A Multi-Task Learning Method Integrating Multi-Information Fusion for Non-Intrusive Load Monitoring
2022 6th Asian Conference on Artificial Intelligence Technology (ACAIT)(2022)
摘要
The aim of non-intrusive load monitoring (NILM) is to determine the energy consumption of each electric load within a range by analyzing the total electricity meter data for a particular area. Since existing methods don’t consider the interactions between various loads, it is difficult to fully explore load features from the limited total meter data. To address this problem, NILM model is proposed based on multi-information fusion and multi-task learning (MIFMTL-NILM). Specifically, 1) Enhancing the features of samples by extracting the load features inherent in the total electricity meter data of various windows. 2) Enhancing the accuracy of load disaggregation and reducing the resource overhead of edge devices, multi-task learning is used to share network parameters across various electrical loads. Finally, the performance and effectiveness of the proposed model are validated using the public dataset UK-DALE and the constructed dataset in the laboratory.
更多查看译文
关键词
Non-intrusive load monitoring (NILM),multi-information fusion,multi-task learning
AI 理解论文
溯源树
样例
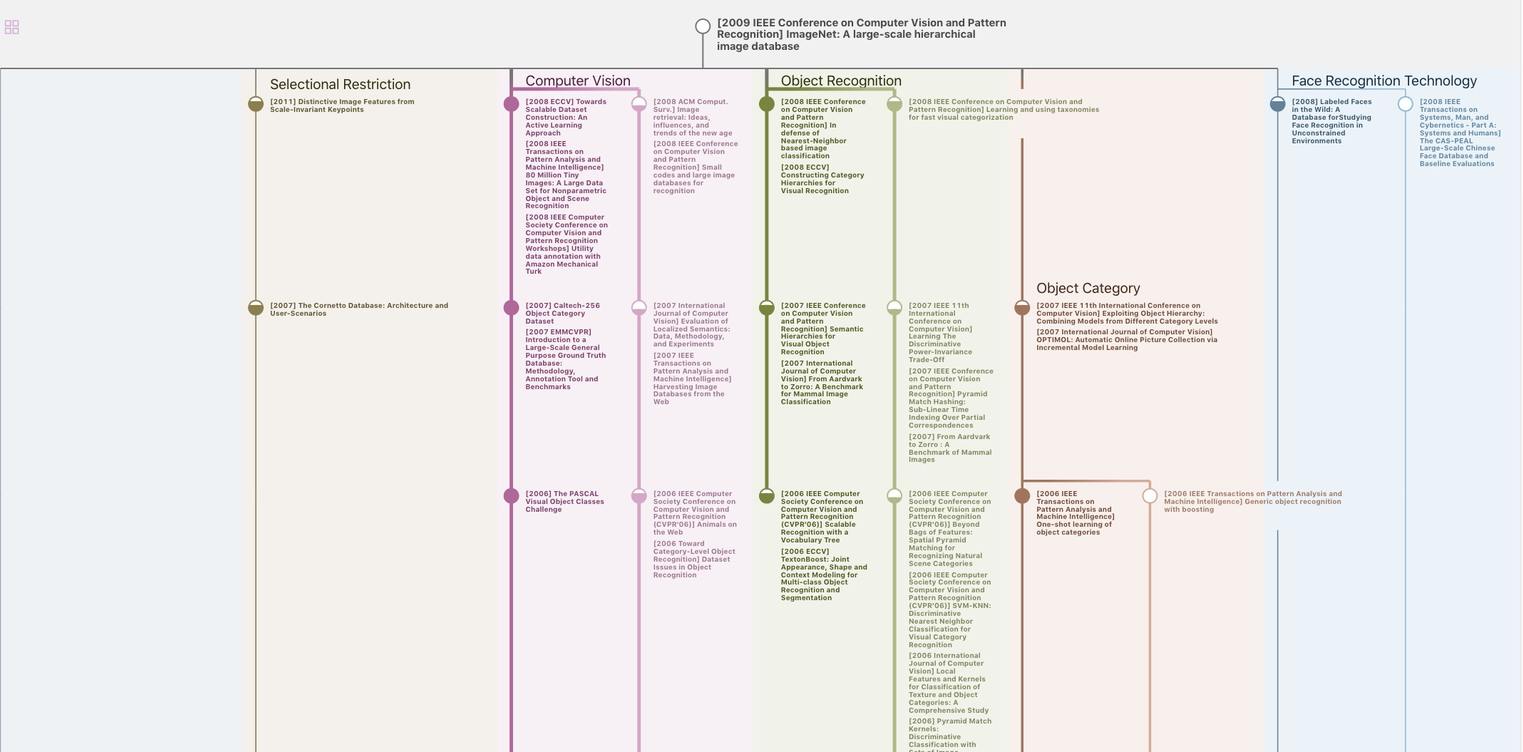
生成溯源树,研究论文发展脉络
Chat Paper
正在生成论文摘要