MuAt-Va: Multi-Attention and Video-Auxiliary Network for Device-Free Action Recognition
IEEE Internet of Things Journal(2023)
摘要
With the growing popularity of Internet of Things (IoT) systems, device-free action recognition begins to attract extensive attention due to its friendly feasibility in broad applications, such as human–computer interaction and smart elderly care. Considering abundant information in the vision modality, existing methods adopt the cross-model methods for performance enhancement. However, the dependency of synchronous multimodal data in the collection and recognition stage brings into the vision weaknesses, such as sensitivity to occlusion and privacy invasion. In this article, we integrate multi-attention structure and auxiliary video information into a novel end-to-end deep learning framework named MuAt-Va, in which video soft labels learned in advance are utilized to teach the multi-attention WiFi feature training process without vision information involved during the test. Specifically, in order to enlarge the application scope and reduce the data cost, we beforehand acquire videos under a satisfactory condition only once, and then leverage teacher–student mechanism to guide the WiFi stream. Instead of straightforwardly concatenating multiantenna channel state information (CSI) from homogeneous wireless signals as previous works, we design a CSI subcarrier-wise, temporal-wise, and view-wise attention module to assign different weights on the basis of data characteristics for the sensing task. Our experiments with multiple subjects data in two scenes demonstrate that MuAt-Va can accurately recognize human actions with more superior performances.
更多查看译文
关键词
Cross-modal learning,device-free action recognition,multi-attention,video-auxiliary
AI 理解论文
溯源树
样例
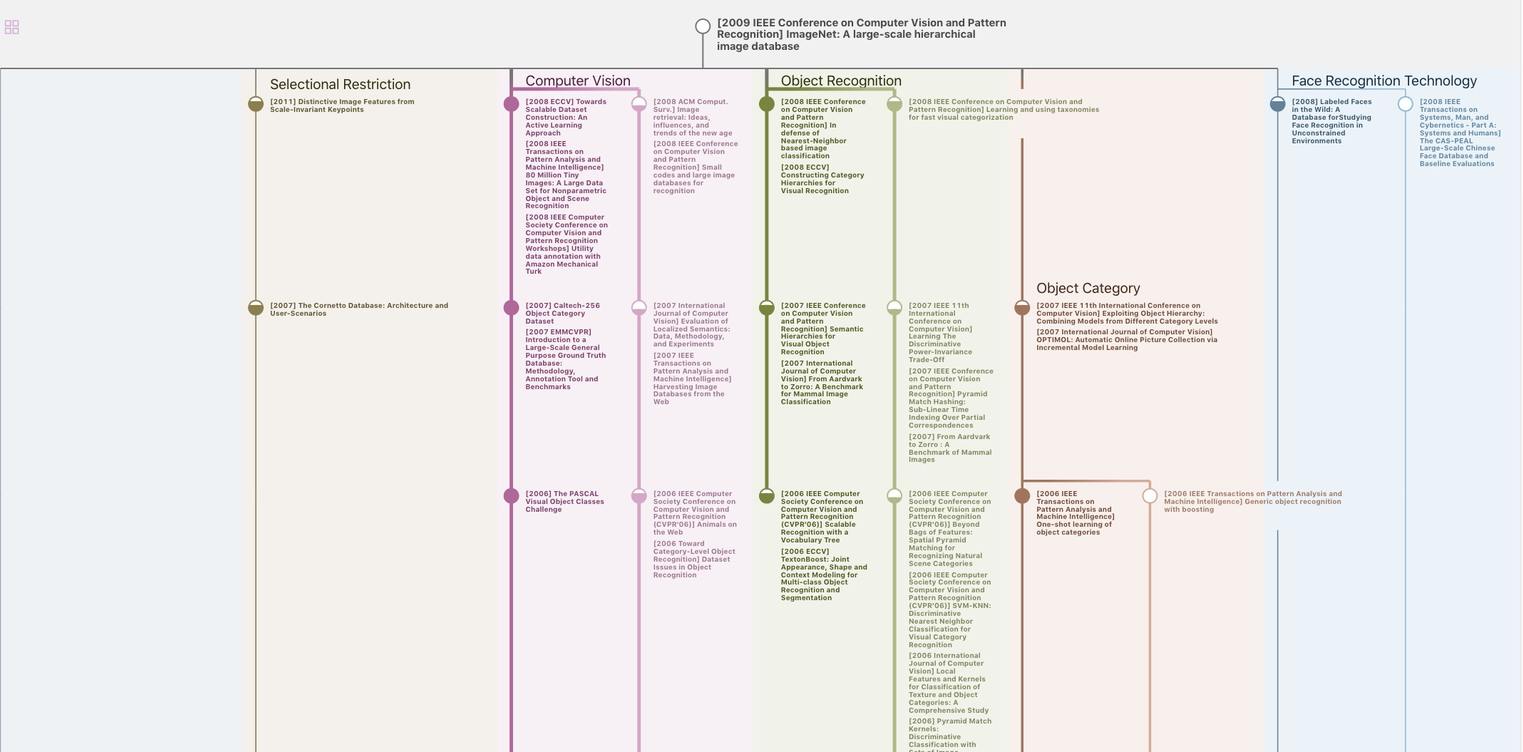
生成溯源树,研究论文发展脉络
Chat Paper
正在生成论文摘要