Uncovering Implicit Inferences for Improved Relational Argument Mining
17TH CONFERENCE OF THE EUROPEAN CHAPTER OF THE ASSOCIATION FOR COMPUTATIONAL LINGUISTICS, EACL 2023(2023)
摘要
Argument mining seeks to extract arguments and their structure from unstructured texts. Identifying relations (such as attack, support, and neutral) between argumentative units is a challenging task because two units may be related to each other via implicit inferences. These inferences often rely on external commonsense knowledge to discover how one argumentative unit relates to another. State-of-the-art methods, however, rely on predefined knowledge graphs, and thus might not cover target pairs of argumentative units well. We introduce a new generative approach to finding inference chains that connect these pairs by making use of the Commonsense Transformer (COMET). We evaluate our approach on three datasets for both the two-label (attack/support) and three-label (attack/support/neutral) tasks. Our approach significantly outperforms the state-of-the-art, by 25% in F1 score, on two out of the three datasets with minor improvements on the remaining one.
更多查看译文
AI 理解论文
溯源树
样例
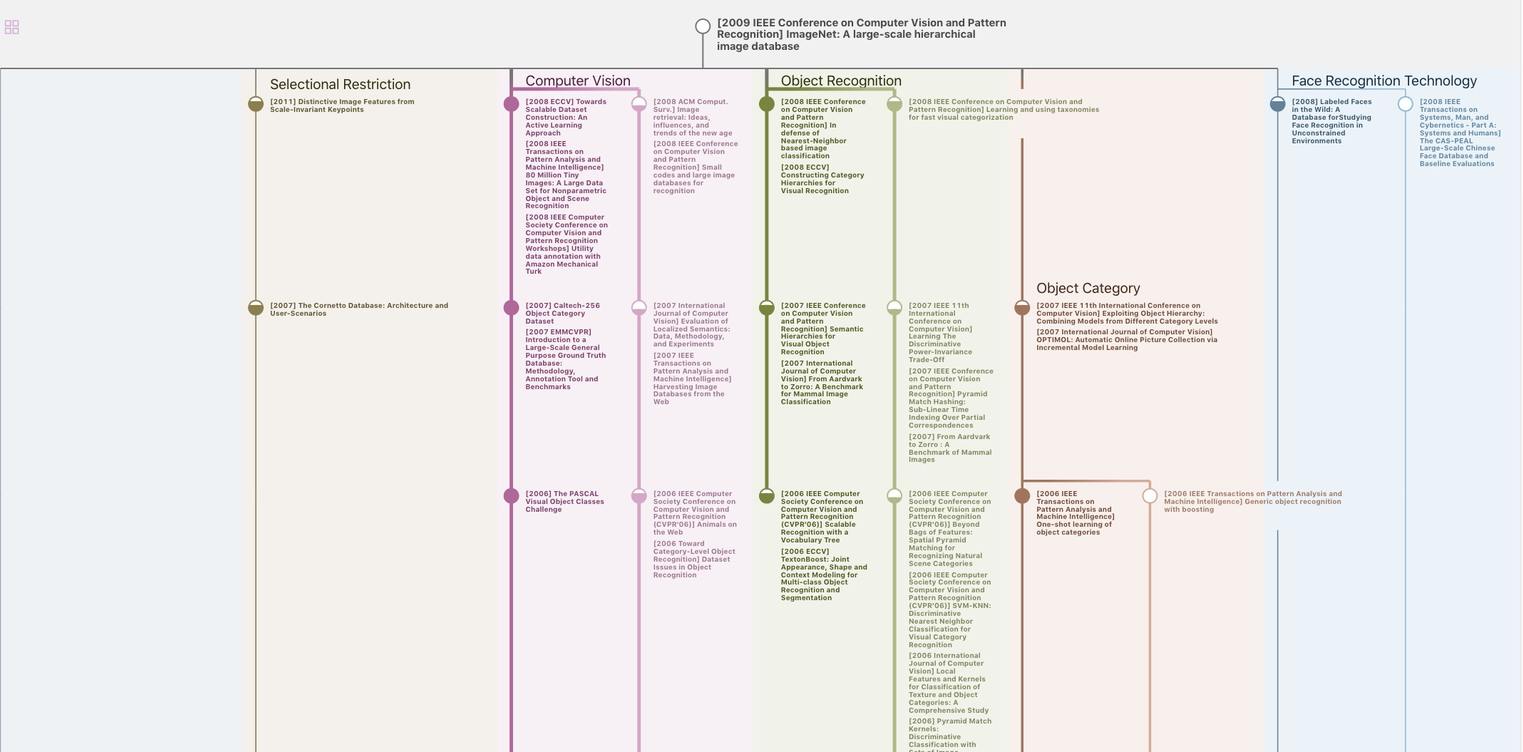
生成溯源树,研究论文发展脉络
Chat Paper
正在生成论文摘要