Dynamic Pilot Design for Multicast in the Internet of Vehicles Running at Different Speeds
IEEE INTERNET OF THINGS JOURNAL(2023)
Abstract
High mobility of vehicles causes time-frequency selective fading over physical channels within the Internet of Vehicles (IoV). To improve the resource utilization efficiency, a novel transmission strategy, based on dynamic pilot design, is proposed, in this article, to reduce the pilot consumption in doubly selective channel estimation for the multicast to vehicles running at different speeds. As the channel coherence time is mainly influenced by the receiver mobility in the multicast from a base station to vehicles, we define a multicast block as the channel coherence time of the slowest vehicle in the multicast group, where common pilot symbols are shared. Then, the multicast data destined for different vehicles are loaded into the block according to their own channel coherence times. To evaluate the performance and resource utilization of our dynamic pilot design, the metrics of overhead rate, spectral efficiency, and energy efficiency are formulated for the IoV multicast using multiple-input–multiple-output (MIMO) orthogonal frequency-division multiplexing (OFDM) transmissions. In terms of these three metrics, illustrative numerical results on the comparisons between our dynamic pilot design and the conventional counterpart are provided, which not only substantiate that the former outperforms the latter but also present useful tools and specifications for the pilot design in the IoV multicast using MIMO–OFDM transmissions over doubly selective channels.
MoreTranslated text
Key words
Channel estimation,Symbols,Vehicle dynamics,Resource management,MIMO communication,OFDM,Dynamic scheduling,Doubly selective channel estimation,dynamic pilot design,energy efficiency,Internet of Vehicles (IoV),overhead rate,spectral efficiency
求助PDF
上传PDF
View via Publisher
AI Read Science
AI Summary
AI Summary is the key point extracted automatically understanding the full text of the paper, including the background, methods, results, conclusions, icons and other key content, so that you can get the outline of the paper at a glance.
Example
Background
Key content
Introduction
Methods
Results
Related work
Fund
Key content
- Pretraining has recently greatly promoted the development of natural language processing (NLP)
- We show that M6 outperforms the baselines in multimodal downstream tasks, and the large M6 with 10 parameters can reach a better performance
- We propose a method called M6 that is able to process information of multiple modalities and perform both single-modal and cross-modal understanding and generation
- The model is scaled to large model with 10 billion parameters with sophisticated deployment, and the 10 -parameter M6-large is the largest pretrained model in Chinese
- Experimental results show that our proposed M6 outperforms the baseline in a number of downstream tasks concerning both single modality and multiple modalities We will continue the pretraining of extremely large models by increasing data to explore the limit of its performance
Upload PDF to Generate Summary
Must-Reading Tree
Example
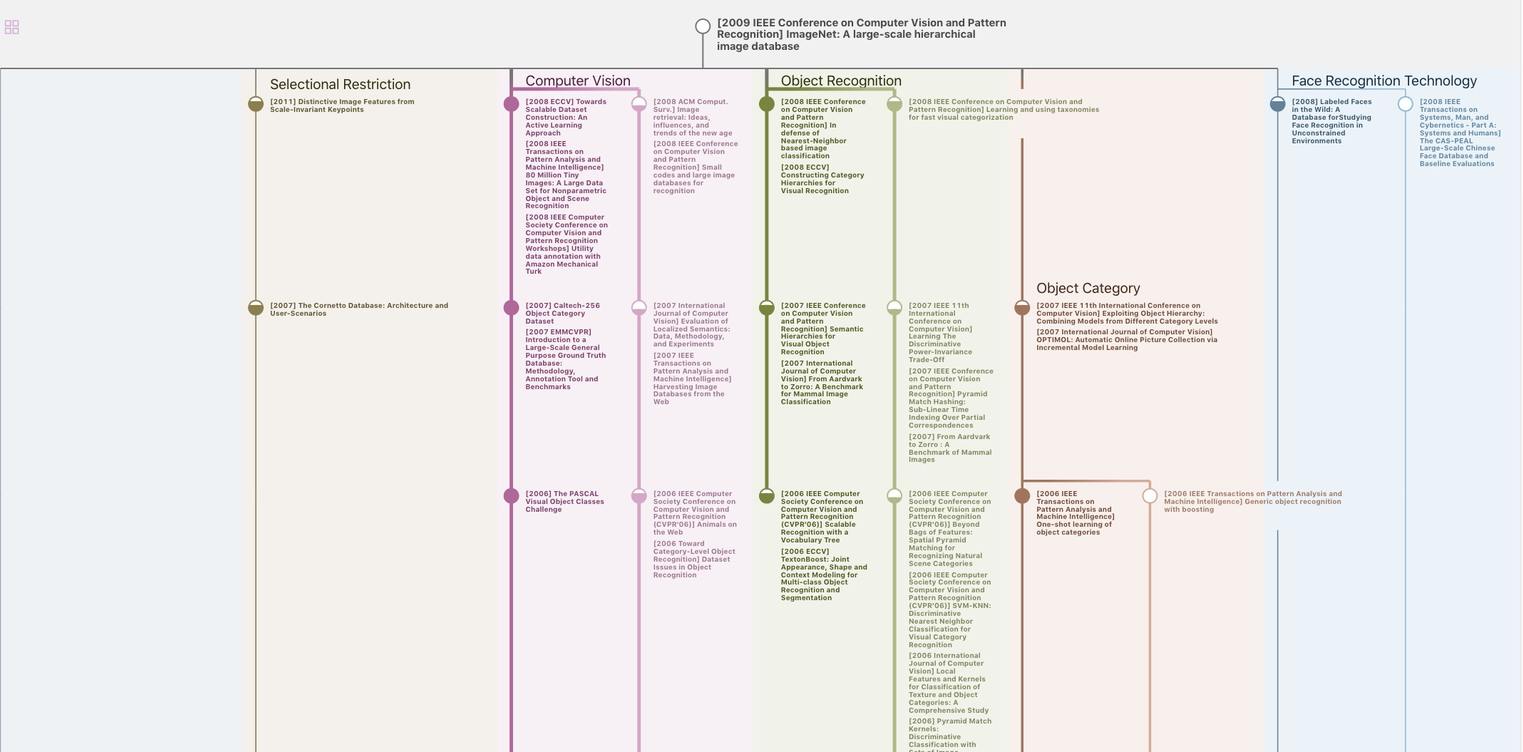
Generate MRT to find the research sequence of this paper
Related Papers
2011
被引用53 | 浏览
2013
被引用9 | 浏览
2013
被引用6 | 浏览
2011
被引用2 | 浏览
2013
被引用15 | 浏览
2014
被引用5 | 浏览
1991
被引用1289 | 浏览
2012
被引用69 | 浏览
2016
被引用37 | 浏览
2018
被引用19 | 浏览
2020
被引用35 | 浏览
2020
被引用23 | 浏览
Data Disclaimer
The page data are from open Internet sources, cooperative publishers and automatic analysis results through AI technology. We do not make any commitments and guarantees for the validity, accuracy, correctness, reliability, completeness and timeliness of the page data. If you have any questions, please contact us by email: report@aminer.cn
Chat Paper