ATC: Approximate Temporal Coding for Efficient Implementations of Spiking Neural Networks
GLSVLSI '23: Proceedings of the Great Lakes Symposium on VLSI 2023(2023)
摘要
Spiking Neural Networks (SNN) update their neurons' states, the most energy consuming action, only after receiving or firing spikes for energy efficiency. So reducing the number of spikes would lead to more efficient SNN implementations. We propose an approximate temporal coding (ATC) for this purpose. Because the reduction of spikes leads to more synapses being used rarely, we develop a pruning method for further energy improvement. Experimental results validate the efficiency of ATC and the pruning method. On the MNIST dataset, for example, 61% of the spikes are reduced, leading to 60% energy saving without any accuracy loss.
更多查看译文
关键词
approximate computing, spiking neural network, temporal coding, pruning
AI 理解论文
溯源树
样例
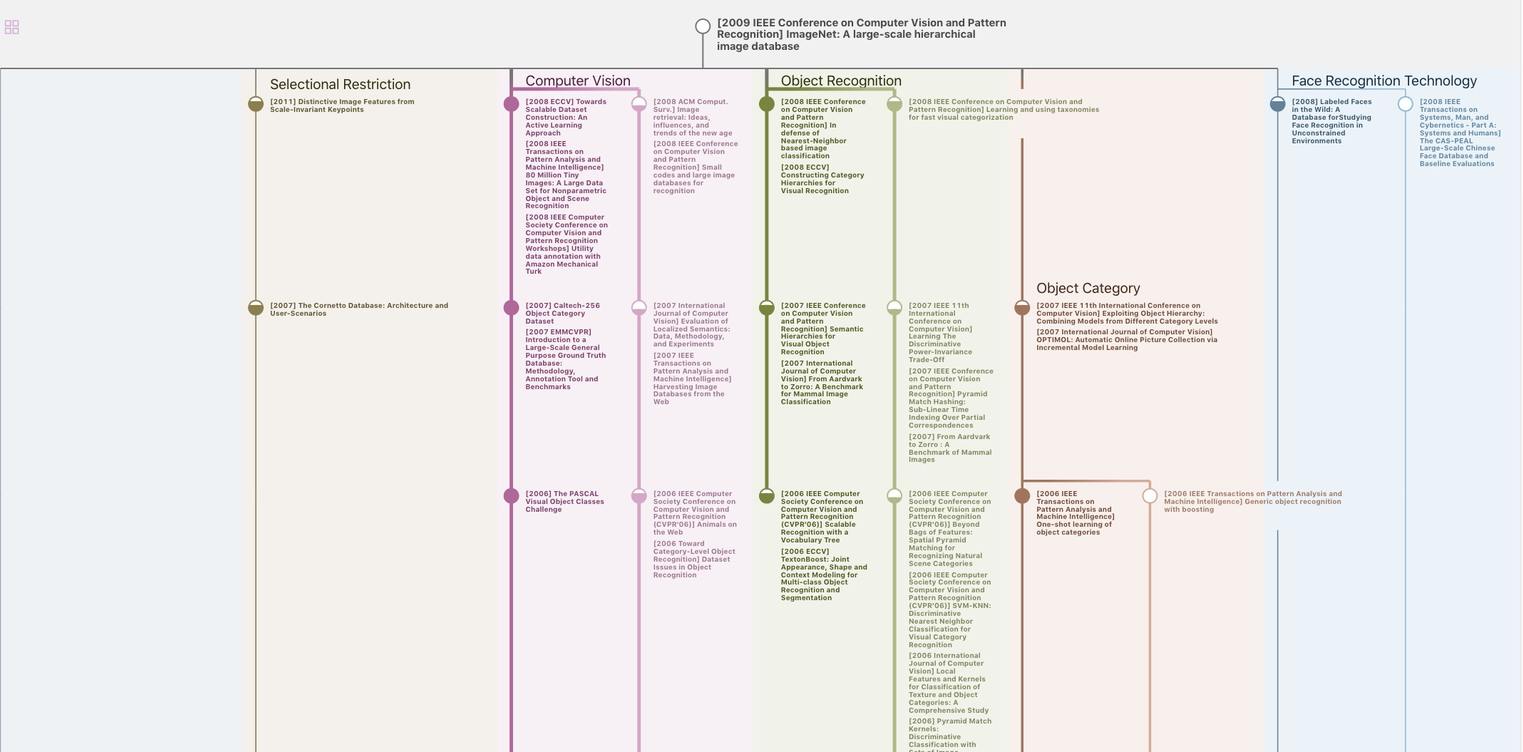
生成溯源树,研究论文发展脉络
Chat Paper
正在生成论文摘要