Leveraging multi-source to resolve inconsistency across pharmacogenomic datasets in drug sensitivity prediction.
medRxiv : the preprint server for health sciences(2023)
摘要
Pharmacogenomics datasets have been generated for various purposes, such as investigating different biomarkers. However, when studying the same cell line with the same drugs, differences in drug responses exist between studies. These variations arise from factors such as inter-tumoral heterogeneity, experimental standardization, and the complexity of cell subtypes. Consequently, drug response prediction suffers from limited generalizability. To address these challenges, we propose a computational model based on Federated Learning (FL) for drug response prediction. By leveraging three pharmacogenomics datasets (CCLE, GDSC2, and gCSI), we evaluate the performance of our model across diverse cell line-based databases. Our results demonstrate superior predictive performance compared to baseline methods and traditional FL approaches through various experimental tests. This study underscores the potential of employing FL to leverage multiple data sources, enabling the development of generalized models that account for inconsistencies among pharmacogenomics datasets. By addressing the limitations of low generalizability, our approach contributes to advancing drug response prediction in precision oncology.
更多查看译文
关键词
pharmacogenomic datasets,drug,multi-source
AI 理解论文
溯源树
样例
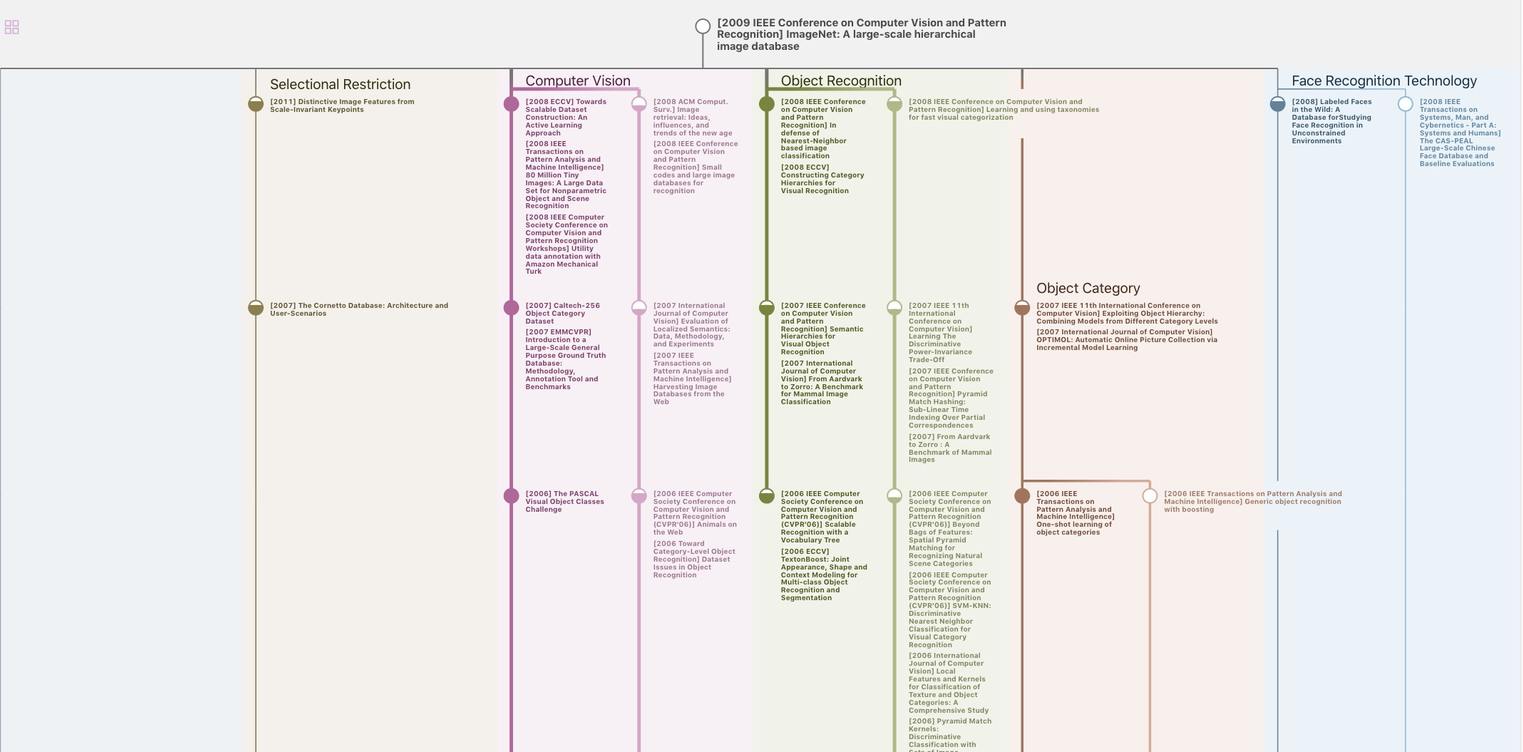
生成溯源树,研究论文发展脉络
Chat Paper
正在生成论文摘要