Transformer-Based Annotation Bias-Aware Medical Image Segmentation
MEDICAL IMAGE COMPUTING AND COMPUTER ASSISTED INTERVENTION, MICCAI 2023, PT IV(2023)
摘要
Manual medical image segmentation is subjective and suffers from annotator-related bias, which can be mimicked or amplified by deep learning methods. Recently, researchers have suggested that such bias is the combination of the annotator preference and stochastic error, which are modeled by convolution blocks located after decoder and pixelwise independent Gaussian distribution, respectively. It is unlikely that convolution blocks can effectively model the varying degrees of preference at the full resolution level. Additionally, the independent pixel-wise Gaussian distribution disregards pixel correlations, leading to a discontinuous boundary. This paper proposes a Transformer-based Annotation Bias-aware (TAB) medical image segmentation model, which tackles the annotator-related bias via modeling annotator preference and stochastic errors. TAB employs the Transformer with learnable queries to extract the different preference-focused features. This enables TAB to produce segmentation with various preferences simultaneously using a single segmentation head. Moreover, TAB takes the multivariant normal distribution assumption that models pixel correlations, and learns the annotation distribution to disentangle the stochastic error. We evaluated our TAB on an OD/OC segmentation benchmark annotated by six annotators. Our results suggest that TAB outperforms existing medical image segmentation models which take into account the annotator-related bias. The code is available at https://github.com/Merrical/TAB.
更多查看译文
关键词
Medical image segmentation,Multiple annotators,Transformer,Multivariate normal distribution
AI 理解论文
溯源树
样例
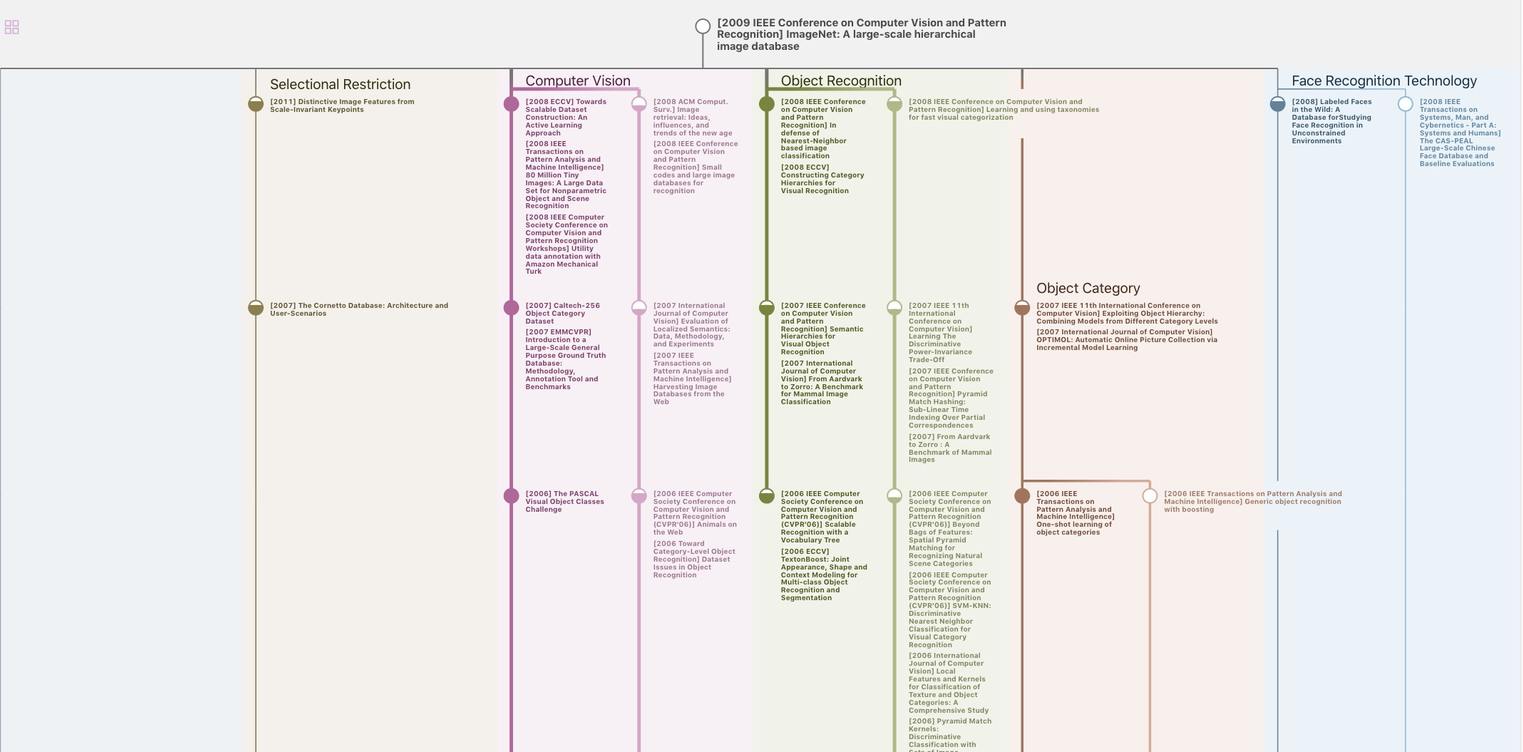
生成溯源树,研究论文发展脉络
Chat Paper
正在生成论文摘要