A Low Overhead Checksum Technique for Error Correction in Memristive Crossbar for Deep Learning Applications
2023 IEEE 41st VLSI Test Symposium (VTS)(2023)
摘要
The matrix-vector multiplication (MVM) is one of the most frequent operations performed in deep learning hardware accelerators. The crossbar array structure with memristive devices as a building block has an inherent capability to perform energy-efficient MVM. However, the memristive devices suffer from various non-idealities as well as limited number of stable levels. Therefore, the reliability and in turn inference accuracy of the deep learning application is negatively impacted. Thus, this paper presents a low overhead checksum-based error correction method for memristive crossbars for MVM computation. The proposed methodology alleviates the problem of storing the checksum value into multiple columns of the crossbar due to the limited number of stable levels of memristive devices. The number of extra columns required for storing the checksum value is reduced, resulting in a significant reduction in the memory overhead by up to 75%. The proposed method scales the checksum value of trained neural networks (NNs) and then performs checksum-aware retraining, and results show negligible impact (∼3%) on the inference accuracy of the NNs on MNIST, Fashion-MNIST, CIFAR-10, and Veg-15 datasets.
更多查看译文
关键词
Matrix-Vector Multiplication (MVM), Checksum, Memristive crossbar
AI 理解论文
溯源树
样例
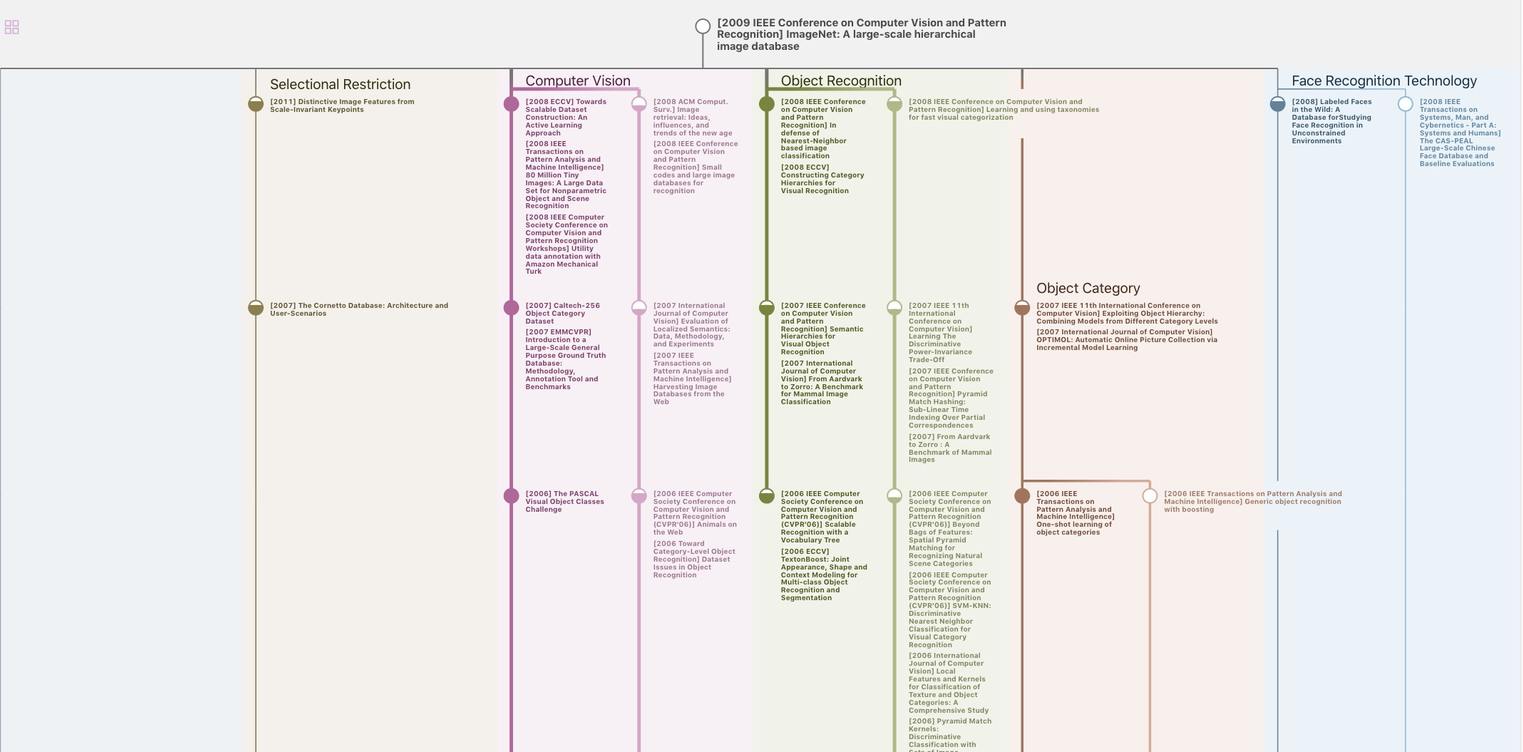
生成溯源树,研究论文发展脉络
Chat Paper
正在生成论文摘要