Tensor decomposition for minimization of E2E SLU model toward on-device processing
arXiv (Cornell University)(2023)
摘要
Spoken Language Understanding (SLU) is a critical speech recognition application and is often deployed on edge devices. Consequently, on-device processing plays a significant role in the practical implementation of SLU. This paper focuses on the end-to-end (E2E) SLU model due to its small latency property, unlike a cascade system, and aims to minimize the computational cost. We reduce the model size by applying tensor decomposition to the Conformer and E-Branchformer architectures used in our E2E SLU models. We propose to apply singular value decomposition to linear layers and the Tucker decomposition to convolution layers, respectively. We also compare COMP/PARFAC decomposition and Tensor-Train decomposition to the Tucker decomposition. Since the E2E model is represented by a single neural network, our tensor decomposition can flexibly control the number of parameters without changing feature dimensions. On the STOP dataset, we achieved 70.9% exact match accuracy under the tight constraint of only 15 million parameters.
更多查看译文
关键词
e2e slu model,decomposition,minimization,on-device
AI 理解论文
溯源树
样例
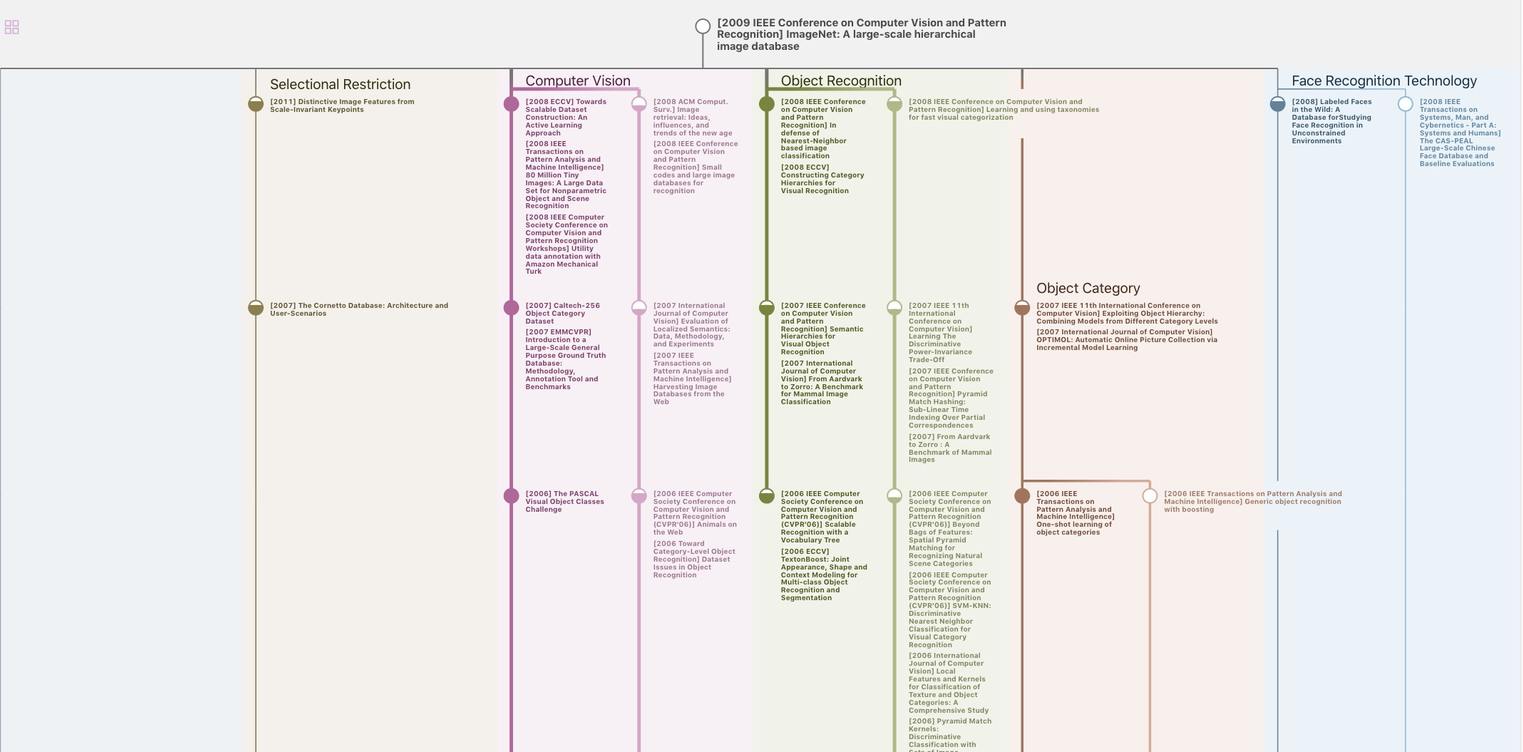
生成溯源树,研究论文发展脉络
Chat Paper
正在生成论文摘要