MetaVL: Transferring In-Context Learning Ability From Language Models to Vision-Language Models
conf_acl(2023)
摘要
Large-scale language models have shown the ability to adapt to a new task via conditioning on a few demonstrations (i.e., in-context learning). However, in the vision-language domain, most large-scale pre-trained vision-language (VL) models do not possess the ability to conduct in-context learning. How can we enable in-context learning for VL models? In this paper, we study an interesting hypothesis: can we transfer the in-context learning ability from the language domain to VL domain? Specifically, we first meta-trains a language model to perform in-context learning on NLP tasks (as in MetaICL); then we transfer this model to perform VL tasks by attaching a visual encoder. Our experiments suggest that indeed in-context learning ability can be transferred cross modalities: our model considerably improves the in-context learning capability on VL tasks and can even compensate for the size of the model significantly. On VQA, OK-VQA, and GQA, our method could outperform the baseline model while having 20 times fewer parameters.
更多查看译文
关键词
learning,in-context,vision-language
AI 理解论文
溯源树
样例
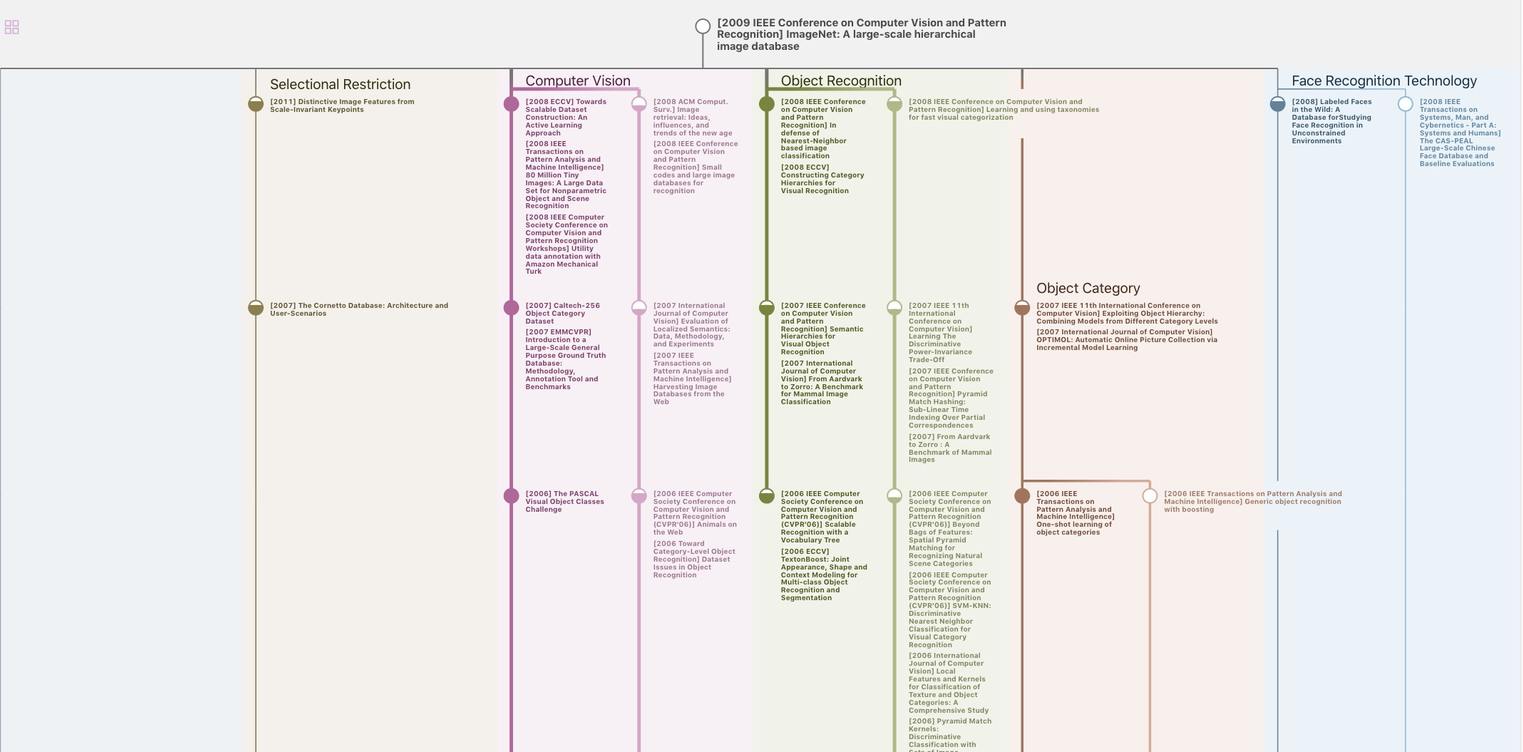
生成溯源树,研究论文发展脉络
Chat Paper
正在生成论文摘要