Multi2Claim: Generating Scientific Claims from Multi-Choice Questions for Scientific Fact-Checking
17TH CONFERENCE OF THE EUROPEAN CHAPTER OF THE ASSOCIATION FOR COMPUTATIONAL LINGUISTICS, EACL 2023(2023)
摘要
In automated scientific fact-checking, machine learning models are trained to verify scientific claims given evidence. A major bottleneck of this task is the availability of large-scale training datasets on different domains, due to the required domain expertise for data annotation. However, multiple-choice question-answering datasets are readily available across many different domains, thanks to the modern online education and assessment systems. As one of the first steps towards addressing the fact-checking dataset scarcity problem in scientific domains, we propose a pipeline for automatically converting multiple-choice questions into factchecking data, which we call Multi2Claim. By applying the proposed pipeline, we generated two large-scale datasets for scientificfact-checking: Med-Fact and Gsci-Fact for the medical and general science domains, respectively. These two datasets are among the first examples of large-scale scientific-factchecking datasets. We developed baseline models for the verdict prediction task using each dataset. Additionally, we demonstrated that the datasets could be used to improve performance measured by weighted F1 on existing fact-checking datasets such as SciFact, HEALTHVER, COVID-Fact, and CLIMATEFEVER. In some cases, the improvement in performance was up to a 26% increase. The generated datasets are publicly available(1).
更多查看译文
AI 理解论文
溯源树
样例
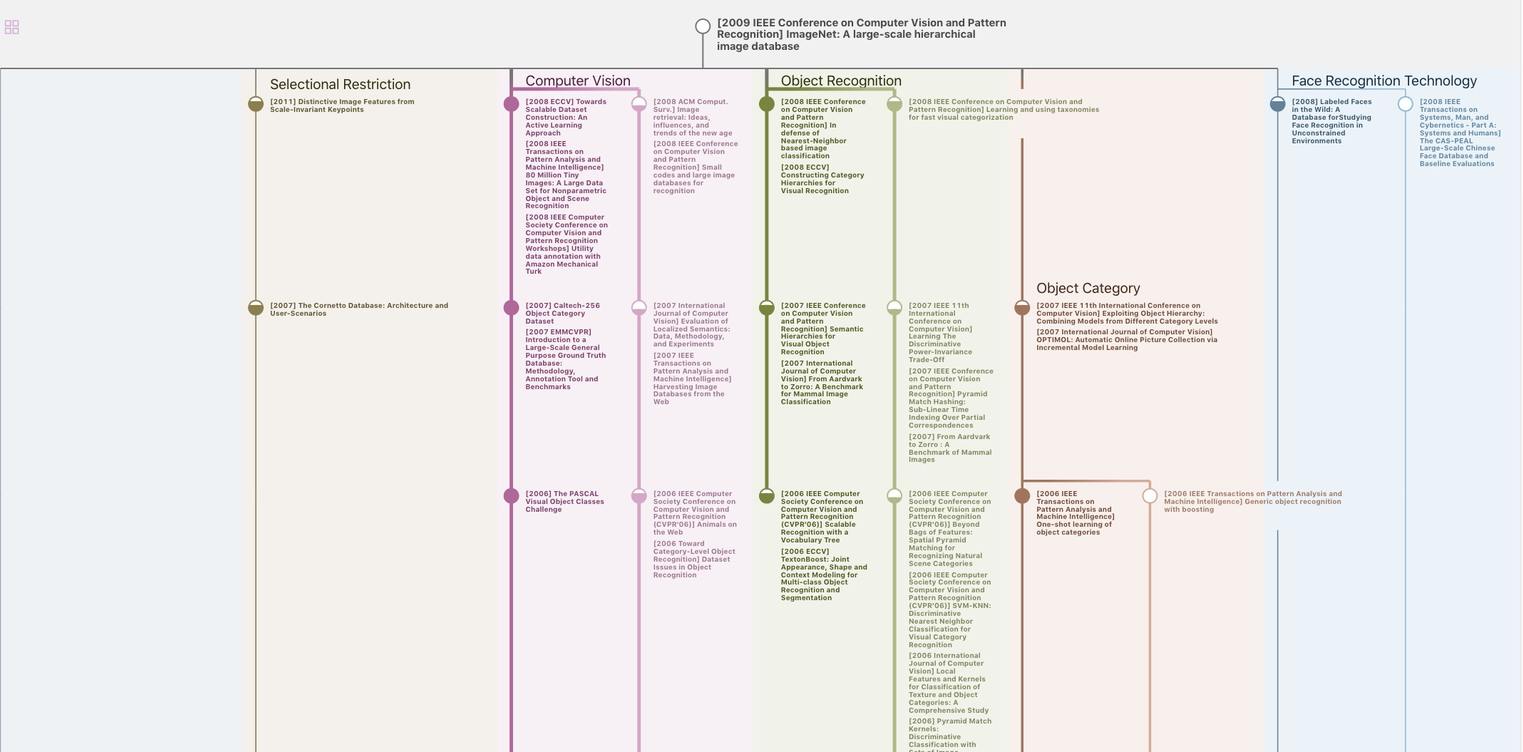
生成溯源树,研究论文发展脉络
Chat Paper
正在生成论文摘要