DeepTH: Chip Placement with Deep Reinforcement Learning Using a Three-Head Policy Network
2023 DESIGN, AUTOMATION & TEST IN EUROPE CONFERENCE & EXHIBITION, DATE(2023)
摘要
Modern very-large-scale integrated (VLSI) circuit placement with huge state space is a critical task for achieving layouts with high performance. Recently, reinforcement learning (RL) algorithms have made a promising breakthrough to dramatically save design time than human effort. However, the previous RL-based works either require a large dataset of chip placements for pre-training or produce illegal final placement solutions. In this paper, DeepTH, a three-head policy gradient placer, is proposed to learn from scratch without the need of pre-training, and generate superior chip floorplans. Graph neural network is initially adopted to extract the features from nodes and nets of chips for estimating the policy and value. To efficiently improve the quality of floorplans, a reconstruction head is employed in the RL network to recover the visual representation of the current placement, by enriching the extracted features of placement embedding. Besides, the reconstruction error is used as a bonus during training to encourage exploration while alleviating the sparse reward problem. Furthermore, the expert knowledge of floorplanning preference is embedded into the decision process to narrow down the potential action space. Experiment results on the ISPD 2005 benchmark have shown that our method achieves 19.02% HPWL improvement than the analytic placer DREAMPlace and 19.89% improvement at least than the state-of-the-art RL algorithms.
更多查看译文
关键词
Chip placement,Reinforcement learning,Intrinsic reward,Three head,Visual reconstruction
AI 理解论文
溯源树
样例
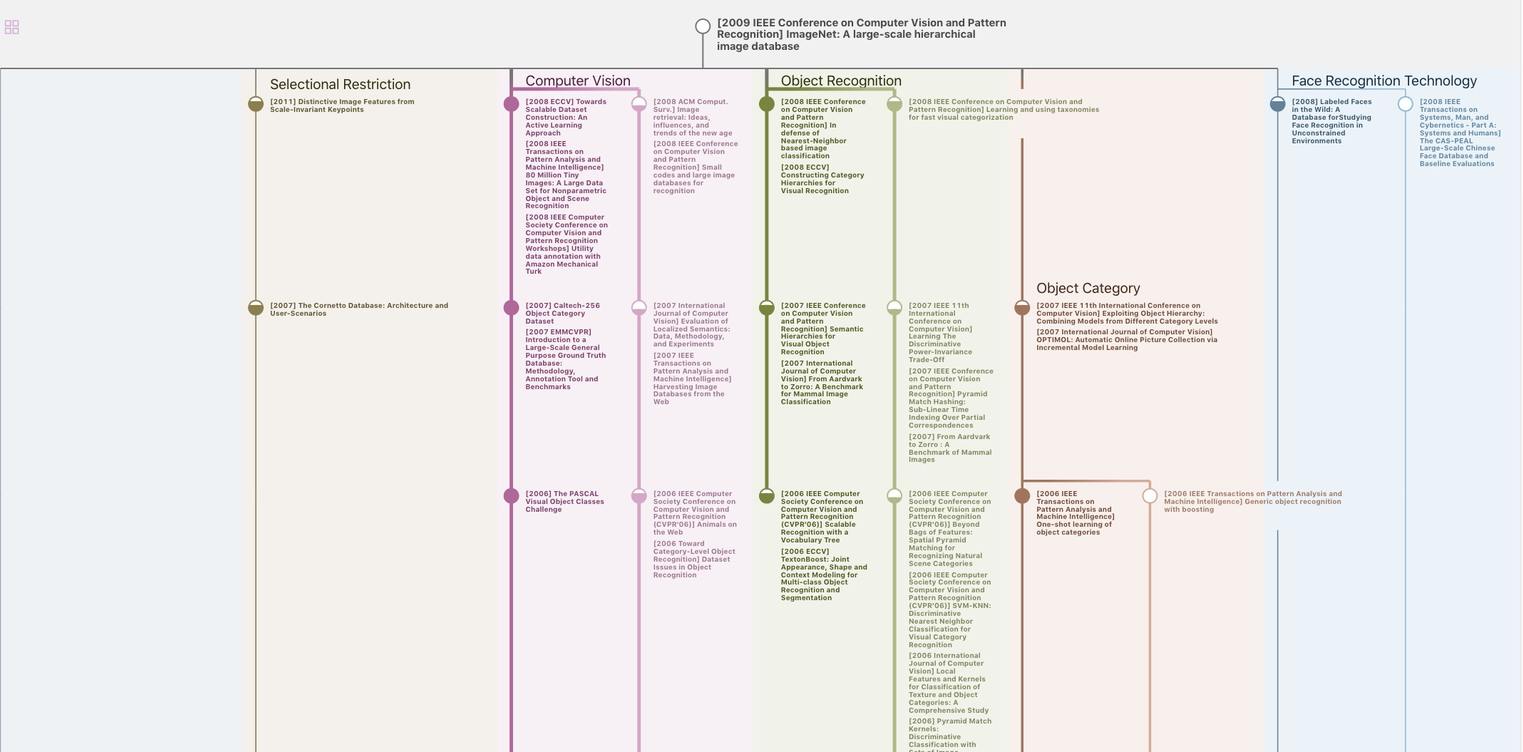
生成溯源树,研究论文发展脉络
Chat Paper
正在生成论文摘要