Stochastically Pruning Large Language Models Using Sparsity Regularization and Compressive Sensing
GLSVLSI '23: Proceedings of the Great Lakes Symposium on VLSI 2023(2023)
摘要
Deep learning models have achieved state-of-the-art performance in many natural language processing tasks. However, their large size and computational complexity make them difficult to deploy in resource-constrained environments. In this paper, we propose a novel approach for reducing the complexity of deep learning models for NLP by combining compressive sensing and Bayesian learning. In our approach, we use compressive sensing with Bayesian learning to identify the most important weights in a deep learning model and represent them in a compressed form. We then prune the non-critical weights and ensure that the model's accuracy is preserved. We evaluate our approach on several NLP tasks, including sentiment analysis and text classification, and compare its performance to that of other compression methods, such as weight pruning and knowledge distillation. Our results show that our approach can significantly reduce the complexity of deep learning models (90% compression) while maintaining high accuracy (<1% drop). In conclusion, our novel approach offers a promising solution for reducing the complexity of deep learning models for NLP and making them more feasible for deployment in resource-constrained environments. By combining compressive sensing and Bayesian learning, we achieve a trade-off between model size and accuracy that is superior to other methods.
更多查看译文
关键词
BERT compression, compressive sensing, pruning, NLP
AI 理解论文
溯源树
样例
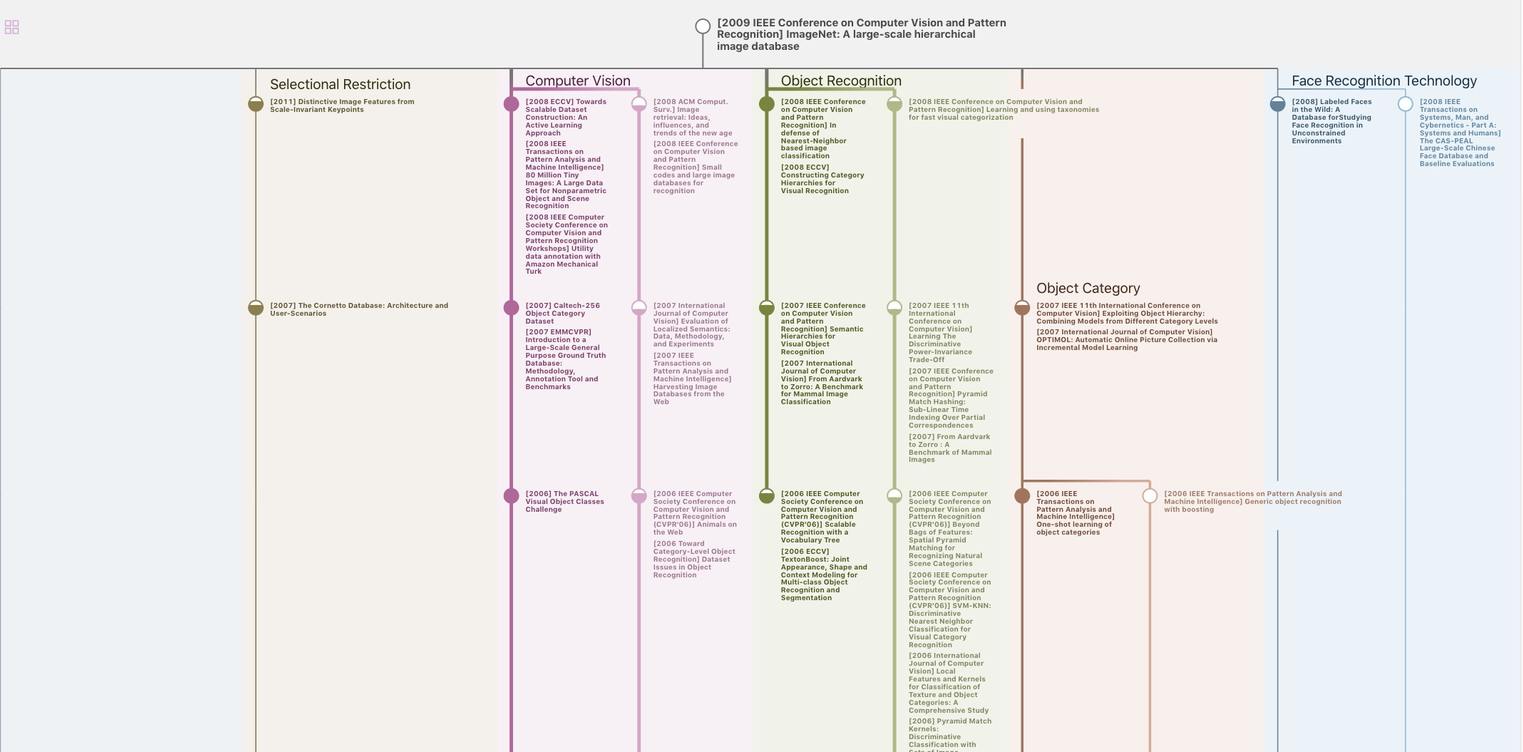
生成溯源树,研究论文发展脉络
Chat Paper
正在生成论文摘要