Physics-informed UNets for discovering hidden elasticity in heterogeneous materials
JOURNAL OF THE MECHANICAL BEHAVIOR OF BIOMEDICAL MATERIALS(2024)
摘要
Soft biological tissues often have complex mechanical properties due to variation in structural components. In this paper, we develop a novel UNet-based neural network model for inversion in elasticity (El-UNet) to infer the spatial distributions of mechanical parameters from strain maps as input images, normal stress boundary conditions, and domain physics information. We show superior performance - both in terms of accuracy and computational cost - by El-UNet compared to fully-connected physics-informed neural networks in estimating unknown parameters and stress distributions for isotropic linear elasticity. We characterize different variations of El-UNet and propose a self-adaptive spatial loss weighting approach. To validate our inversion models, we performed various finite-element simulations of isotropic domains with heterogenous distributions of material parameters to generate synthetic data. El-UNet is faster and more accurate than the fully-connected physicsinformed implementation in resolving the distribution of unknown fields. Among the tested models, the selfadaptive spatially weighted models had the most accurate reconstructions in equal computation times. The learned spatial weighting distribution visibly corresponded to regions that the unweighted models were resolving inaccurately. Our work demonstrates a computationally efficient inversion algorithm for elasticity imaging using convolutional neural networks and presents a potential fast framework for three-dimensional inverse elasticity problems that have proven unachievable through previously proposed methods.
更多查看译文
关键词
Model-based elastography,Elasticity imaging,Deep learning,Tissue biomechanics
AI 理解论文
溯源树
样例
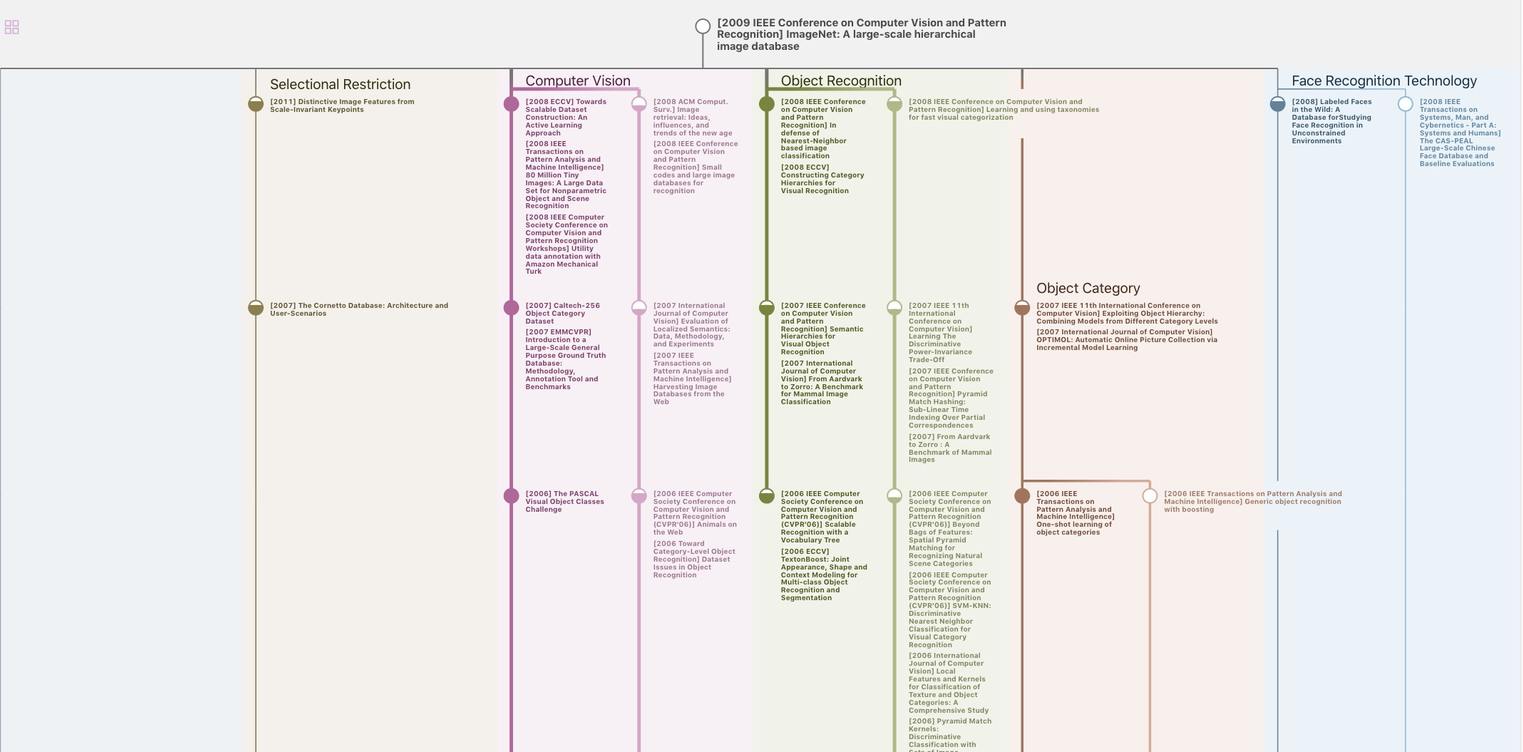
生成溯源树,研究论文发展脉络
Chat Paper
正在生成论文摘要