Exploring the Classification Performance of Different EEG Bands for Anesthesia Monitoring
Iran Journal of Computer Science(2022)
摘要
The use of anesthesia during major surgery is unconditionally obligatory, although the intraoperative and postoperative complications of anesthesia turned out to be common too. It is because of the lack of choosing the optimal dose of anesthesia or, in other words, not being able to detect the depth of anesthesia. Therefore, correct prediction of the patient's deep-sleep state or the transitional state from deep sleep to awake could help to attain the optimal dose of anesthesia that removes unnecessary use of anesthetic agents. In this work, we developed a machine-learning model that can predict the deep-sleep state based on different bands of EEG signals when the patient is under anesthesia. In this work, an open-source database containing the EEG data of anesthetic patients during surgery was collected. The data were separated into three states: into the deep-sleep state (IntoDeep), the deep-sleep state (InDeep), and the awake state (InAwake). The raw EEG signals were filtered and their standard bands (alpha, beta, delta, theta, and gamma) were separated and their signal powers were calculated. These band-power values are considered the features of the signal. An artificial neural network model was trained with the features and validated. Finally, the model was tested with the testing samples and found almost similar classification accuracy for all bands with slight variations. The final results conclude that the most potential bands are beta and delta for the deep-sleep state classification from EEG signal in the case of anesthetic patient monitoring.
更多查看译文
关键词
Depth of anesthesia,EEG band,Deep sleep,Artificial neural network (ANN),Anesthesia monitoring
AI 理解论文
溯源树
样例
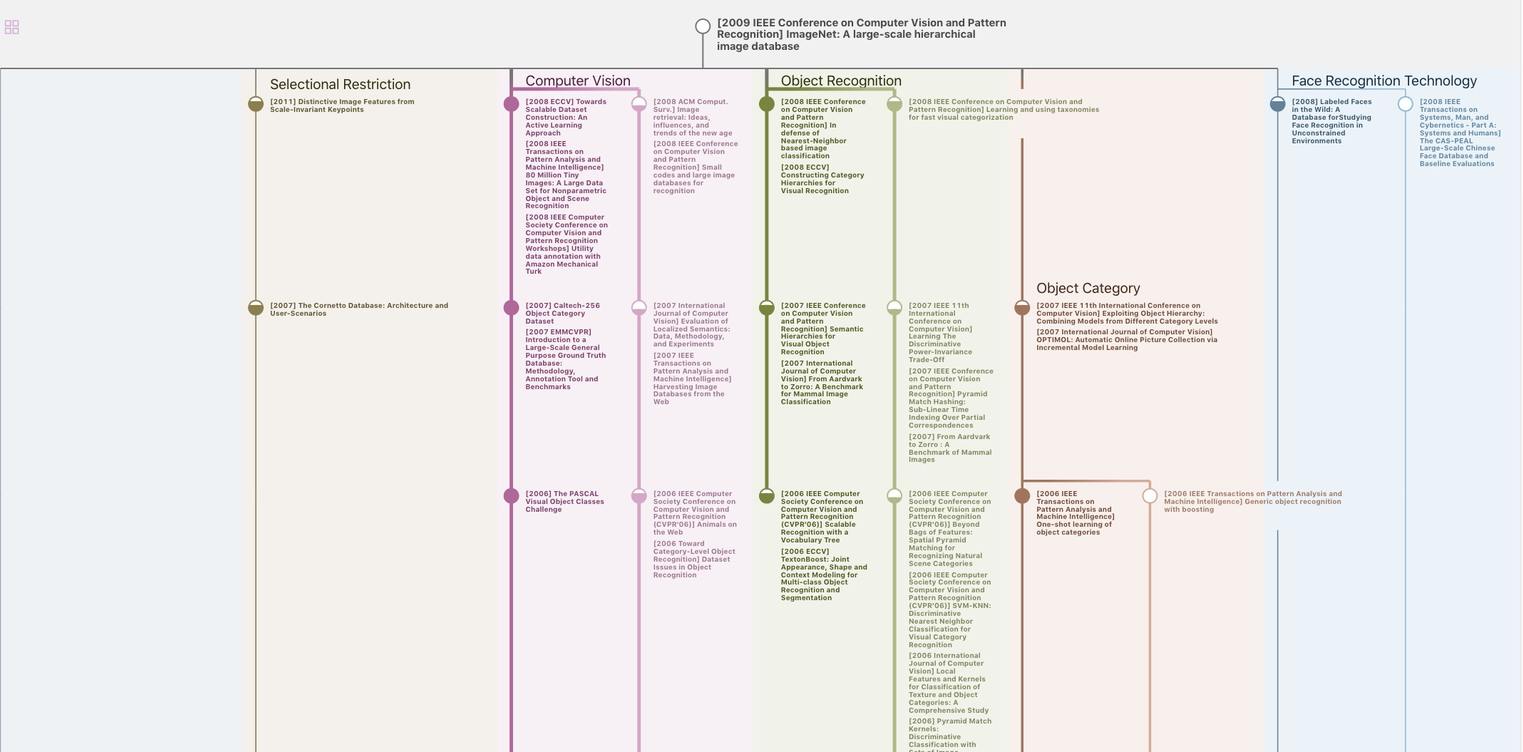
生成溯源树,研究论文发展脉络
Chat Paper
正在生成论文摘要