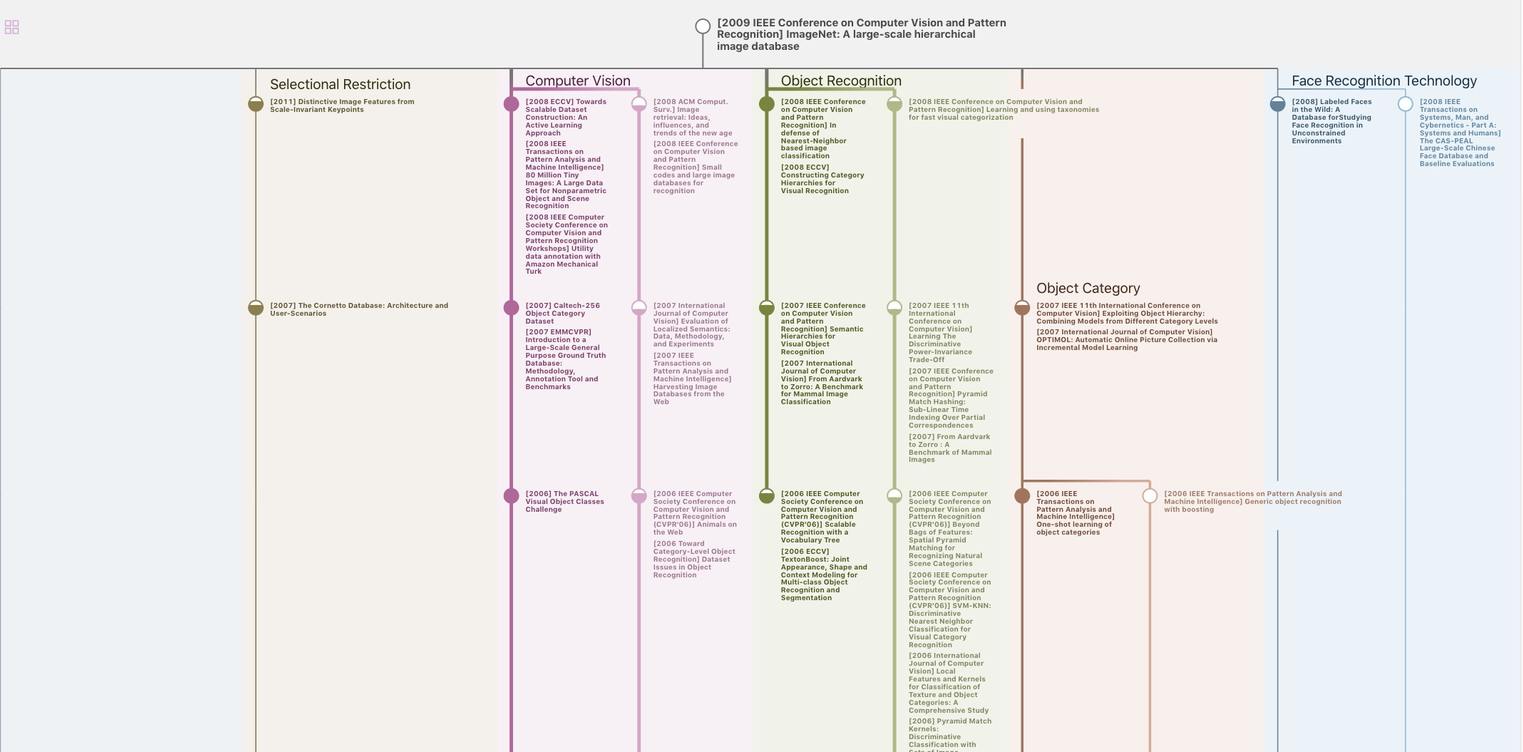
生成溯源树,研究论文发展脉络
Chat Paper
正在生成论文摘要