Scalable Scan-Chain-Based Extraction of Neural Network Models
2023 DESIGN, AUTOMATION & TEST IN EUROPE CONFERENCE & EXHIBITION, DATE(2023)
摘要
Scan chains have greatly improved hardware testability while introducing security breaches for confidential data. Scan-chain attacks have extended their scope from cryptoprocessors to AI edge devices. The recently proposed scan-chain-based neural network (NN) model extraction attack (ICCAD 2021) made it possible to achieve fine-grained extraction and is multiple orders of magnitude more efficient both in queries and accuracy than its coarse-grained mathematical counterparts. However, both query formulation complexity and constraint solver failures increase drastically with network depth/size. We demonstrate a more powerful adversary, who is capable of improving scalability while maintaining accuracy, by relaxing high-fidelity constraints to formulate an approximate-fidelity-based layer-constrained least-squares extraction using random queries. We conduct our extraction attack on neural network inference topologies of different depths and sizes, targeting the MNIST digit recognition task. The results show that our method outperforms the scan-chain attack proposed in ICCAD 2021 by an average increase in the extracted neural network's functional accuracy of approximate to 32% and 2-3 orders of reduction in queries. Furthermore, we demonstrated that our attack is highly effective even in the presence of countermeasures against adversarial samples.
更多查看译文
关键词
Deep neural network,testing,scan-chain,model extraction,scalability
AI 理解论文
溯源树
样例
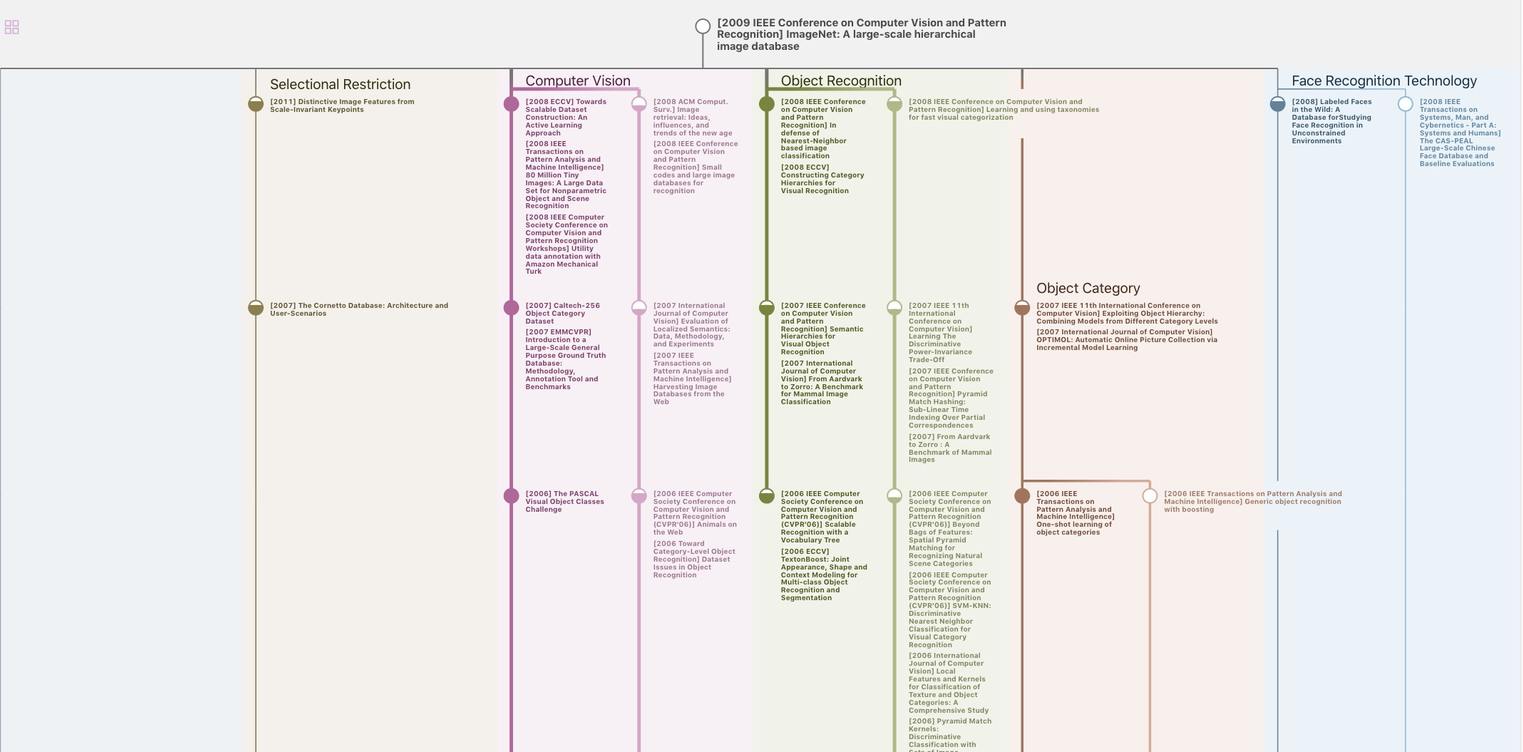
生成溯源树,研究论文发展脉络
Chat Paper
正在生成论文摘要