TwiRGCN: Temporally Weighted Graph Convolution for Question Answering over Temporal Knowledge Graphs.
EACL(2023)
Abstract
Recent years have witnessed much interest in temporal reasoning over knowledge graphs (KG) for complex question answering (QA), but there remains a substantial gap in human capabilities. We explore how to generalize relational graph convolutional networks (RGCN) for temporal KGQA. Specifically, we propose a novel, intuitive and interpretable scheme to modulate the messages passed through a KG edge during convolution, based on the relevance of its associated time period to the question. We also introduce a gating device to predict if the answer to a complex temporal question is likely to be a KG entity or time and use this prediction to guide our scoring mechanism. We evaluate the resulting system, which we call TwiRGCN, on TimeQuestions, a recently released, challenging dataset for multi-hop complex temporal QA. We show that TwiRGCN significantly outperforms state-of-the-art systems on this dataset across diverse question types. Notably, TwiRGCN improves accuracy by 9--10 percentage points for the most difficult ordinal and implicit question types.
MoreTranslated text
Key words
question answering
AI Read Science
Must-Reading Tree
Example
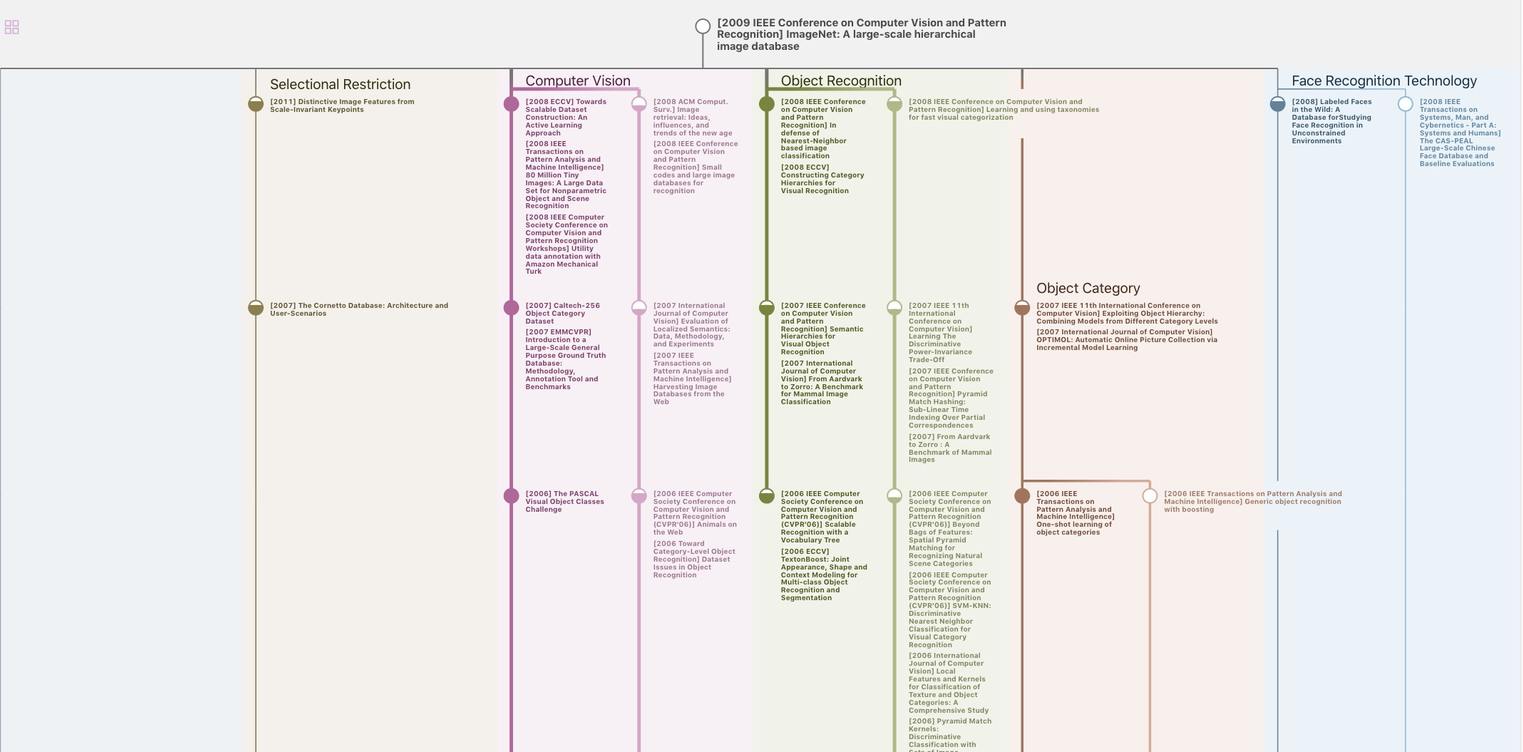
Generate MRT to find the research sequence of this paper
Chat Paper
Summary is being generated by the instructions you defined