Machine Learning Aided NR-V2X Quality of Service Predictions
2023 IEEE Vehicular Networking Conference (VNC)(2023)
摘要
Vehicle-to-Everything Communication (V2X) technologies aim to meet strict quality-of-service (QoS) requirements of vehicular connectivity applications such as safety message exchange, remote driving, and sensor data sharing. The high reliability requirement is particularly important to enable safety relevant applications. Thus, predicting QoS levels becomes key to ensure the reliability of the connected vehicle applications. Recently, machine learning (ML) algorithms are demonstrated to provide dependable predictions to plan, simulate, and evaluate the performance of vehicular networks. In this paper, we propose ML aided New Radio (NR)-V2X QoS predictions scheme to provide Packet Delivery Ratio (PDR) and throughput predictions with the input of Modulation and Coding Schemes (MCS), distance-to-base station, Signal to Interference plus Noise Ratio (SINR), and packet size. Seven different ML algorithms based prediction models are trained and evaluated by using NR-V2X simulation data. We provide performance comparisons between Support Vector Regression (SVR), Deep Neural Network (DNN), Random Forest (RF), Gradient Boosting Machine (GBM), Extreme Gradient Boosting (XGBoost), Categorical Boosting (CatBoost), and Light GBM (LGBM) for predicting throughput and PDR. We demonstrate that CatBoost and RF are the best performing algorithms to predict throughput and PDR of NR-V2X networks, respectively.
更多查看译文
AI 理解论文
溯源树
样例
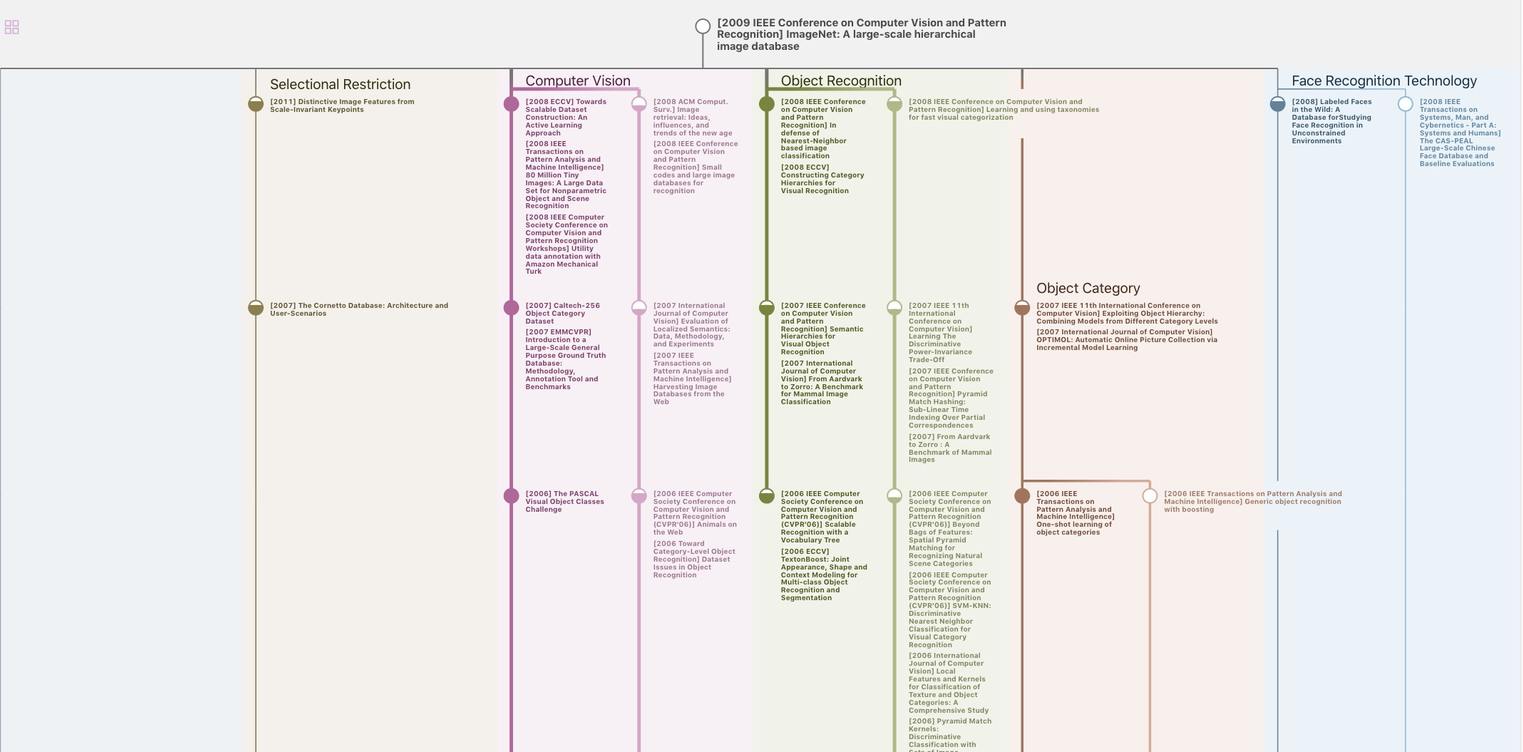
生成溯源树,研究论文发展脉络
Chat Paper
正在生成论文摘要